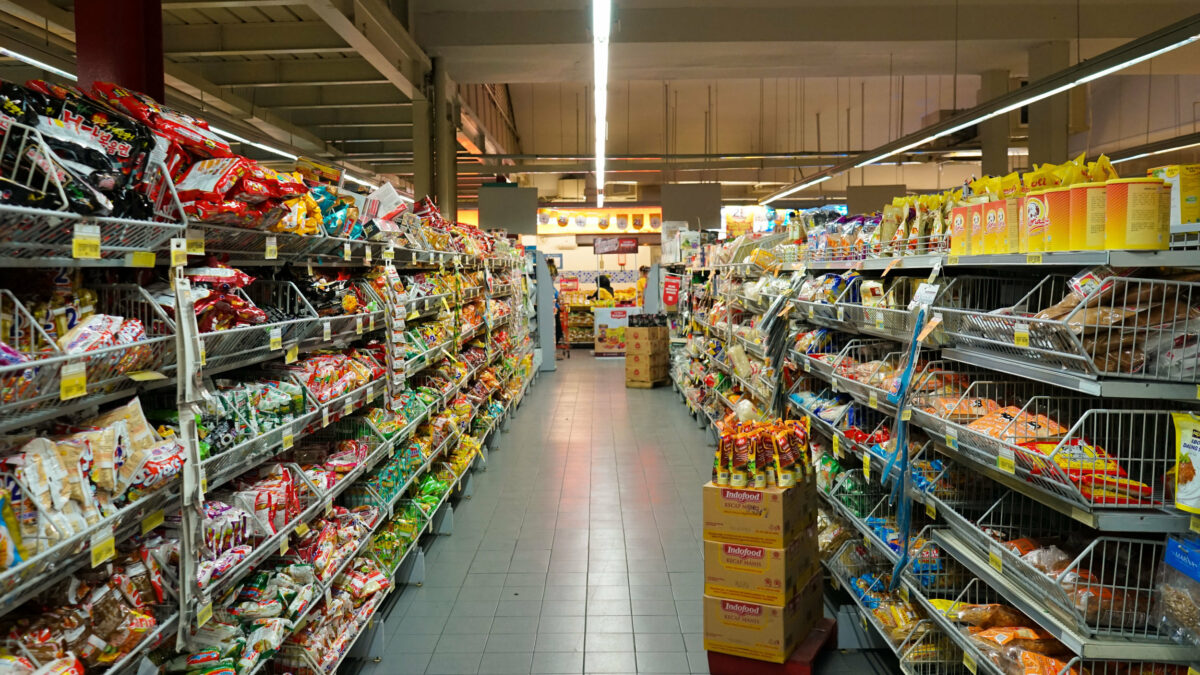
There is widespread agreement that the Internet of Things will be a transformative factor in the business use of information. The prospect of billions of connected devices promises to transform home activities, transportation, industrial operations, and many other aspects of our lives.
The bad news about the IoT is that we have a lot of work to do before we are ready for it. We’ve got to up our games considerably with regard to data management and analytics if we’re going to capture, store, access and analyze all the IoT data that will be flowing around the Internet. The good news (in addition to its potential) is that most organizations have a few years to get better at these capabilities before the real onslaught hits. The sensor devices, IoT data standards, and data management platforms are still in their relatively early stages, and no customer, business partner, or CEO could reasonably expect that you could tame all that IoT data today.
But they will soon. So it’s time to think now about the data management and analytics capabilities you will need to have, say, over the next five years as the IoT matures and blooms. I’ll describe eight of them, but of course there are some other capabilities that underlie them (data security, for example). These are about running, but you will have already needed to walk first.
Data quality tools on steroids — The IoT is going to generate a massive amount of data, and a good bit of it is going to be of problematic levels of quality. Sensors will send bad data, devices will go offline and create missing data, and integration platforms will fail to integrate. So companies need to improve their data quality capabilities massively and employ automated tools to a large degree. This includes identifying data quality problems, determining their seriousness, and fixing them both after data have been collected and at the source.
Data curation on a grand scale — Similarly, companies will need to become much better and faster curators of multiple data sources. If you’re a car manufacturer, for example, your cars already have a couple of hundred sensors in place, and you’re probably planning a lot more. Data curation allows companies to keep track of their data sources, their formats, and their interrelationships. And the scale of the IoT is going to mean that companies will have to make widespread usage of tools like machine learning, which are already being applied to data curation in some companies and vendors.
Qualify your alerts — Alerts are one of the key ways to analyze IoT data, in that organizations will need to know what readings are in and out of normal bounds. But the vast amount of IoT data is going to make alert fatigue a common occurrence unless you have done a good job of qualifying alerts to ensure that they are real and important. You’ll also need to qualify the many security alerts that your IoT system will probably generate. All of this is going to require some high-quality diagnostic models, and I’m guessing that you don’t have them today.
Swim in a data lake — You’re not going to be able to undertake an extended ETL (extract, transform, and load) process to store your IoT data in a traditional data warehouse. Some data may eventually go there, but you need to store and refine it first. So you had better establish a data lake that lets you store the data in whatever format it comes in until you need to analyze it. By the time the IoT data arrives in force, you should be well-practiced in moving data into and out of your data lake.
Predictive analytics — Most organizations thus far have only employed descriptive analytics with IoT data—bar charts, alerts, means and medians. These are useful but not nearly as useful as predictive analytics. We’ll want to know whether a machine is about to break down, whether your car is likely to arrive on time, and whether your good health will persist. That takes a solid competency at predictive analytics.
Automated recommendations and actions — IoT data will flow into your organization at a fast and furious rate, and you’re not going to have enough humans to examine and decide upon it. That means you should be well-versed in building and using automated decision systems by the time the IoT is mature. This capability could take a variety of forms—simple rules, event-driven systems, or sophisticated cognitive capabilities (see the next two items). By the time the IoT is ready, you should be ready to employ the right automation technology for any situation.
Machine learning to create analytical models —Automating IoT processes will require a large number of analytical models, and you won’t have the time or people to create them using traditional hypothesis-based methods. Each type of device and data is going to require its own set of models, and the analysis situations will change quickly. So machine learning is the ticket to developing models rapidly and with much greater analyst productivity. Start now to develop a facility with it, because machine learning is relevant to a wide variety of situations. Machine learning models can also be helpful in identifying unauthorized intruders into your systems, which is critical for IoT security.
Deep learning models for image and sound data — Deep learning, which is based on neural network methods, is the best way to analyze large amounts of image and sound data. Want to know if the drone images you’re receiving detect an unauthorized intruder? Are your sonic sensors detecting squeaks and squeals from your car engine that indicate a lack of lubrication? Deep learning models are the way to make sense of this data. They can also be used to identify patterns in cybersecurity attacks.
No doubt there will be other capabilities that IoT-centric organizations will need to develop, but this is a good start. And many of the ones I have mentioned have relevance to other types of data and analysis contexts. An IoT-capable data and analytics environment is basically one that is state of the art given the technologies and analytical methods that are available today. So it’s time to get busy and make sure you have an implementation trajectory that will ensure you are ready when the IoT data starts flowing in a big way.