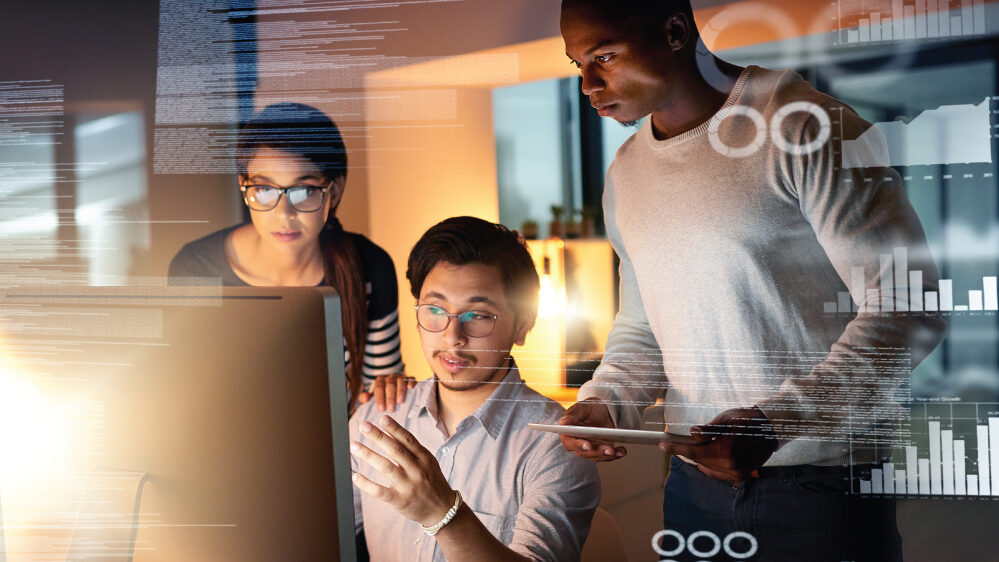
Read below for a roundup of interesting sites, resources, and articles from around the web, curated and contextualized by unbiased analytics experts at IIA. Highlights include articles on what's holding back AI from being fully autonomous, the different types of data engineering teams , and a case study on data products at Regions Bank. Follow us on Twitter and LinkedIn to receive daily updates on IIA content and curated content as it becomes available.
Featured Articles on Analytics Strategy
Breaking 6 Analytics Habits to Unlock Value (Towards Data Science)
This article discusses six habits that analytics professionals should break to unlock value. These habits include relying too heavily on existing data sources, neglecting to explore different models, not considering data usage rights, ignoring data security, and failing to communicate results. The article also provides strategies for breaking these habits, such as investing in data quality, experimenting with different models, and taking a proactive approach to data security.
Different Types of "Data Engineering" Teams (SeattleDataGuy)
This article breaks down the various types of teams that data engineers can be part of and how the data engineering role differs from team to team. The author covers everything from data infra teams to data platform teams.
Manifesto for the Data-Informed (Medium)
Building a data-informed culture is hard, and it’s “not just creating dashboards, running a/b tests, and shipping ml models.” The author proposes that being a data-informed organization really means internalizing the following set of values:
1. Conviction around a purpose
2. Setting verifiable goals
3. Company-wide familiarity with metrics
4. Active testing of beliefs
5. Accepting probabilities rather than thinking in absolutes
Featured Articles on Analytics Use Cases
AI in Medicine is Overhyped (Scientific American)
AI in medicine has been touted as a revolutionary tool to increase accuracy and efficiency, but many medical professionals disagree. Find out why AI may not be the cure-all it appears to be in the Scientific American article below.
What Machines Can't Do (Yet) in Real Work Settings (MIT Sloan Review)
This article from the MIT Sloan Review looks at the limitations of machine learning and automation in real work settings. While machines have become increasingly capable, they still lack the human ability to adapt, learn, and unlearn. Machines are also limited by their lack of context sensitivity and their inability to understand complex social dynamics. As a result, machines are still not capable of performing all tasks that require human judgment and social skills. This article provides an overview of the current limitations of machine learning and automation in real work settings.
Developing Successful Data Products at Regions Bank (MIT Sloan Review)
This article examines Regions Bank's successful process for creating data products using a three-pronged approach of data strategy, data governance, and data monetization.
Featured Articles on AI
Why So Many Organizations Are Getting AI and Machine Learning Wrong (Towards Data Science)
This article argues that many organizations are adopting AI too fast and expecting unrealistic results. Instead of optimizing day-to-day processes, many organizations are just using AI to "automate bad decisions faster". The author posits that in order to gain effectiveness from AI, we need to dispel some of the myths about what its capabilities are.
Is ML Explainability the Wrong Goal? (Towards Data Science)
This article provides an argument against the importance of explainability in machine learning models. The author suggests that accuracy and performance should be prioritized over explainability in ML models as some models may never truly be explainable. Additionally, the article covers the difference between explainability and interpretability and poses the question of when we should consider a use-case high-stakes.