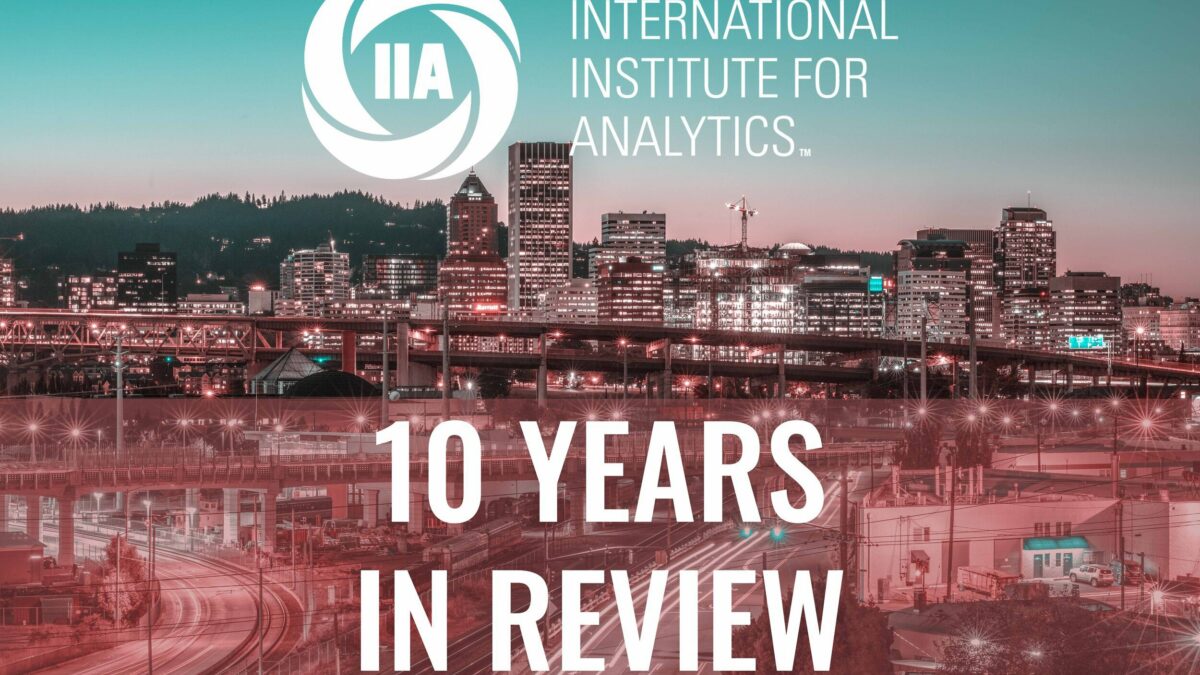
Co-written by Jack Phillips.
We co-founded the International Institute for Analytics in 2010. Since it’s now 2020, our sophisticated math skills tell us that IIA has been around for about a decade—although our first full year of operation was in 2011. We thought it might be interesting to reflect on the state of the field that IIA addresses and how it has changed over time.
What to Call It?
First, a few reflections on the term “analytics.” By 2010, Tom had published a Harvard Business Review article and co-authored a book, both entitled “Competing on Analytics.” It seemed obvious that our new research and advisory firm should have “analytics” in its name. However, that proved to be a somewhat challenging issue over time. There is certainly still some popularity around “analytics,” but other terms like “big data,” “artificial intelligence,” and “data science” have chipped away at it—even though all of these terms involve heavy use of data and statistical analysis. The use of the term “analytics,” if Google Trends is a good indicator, is declining somewhat now, but it’s still substantially more popular than any of the alternatives. As a generic name for the use of data and statistics in business, “analytics” has proven to be both popular and durable—the best if not the only term for the field.
Vendors v. Users
When we started IIA, we envisioned that the sponsorship of the research and advisory services would be from a mix of vendors and users of analytics. That turned out to be somewhat true, but the role of vendors has ended over time. This is in part because representatives from user companies don’t like to be sold to all the time, but perhaps more so because of the incredible rise over the decade in the use of open source statistical tools and programming languages. Open source programs have some great capabilities, but they don’t sponsor research or conferences. We also know there is no perfect option for analytics as each organization has so many other factors impacting their data.
