
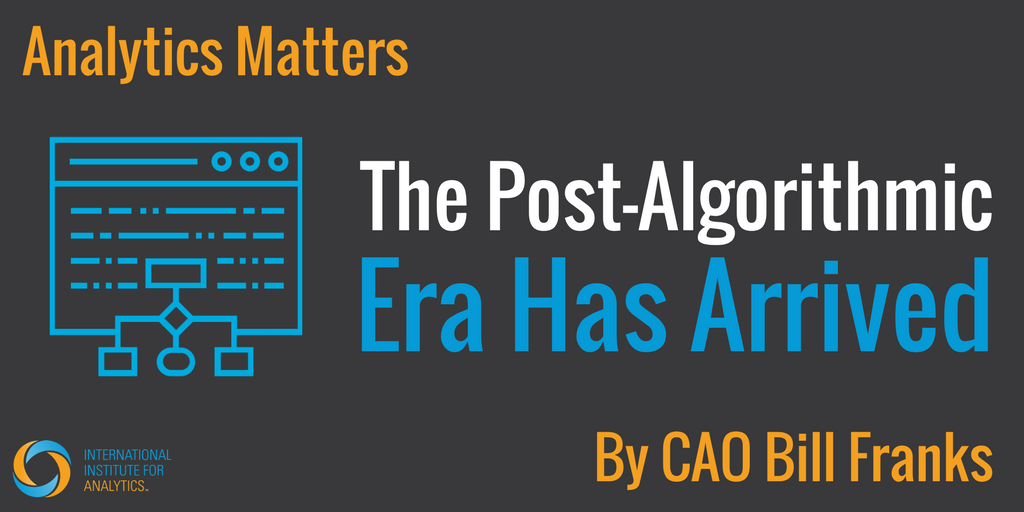
Last week, IIA hosted our annual Predictions and Priorities webinar, as well as the associated research brief. When we sat down to determine what we should focus on this year, Tom Davenport and I both immediately raised a trend that we’ve recently been discussing with organizations. After reconciling our semantics, we realized that we were both excited about the same base trend. I want to reiterate it here as I think it is a critical trend to understand and adapt to. Namely, “the post-algorithmic era has arrived”.
DOES THIS MEAN ALGORITHMS ARE A THING OF THE PAST?
Of course not! In fact, algorithms are being embedded into more and more business processes every day. If anything, analytics processes built on top of algorithms are going to continue to rise in importance for the foreseeable future. If that’s the case, then what does “post-algorithmic era” mean?
The defining factor of the post-algorithmic era is that having access to algorithms, and knowledge of how to execute them, is no longer a differentiator. Not long ago, a large part of the value proposition for an analytics professional was:
Knowing how to use the then-few (and complex) software tools that contained algorithms, and
Knowing from experience which specific algorithms might work best in any given situation
It took a high degree of skill to use the analytics software packages. At the same time, processing was so expensive that it was necessary to limit the options explored. So, analytics professionals with the two listed skills differentiated themselves as highly valuable.
WHAT IS THE NEW REALITY?
The reality today is that algorithms are ubiquitous and widely available. Algorithms are not only available in commercial software, but many algorithms (and the software packages that contain them) are open source and free. Furthermore, it is possible to simply rent algorithms within a simple, user-friendly interface for little cost on the cloud. This is a far cry from the days where major investments in hardware and software were a necessary precursor to even thinking about building meaningful analytics processes. Simply knowing how to access algorithms is not a differentiator today.
Similarly, processing cost has decreased substantially such that today we can literally try every possible algorithm that is appropriate for a given situation and let the results tell us which is best. This has long been a common approach in machine learning. In the past, analytics professionals added value by being able to tightly narrow and target the set of algorithms to try. Today, it is so inexpensive to try all the options that even if you’re confident you’re right about the direction to go, it still makes sense to validate through testing. In practice, knowing what works best (and when) isn’t a differentiator anymore either.
HOW DO YOU ADAPT?
The post-algorithmic era will be defined by analytics professionals who focus on innovative uses of algorithms to solve a wider range of problems as opposed to the historical focus on coding and manually testing algorithms. We can now let cheap processing test all the viable options for a given problem while we consider what to do with the results.
It is critical to note that analytics professionals will still have to ensure a problem is well defined, that the data is properly prepared, and that the portfolio of algorithms being tested is theoretically sound. The fact that the testing of the options will be automated does not negate these base needs.
2018 is the time to begin implementing tools to do the automated testing of algorithms for you. Many tools, both open source and commercial, are available today to enable this. Or, you could build your own. The key is that your organization must move to this higher level of automation to remain competitive in the analytics space.
EVOLVING THE VALUE PROPOSITION OF ANALYTICS PROFESSIONALS
Simultaneously, the value proposition of analytics professionals will continue to evolve. With time freed from basic coding and testing, analytics professionals can redirect that time and energy to solving more problems and operationalizing results. Operationalizing is a critical step that has only recently begun to get the attention it deserves. One implication of this is that business acumen, already an important skill for analytics professionals, will be even more important in the future than it is today.
Analytics has helped to disrupt software, data architectures, and business processes over the past few decades. It is now time for us to disrupt how we in the analytics community do things. This means changing some long standing habits and updating how we define our value propositions.