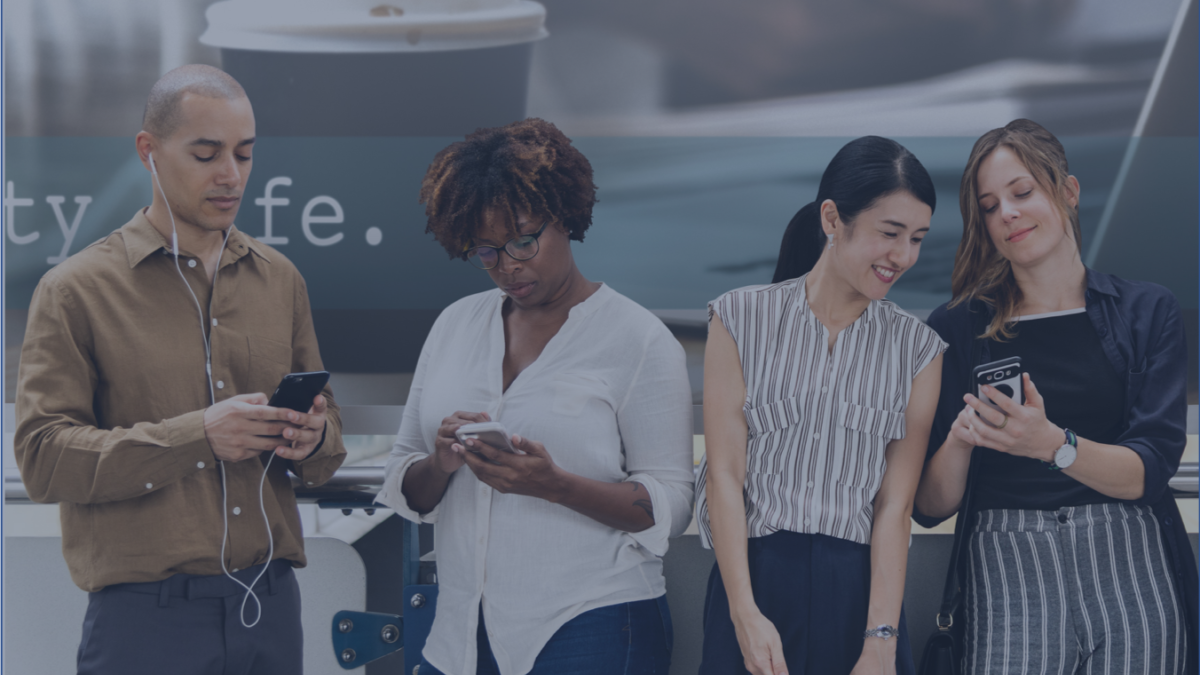
It seems to be inevitable. Any popular technology or approach to business change apparently has to involve a large amount of breathlessly positive media-driven hype, and then must be followed by potshots and disparagement. The positive press usually lasts for a couple of years or so, and then authors, journalists, and speakers who seek attention realize that they can’t get much of it by jumping on the optimistic bandwagon. They then begin to criticize the idea. Both the positive and negative hype are typically equally unrealistic.
Since there has been enormous positive hype about AI—it will transform organizations virtually overnight, eliminate human drudgery, cure cancer, etc.—it’s not surprising that the negative accounts have started to emerge. In my inbox today, for example, I noticed stories like these:
“A new white paper is about to release that shows 85% of Artificial Intelligence projects FAIL.” (I have provided the link, but I would advise you not to click on it for reasons I describe below)
“What if AI in health care is the next asbestos?” (complete with photo of a piece of asbestos)
Why AI is inherently biased. (But wait, there’s more: “And it’s not the first example of AI—a technology developed by an industry that is overwhelmingly populated by white men—producing results that reflect a racial bias in particular.”)—referring to this report.
Like the positive hype, these scary accounts, which usually emerge from PR firms, usually have at least a grain of truth to them. Yes, it’s true that some AI projects fail; it’s still an emerging technology, and many companies have undertaken pilots or proofs of concept to learn if it will successfully address particular use cases. It doesn’t work for all of them. But no one—repeat, no one in this world—has done a study of all AI projects, or even a systematic sample of them, to see what percentage of them fail.
The particular PR message and white paper saying that “85% of Artificial Intelligence projects FAIL” actually has no data at all on what percentage of projects fail. It simply refers to a 2018 Gartner report predicting—not documenting—that “through 2022, 85 percent of AI projects will deliver erroneous outcomes due to bias in data, algorithms or the teams responsible for managing them.” As with many Gartner predictions, no data sources are cited. Nor is it clear that all, or too many, of the “outcomes” delivered by AI will be “erroneous.” I guess Gartner, known for its “hype cycle,” now feels obliged to help nudge technologies along the path to the “trough of disillusionment.”
What happens around such predictions, of course, is that vendors or consultants say, “Use our solution and avoid the problem.” In the case of the “85% FAIL” white paper, the authors are advocating their particular brand of localization for natural language processing. Vendor hype is not behind the “AI as asbestos” metaphor; that comes from Jonathan Zittrain, a smart Harvard Law School professor whose work I normally respect. I don’t know what makes him think that AI will cause cancer or something similarly dire, but it’s not because he has a product to hawk.
The key message here, however, is to deflate both positive and negative hype. If you have an analytics background you know, as Bill Franks and I have both argued on this site, that AI is mostly just an extension of what we’ve been doing for a while in predictive analytics. It’s a powerful but familiar tool that isn’t going away and won’t fail 85% of the time. You should be adding AI capabilities to your organization’s analytics portfolio, but you shouldn’t be promising miracles for them.
Similarly, you may have to puncture some of the negative hype balloons as well. It’s important to point out that while AI is great at churning out models, producing business change from those models is as difficult as it has ever been. And yes, there can be algorithmic bias in AI models, but the decisions they make are usually much less biased than those made by humans, and there are often ways to detect any bias in algorithms. In general, try to communicate that organizations that don’t try to change their processes with AI and analytics will be at a substantial competitive disadvantage to those that embrace these tools aggressively.
Analytics and AI professionals didn’t generally create the positive hype, so they shouldn’t be blamed for it. And they certainly aren’t behind the negative hype either. It may be unfair to ask them to play a role in disseminating the truth about their chosen profession, but I’m not sure who else will play that role.