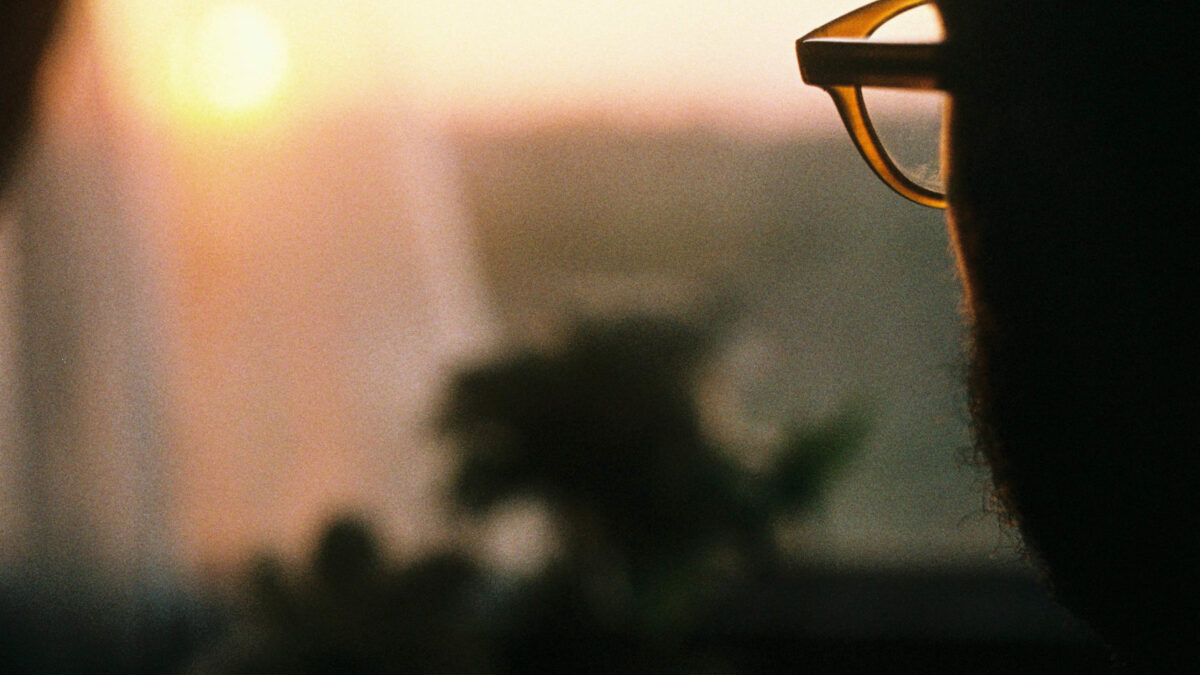
Creating an Evolutionary Analytic Platform for Risk Estimation
This article describes the potential for AI to augment risk estimation for both individual investors and financial market assets. AI processes vast amounts of a variety of data to identify patterns underpinning processes and metrics. Evolving data resources including digital touch points provide AI with attributes that can enhance risk estimation to ultimately augment elements of modern portfolio theory.
There has been heightened emphasis on the utilisation of AI in various applications throughout industries. This algorithmic learning and replication technique that processes data to learn how activities work, has reached intense depths given the creation of vast data resources in the evolving digital era. The results of AI initiatives have been both mixed and alarming as applications including voice recognition, identifying fraud, understanding consumer propensities and replicating tasks performed by humans have intrigued business leaders and heightened concerns in the workforce as to the potential of labour displacement.
One particular AI-based initiative is in the financial sector that falls in the realm of FinTech. This has been the creation of robo-advisors or the use of algorithms to enhance the activities of financial advisers interacting with investors regarding asset allocations among various financial market based assets (e.g. equities, fixed income, commodities, etc) for wealth and retirement management. These platforms can augment the tool set of “human advisors” and also introduce a more total technology interface (e.g. evolving robo-advisors) that investors can access for investment advice.
The AI analytics “under the hood” of this technically intensive initiative processes vast data resources that describe investor attributes and financial market asset classes. Algorithms can be used to identify relationships that can exist between investor stage in life (similar to a product life cycle approach), wealth and risk appetite or attributes of foreign exchange based products, stocks, bonds etc. domestically or globally.
Algorithmic learning and replication technique that processes data to learn how activities work, has reached intense depths given the creation of vast data resources in the evolving digital era.
THE BASIS OF RISK
One of the main factors that ties the entities of market fluctuations and investor propensities is the term “risk”. This term applies to the risk that investors can tolerate regarding fluctuations in portfolio value and also applies to the volatility of potential returns corresponding to financial assets. It is this factor that may provide a great need for evolving applications of data science and AI while maintaining the need for the skilled financial “human factor” as well in financial advising. On the investor side of the equation, risk generally entails the potential of gaining or losing value in the financial assets comprising their portfolios. A generally accepted principal for investor risk is that achieving higher portfolio returns involves increasing the amount of risk (or adverse fluctuations in asset value) or, according to Modern Portfolio Theory, investors will choose the scenario or portfolio of lower risk given equal expected rates of return. Some basic ideologies involve the idea that younger investors can take on more risk relative to older ones because of the increased time to recoup losses because of a longer investor horizon. While simple personality traits entail some high-risk takers or conversely risk averse personalities.

