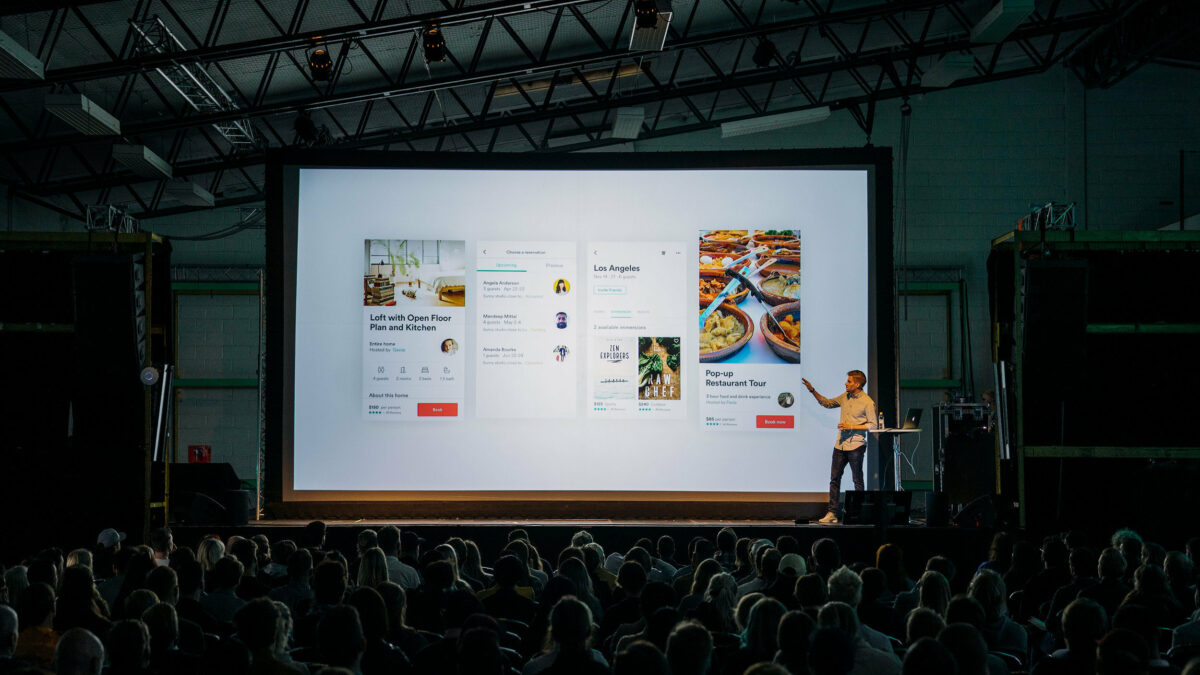
One of the hottest topics in analytics today is marketing attribution. Attribution, for those unfamiliar, is the process of assigning credit to various marketing efforts when a sale is generated. In the modern world, this is no easy task. There are myriad ways to touch a customer today and the goal of attribution is to tease out the impact that each touch had in convincing you to make a purchase.
Was it the email you were sent? Or the Google link you clicked? Or the banner ad you clicked when visiting a different site? Or the ad you saw with your video on YouTube? Or one of many other potential touch points? Or is it a mix? It is quite common today for a customer to have been exposed to multiple influences in the lead up to a purchase. How do you attribute the related sale and profit back to the individual influences?
The question is not simply academic because it has real world consequences. Budgets are set based on performance. So, the person in charge of Google advertising has a huge motivation to ensure that they get all the credit they deserve. Also, accurate attribution will allow resources to be properly focused on the approaches that truly work best. If only it were as easy to attribute credit accurately as it is to make the case for doing it!
COMMON ATTRIBUTION OPTIONS
The most common attribution methods, such as the “last touch” method, are very simple. If the last thing I do before a purchase is to click a banner ad, for example, then the banner ad gets 100% of the credit while any prior interactions get nothing. Sorry, email team, search team, and everyone else! While this method is simple to implement, it doesn’t sit well with analytical minds. As a result, organizations have tried all sorts of different methods. These include:
A uniform credit to all touch points involved.
Set weightings. Perhaps the final touch point gets 3 credits, the first gets 2 credits, and everything in between gets one credit.
Exponential weightings, with a set decay rate. For example, the final touch point is given one unit of credit, the prior touch point gets 1/2 credit, the one before that gets 1/4, and so on.
Weights customized by the perceived power of a given touch point. If email is considered more motivational than a banner ad, email starts with more credit than a banner ad within any of the prior methods.
Weights determined by the parameters from a model that predicts conversion as a function of the touch points involved.
I could go on. But, by now you should get the point that there are many attribution options to choose from. Don’t forget any given vendor’s “secret sauce” option that purports to be superior and that you can only gain access to by paying a large sum of money!
THE LACK OF OBJECTIVE ASSESSMENT CRITERIA
One day, when I was involved in a heated discussion about what the “best” attribution methodology is, something hit me like a ton of bricks. Namely, there is not a quantifiable, objective benchmark against which to measure attribution algorithms to determine which is best.
When building a response model, for example, the measure of success is the ability to correctly identify responses. The better a model can do this, the better it is, and there is no subjectivity involved. If my model does a better job of finding responders than yours, then we would agree that mine is objectively better with no argument. The majority of common analytic techniques applied in a business setting fall into this category.
There are other types of analysis, such as cluster analysis, that don’t have a “correct” answer to compare against and therefore involve subjectivity. Attribution falls into this more murky space. We don’t know the actual and true influence of our various touch points and so have no way to validate if one method is “better” than another. We can certainly identify the extent to which the results of different attribution methods yield different results, but then we’re left to argue subjectively one against the other.
Naturally, you can expect the email team to be convinced that the method yielding the most credit for email is the way to go. Other teams will be similarly biased and there is no objective way to settle the argument. So, often the method that is selected is the direct result of the group that has the most political clout in an organization. What a mess!
A STARTLING FINDING
Suresh Pillai, Head of Customer Analytics & Insights for Europe at eBay, and I both spoke at an event in London recently. He talked about the journey that his team had undertaken to find the right attribution approach. He showed a wide range of attribution options and then demonstrated that, in his company’s case, all of them yielded substantially the same results in aggregate. While the exact percentage of credit to a touch point might vary by a few percentage points, the credit was consistently sized compared to others. In other words, touch point 1 always got about twice the aggregate credit of touch point 2 regardless of how simple or fancy the attribution methodology.
Next, Suresh showed the result that shocked me. He added a comparison bar for a purely random attribution method. Shockingly, the pattern of credit in aggregate for the random model also fell right in line with the others. In other words, you could randomly assign credit and still get substantially the same aggregate answer as with any other option even though the credit for individual cases would vary! This is another case of Occam’s Razor at work!
WHAT TO DO?
My big takeaway is this: Spend less energy battling over attribution formulas. If one formula is politically more acceptable to your organization than others, just go with it. You’ll be getting substantially the same results no matter what you choose.
Naturally, you might question whether or not eBay’s findings would apply to your specific situation. If so, run the simple test that eBay did. Namely, compare the aggregate results of various options and also compare those results to a random allocation. If they are all virtually the same, make a choice that people are comfortable with and move on to other problems.
If you do find that some methods yield substantially different results from a random allocation, you should expect a battle. With no objective way to know which solution is truly best, the winning approach will be that which satisfies the most stakeholders’ personal interests while offending the fewest.
In the end, your organization must agree on an attribution methodology. However, with all the opportunities for analytics to transform your business decision, don’t get distracted with attribution methodology arguments that will have little real impact on your business. Stay focused on things that will truly matter.