Bob Muenchen, the University of Tennessee statistical computing guru, compiles the best data about open sources in his latest rankings of tool popularity, the top ten tools include only three proprietary vendors, and they’re close to the bottom. The top five are Python, SQL, Java, Amazon Machine Learning (another important trend is the rise of cloud vendors, of course), and R. SAS, the traditional proprietary leader in this space and an early sponsor of both IIA and my analytics research, came in tenth. Microsoft Excel isn’t on this list, but we suspect that it’s still the most popular tool for simple analytics.
Big Change in Tech
The changes in terminology and vendors mirror changes in analytical technology. Tech, of course, changes faster than the human approaches to data and analysis. Over the past decade, we’ve seen a lot of change, including:
The rise of open source statistical analysis tools like R
The emergence of new open source tools for storing and accessing data, like Hadoop
The shift to programming languages like Python as opposed to statistical packages
The rapid growth in visual analytics tools such as Tableau and Qlik
The birth and rapid ascendance of cloud vendors of storage and algorithmic tools including AWS, Microsoft Azure, and Google Cloud
The rebirth of AI and relatively new methods for it like deep learning
The increased automation of analytics and data science, such as automated machine learning.
These are fantastic developments, but they make it hard to be either a vendor or a professional user of analytics. Vendors have to be constantly updating their offerings to emphasize the latest new thing, and of course it is difficult to make money with so many open-source alternatives. Users of analytical tools have to continuously master new technologies; this has not been a good decade for those who prefer to rest on the skills they learned in school. And none of the previous capabilities needed by analytical professionals have gone away; they still need to possess business acumen, to tell good stories with data, and to manage organizational change. Perhaps needless to say, it’s become increasingly difficult to be a “unicorn” who possesses all these technical and organizational skills; diverse teams with complementary capabilities are almost always required.
A Needed Shift Toward Deployment
At the beginning of the decade we seemed to assume that the job of the analyst or data scientist was to build a model, and that it would inevitably be implemented. But that turned out not to be the case; many models were not deployed for any number of reasons. Some companies even admit to deployment percentages of zero. As model creation gets more automated and presumably easier, that could free up analytical professionals to focus more on deployment issues. And as we argued in the 2020 Predictions and Priorities webcast, technologies like containerization and “MLOps” frameworks are also making deployment somewhat easier. We expect that the job focus of most analysts and data scientists will continue to shift toward deployment.
From Decentralized to Centralized to Coordinated
At the beginning of the decade, it was a common organizational strategy to aggregate a decentralized analytics group into a fully centralized organization. Centralizing analytics had a number of benefits; critical mass, the ability to form diverse teams for projects, and being able to rotate analysts among different types of projects. Of course, any centralized function can be bureaucratic. But in many cases, we believe it was the success of centralized groups that led to their own demise. Business leaders realized what analytical talent could do for their businesses, and decided that they wanted it reporting directly to them. In addition, the panoply of different analytical technologies and use cases meant that analytics could be applied virtually anywhere within a business. It’s difficult for a fully central group to service all those possibilities.
Organizational structures for analytics will continue to evolve, but they are arguably drifting toward pervasiveness. “Digital native” firms like Google, Amazon, and Facebook don’t typically have centralized or even coordinated analytics groups; instead, analytics and AI are everywhere. Virtually all decisions, strategies, and processes are supported by data and analytics. The lack of a central or even identifiable group of analysts and data scientists isn’t evidence that analytics are important, but just the opposite: the entire business is based on analytics.
The Future..
The success of these analytically pervasive businesses—and the continued rapid rise in data in business and society—mean that analytics, data science, and AI will be with us for the foreseeable future. We simply can’t do without tools, methods, and talents that will help us make sense of all that data. As a result, no one could plausibly call analytics a fad or a movement that only took place in the 2010s. We’ll be writing it, being apart of it and sharing with you over the next decade, the fantastic and highly positive developments that will characterize the field by 2030.