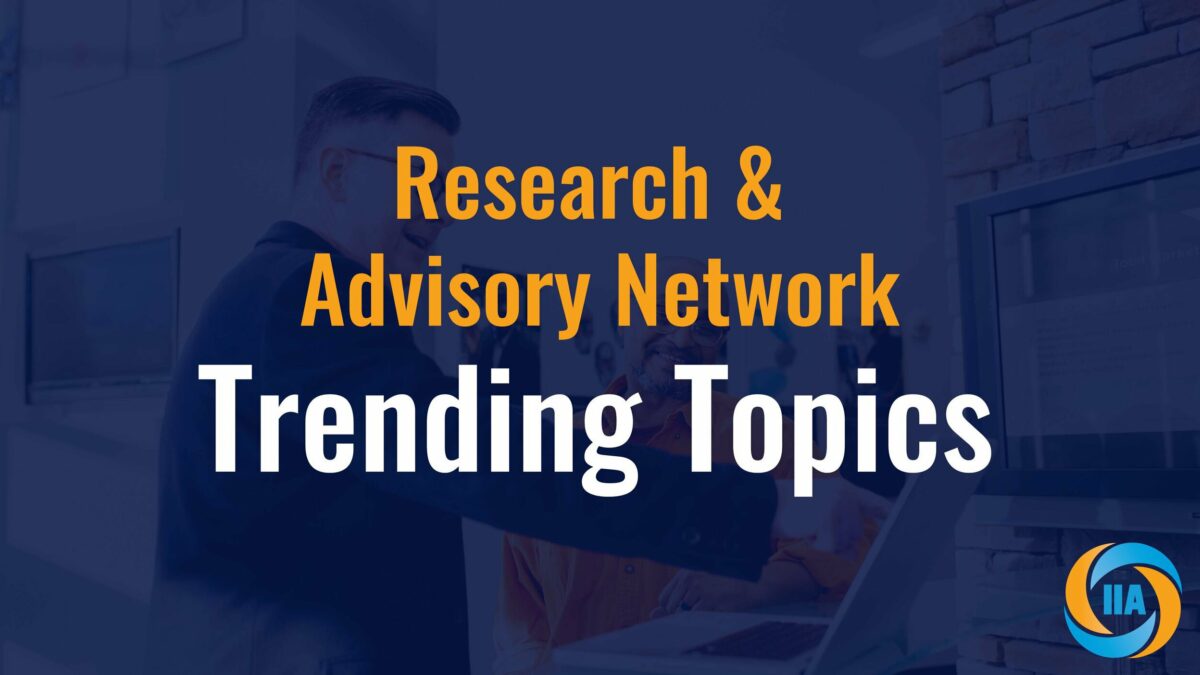
Welcome to the trending topics update!
IIA is in the fortunate position of working with some of the most influential Fortune 1000 analytics teams. On a daily basis we partner with these teams to accelerate the progress on their most pressing projects and initiatives. Collectively, we’ve worked with these analytics professionals on a couple thousand different topics over the years and thought it could be helpful to the broader analytics community to begin sharing some of those topics.
This is our third installment and you can check out my previous one here when we reviewed the following two topics:
Developing a future-state for your data program because your biggest present issue is that your demand-side (i.e. your internal customers) processes are not set up to consume what you provide.
What are some best practices with an enterprise-wide tableau rollout to thousands of users as part of a strategy for bi and self-service?
But for this iteration, without further ado…
Topic 1:
Reconciling different workstyles and behaviors across the data science and data engineering teams.
Summary: We’ve had the good fortune of sharing insights from Experts like Jennifer Prendki who provided a framework to install an agile mindset to data science teams and then also Chris Bergh who shared the seven steps to implement DataOps (with a heavy element involved from engineers). What is emerging as a friction point however, is that these different, though intimately related teams, look at data incredibly differently. That difference in perspective on data, and outputs associated with their interaction with data, can create inefficiencies and even breakdowns in process.
Data engineering teams focus and care about different things than data analytics teams. They tend to be focused on data processes and formats rather than what’s in the data itself. For example, the data engineering team may report that “…data loaded correctly and passed all the tests…”, but the data science team catches that an important variable has gone to zero. How did that anomaly get past them?
A few quick hit ways we’ve seen to manage this data concept incongruity include:
Accept that these are two different groups with two different views of data. Data engineers are not going to explore the data as a data scientist would.
When working together, data scientists need to engage with engineers about their system rather than about the specifics of the data. They need to show them that the system has a hole in it and they may be missing some parameters.
There is a bit of a yin and yang that needs to take place here. Data scientists should get involved in engineers’ world to work with them on what it means to test data well. For example, helping them define unsupervised measures to use as tests for machine learning algorithms.
Then understand that if data engineering does take more ownership of knowing what the data means, data scientists will need to take more ownership of deployability. That is, don’t throw dodgy algorithms over the wall and expect engineers to work magic to get them to scale.
Topic 2:
How can an organization that is used to one-off analytics project work evolve into a product/portfolio way of thinking? In other words, shifting from a project and ad-hoc approach to a data/analytics product mindset that services internal stakeholders in a more wholistic way.
Summary: The word “product” is a bit loaded with different meanings to different constituents. For the purposes of this topic, IIA’s clients tend to be thinking about data as a product in a similar way to a consumer software organization thinking about the interest and use of their offering by their target market. For example, consumers don’t go to Strava or Acorns for a single interaction. These companies have a product/platform approach to provide longstanding, multifaceted value to their consumers.
Taking this mindset to the internal data analytics team can mean presenting yourself in an entirely new way to have your consumers (i.e. your business colleagues) thinking about you differently. That means there is as much of an effort to resource a team that can engage and delight your demand side constituents with flawless UX/UI and marketing, as there is for gathering the necessary supply side resources (e.g. engineers, architects, etc) into one organization, ensuring that you have a unilateral focus on the output of your product for your consumer market. A few keys we’ve seen with this approach include:
Pitch your organization as a software organization with an end-to-end platform that productionalizes products that are critical to achieving company strategies.
CIOs and on down are starting to realize that DevOps software practices are important for analytics organizations too. This is especially true when you consider that your efforts could lead to external products and future revenue streams.
Treating yourself as a software organization means emphasizing that you don’t function as an offshoot of IT, rather there may be IT functions within your data product team organization.
There’s a precedent in many companies to value building some sort of product. You’ll have more success if you position your organization this way rather than as yet another data warehouse team or another ETL process team, which are traditionally IT.
Your goal is to evolve the way the company thinks, and if you approach your mission through the lens of software development, you may not find it as hard as you think to get your business colleagues and leadership on board.
These couple may not track with what you're working on, but hopefully are interesting to see what others are up to in this small community of analytics professionals that we all work in.
Till next month!
— Doug