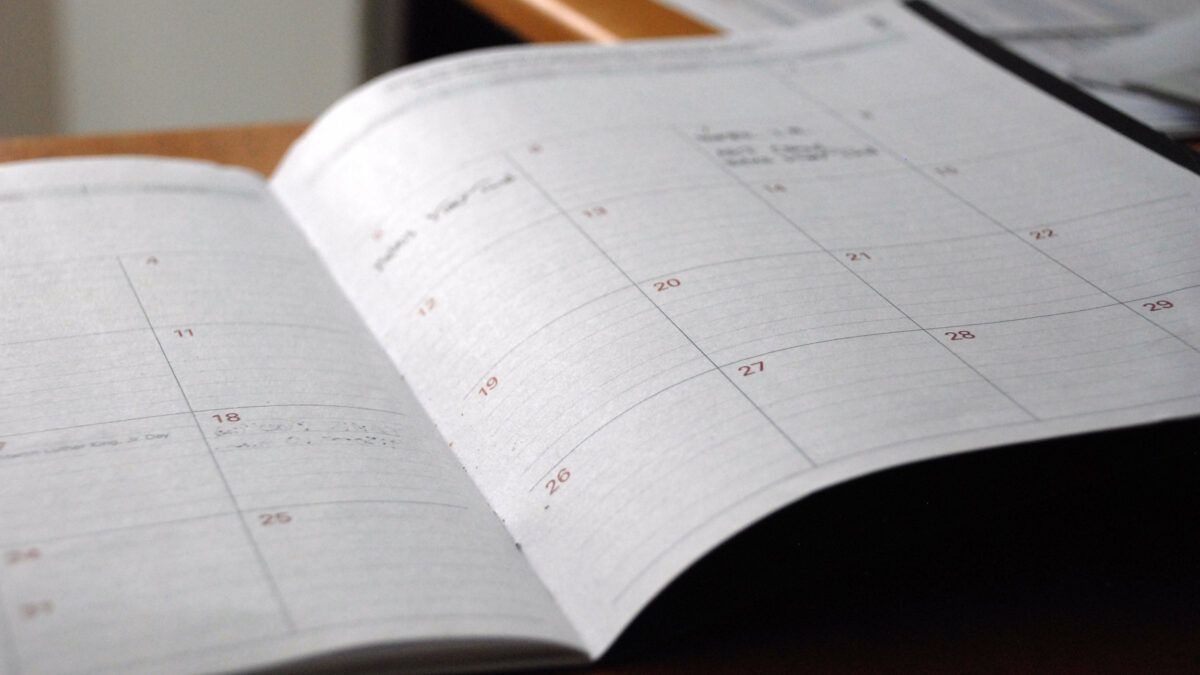
2019 was a big year for IIA and analytics in general. We had made a list at the end of 2018 of the 6 things that we thought would be the most influential and important in the upcoming year - let’s see how we did!
Past Predictions and Priorities
Check out our predictions from last year and read the corresponding blog posts to see what was correct and what changed throughout the year!
Our predictions for 2019 were the following:
1. An increasingly intense focus will be placed on the ethics of analytics
The reality is that most companies haven’t historically had any formal programs and policies around ethics and analytics. There are certainly some hot spots, such as the privacy considerations surrounding sensitive personal data. But, ethics as an overarching theme simply hasn’t been a focus or a priority. Rather, individual people or teams are left on their own to do what’s right. This leads to spotty results and myriad risk points… Click here to read more!
2. Data will continue to gain strength as a differentiator
Not long ago, the role of Data Scientist was what most companies wanted to discuss with me in terms of roles they needed to understand and add to their organizations. Then, the role of Data Engineer became a big topic of discussion. In the past year, there has been a massive increase of attention being paid to yet another role that is still new enough that its title hasn’t been standardized. This role is referred to by a range of names from Analytics Translator, to Analytics Catalyst, to Analytics Liaison, and more. I like Analytics Translator most, so I’ll use that for the remainder of this blog, but there is no guarantee that another title won’t win out in the long run… Click here to read more!
3. The AI arms race will expand in fraud and cybersecurity
The vast majority of people building analytics and data science processes have every intention of being good and ethical. As a result, most potentially unethical and evil processes arise in situations where that wasn’t the intention. The problem is typically that proper focus and governance is not in place to keep analytics and data science processes on the side of good. On top of that, what is good and what is evil isn’t nearly as clear cut as we’d wish it to be… Click here to read more!
4. Analytics and AI teams will merge into one organization
Perhaps the best reason for dealing with all types of analytics through one organization is that the different categories often overlap, and should do so even more than they do today. An analyst hoping to do prediction should, in many cases, explore his or her data with descriptive analytics first. Companies planning to push analytics that make recommendations out to customers or employees—in other words, prescriptive analytics—first need to develop predictive models.… Click here to read more!
5. Model deployment rates will become a big issue
Analytics, we have often assumed, is all about modeling, statistics, and algorithms. The more generous explainers of the field would admit that it is also about data—the integration, cleaning, and massaging of it. Few, however, think first of “deployment” when the subject of analytics is raised. And that is a problem. Great data and fantastic models have no impact on the world unless they are deployed into operational systems and processes. If they’re not used to improve marketing campaigns, decide on optimal inventory levels, or influence hiring processes, they might as well not exist. The same goes, by the way, for AI models and systems. If they’re not deployed they’re just expensive and interesting baubles… Click here to read more!
6. The ascension of citizen data scientists and business analysts will accelerate
You’ve spent a lot of time and energy finding just the right person to fill your new data scientist role. The individual has settled into the position and is proving to be a successful hire for your organization. Looking forward, what can you do to retain the individual and help him or her stay engaged and successful?... Click here to read more!
How Did We Do?
Looks like we were able to correctly predict a lot of 2019’s analytical milestones! What do you think?
Keep an eye out for our 2020 predictions in our upcoming webinar with Bob Morison, Bill Franks, Tom Davenport, and Drew Smith! Register here: http://bit.ly/2Qi9F6M