AI AND THE EVOLUTION OF INVESTOR RISK ESTIMATION
Previous research introduced an approach to augment Modern Portfolio Theory which involves the concept of a wealth allocation framework that more broadly defines individual investor risk. This augmented notion of investor risk includes more descriptive variables involving behavioural finance to better estimate personal risk. Once this is established, the appropriate asset allocation can be achieved. These attributes can be harnessed through evolving data resources that provide AI with the inputs to better estimate investor risk. These data resources can include behavioural attributes that can be extracted from investor activities corresponding to financial market characteristics (e.g. how investors react to volatility in various markets), simple consumption patterns of individuals along with general descriptors of investors. The risk profile of individual investors presents an attractive task of data scientists skilled with AI modeling.
AI modeling in this Fintech application involves the analysis of more descriptive and behavioural attributes of investors which can be processed to estimate personal risk. Many new attributes are available due to the digitation of organisational and consumer activities. A broader spectrum of attributes www.europeanbusinessreview.com 65 that describe consumer risk tolerance can provide wealth/financial advisors a more accurate profile of individual risk.
Digital transformation across industry sectors has generated micro-detailed data that record and describe activities. Consumption patterns of individuals can be tracked in much greater detail that provide insights into cash-flow status and requirements of daily living that can play a critical factor in determining risk estimation. Individual risk can also be estimated at a more personalised level given the emergence of digital touch points or records of consumers’ direct activities with various technologies. For the FinTech case, digital touch points refer to the reaction of investors to market swings that effect the value of portfolios. In other words, investor behaviour in liquidating or adding to asset classes in response to market volatility of assets can provide a more objective portrayal of investor risk.
This type of analytic approach applied to individual risk estimation provides significant value as it entail the analysis of more objective data resources that learn from actual individual behaviour rather than wealth advisors relying on risk assessments based off of self-reported attributes from investors (e.g. investors may perceive themselves as risk averse or aggressive in risk taking, however behavioural patterns may prove otherwise). The AI approach ultimately seeks to enhance the estimation of the personal utility function in Modern Portfolio Theory.
AI AND MARKET RISK
The other side of risk deals with market risk. A prime component of measuring risk of financial assets is a term referred to as volatility, which entails the potential magnitude of change of a financial assets value. For instance, what is the chance a given stock price of a company in the tech sector will fluctuate up or down more than four standard deviations from a base measurement over the coming month? The higher the probable change in value, the more risky the asset. To add to the complexity of this measure, one must consider the interrelated forces between financial market assets. Take for example the notion of higher interest rates and their effects on the price behaviour of stocks, or the differential of interest rates between trading countries’ and the effects on their corresponding currencies. Now add yet another component that adds yet more complexity, which entails economic conditions or geopolitical factors that can add multiple entities of data variables and data points that can effect volatility of a host of financial assets (Bloomberg). This is a scenario in which AI is naturally applicable. The equation for advisors is to maintain an acceptable level of risk between investor profiles with that of financial asset classes to achieve desired returns.
AI and data science are the “the new kids on the block” regarding the arsenal of quantitative and statistically based methods that provide decision support in the financial market/investment sector.
NOT JUST ANALYTICS BUT TIMING OF MODELS
AI-based platforms are created by applying algorithms to data that underpin processes and activities to identify patterns that are used to create models to perform those tasks they learned to replicate. However, drastic changes in the characteristic of the data and the patterns that exist require a re-optimisation of those models. As characteristics of financial markets change (e.g. higher interest scenarios, geo-political turmoil, etc), risk profiles change, and not just financial market risk but the risk profiles of investors may be altered as well.
This process refers to the evolution of financial modeling, where more traditional economic, quantitative and statistically based techniques can be augmented by AI regarding such areas as retirement programs, account aggregation and more shorter-term interactive, investment advice. AI and data science are the “the new kids on the block” regarding the arsenal of quantitative and statistically based methods that provide decision support in the financial market/ investment sector.
Simply put, AI in the Fintech industry introduces significant value as it supplies enhanced decision support for financial advisors and can also provide an automated platform to facilitate the needs for their customers in the digital era. The optimal platforms remain to be a combination of experienced and skilled human experts, existing decision support methods now augmented with AI, and the addition of data scientists skilled in this area who can monitor the effectiveness of these evolving analytic techniques in this dynamic and ever changing space.
Individual risk can be estimated at a more personalised level given the emergence of digital touch points or records of consumers’ direct activities with various technologies.
The evolving risk estimation platform should enhance the current state of equating portfolio risk and asset allocation with investor risk by broadening the knowledge base of wealth management firms regarding investor advice, mitigating risk relative to asset returns, options of alternative asset based portfolios and new risk models. Some firms have embraced the AI-based evolving risk platform. Vanguards Personal Advisor services involves a hybrid process of machine and human based investment services and Morgan Stanley offers its Next Best Action platform to enhance its investor services. AI has introduced concerns throughout industries regarding the potential of labour displacement due to automation, however reality may be that more knowledge-based workers and data and analytic skilled workers may prove to provide a balance in the equation.
Originally published in European Management Review