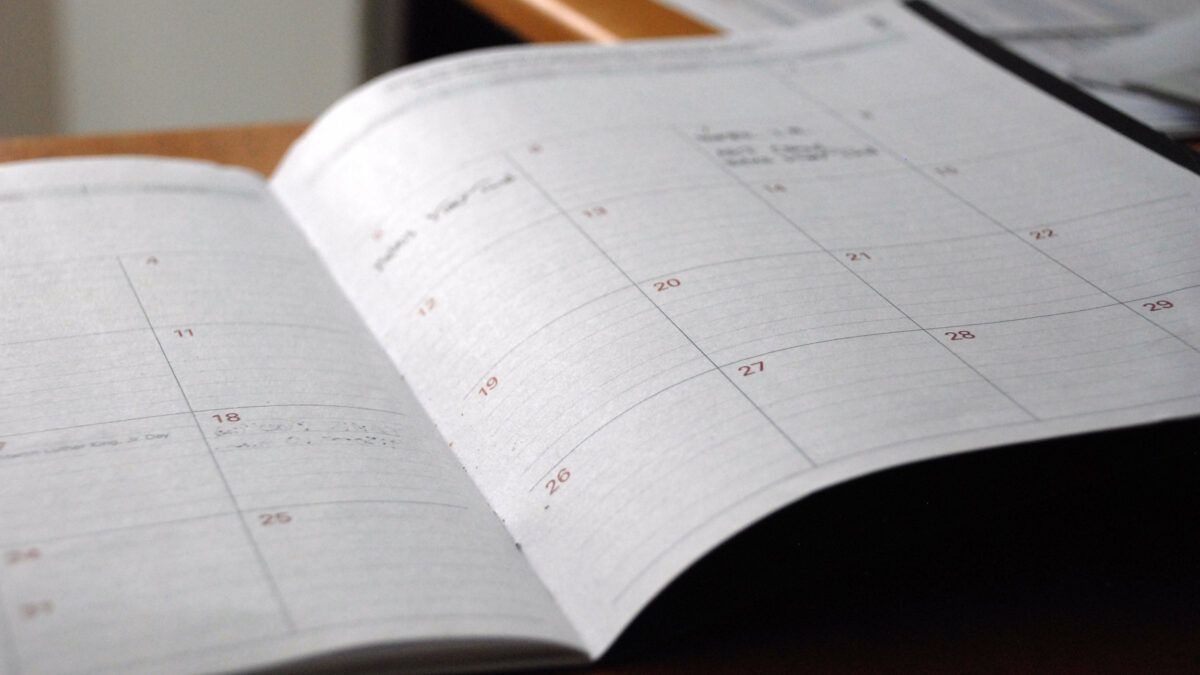
So the question is…when do you sample and when do you not? And does it even matter anymore in the world of big data? As I’ll lay out here, in most cases today there is no point in wasting energy worrying about it. As long as a few basic criteria are met, do whatever you prefer.
First, let’s take care of the cases where sampling just won’t work. If you need to find the top 100 spending customers, you can’t do that with a sample. You’ll have to look at every single customer to accurately identify the top 100. However, such scenarios, while common, aren’t the most prevalent type of analytic requirement. They do represent an easy victory for the “no sampling” crowd, however. Similarly, even a model built on a sample will need to be applied to the universe to use it appropriately. So, when it comes time to deploy, sampling isn’t an option.
Second, let’s remember that many analytic processes are going to deal with or remove outliers and extreme values in some way. As opposed to the “top 100” question above, many of the top or bottom observations may be removed or adjusted so as not to have too much influence. Even if such observations are available in a dataset, they won’t be used.
The point above is important. When building a customer propensity model, for example, you want it to apply broadly to the “typical” customer. Perhaps there really is a customer that spends 1,000 times the next highest customer. Even if true, that customer is so extreme and atypical that you shouldn’t include them in your model. The model is meant to differentiate the masses and a few extreme customers can compromise the power of the model for the purpose it was intended. Any customer who is legitimately that extreme is worthy of special handling from an organization to begin with. You don’t need a model to tell you that.
Last, let’s come back to a typical scenario. You need an average. Or you want to get parameter estimates from some sort of predictive model. Statistically speaking, a sample of sufficient size that is correctly drawn to mimic the population is going to get you the same answer as if you used all of the data. There is no difference between the results from a sample or the universe for most types of metrics and models.
There are those who will vehemently argue that if you don’t need to sample, then don’t. I can see that view. One hole in this view, however, is that a correct modeling process will involve some combination of development and validation data sets…and these are effectively samples anyway! Others will argue that you should only use the amount of data needed and that using more than the minimal sample required is a waste of time and resources. I can also see this view. One hole in this view is that if the resources available can easily handle all the data in a timely manner, then not much is wasted.
Where I net out is that I really don’t care. If someone doing a project for me wants to sample, I’m ok with that as long as the sample is sufficiently large and drawn correctly. If someone wants to use the universe, I’m ok with that too as long as the extra resources required compared to a sample aren’t pragmatically meaningful. I am confident I’ll get the same results, so I’ll stay out of the argument over sampling.
I realize that this position of indifference may concern virtually everyone since most people land on one side of the fence or the other. I guess my point is simply that there are plenty of other, more “meaty” topics to spend time debating when developing an analytic process. I don’t see the use in losing much sleep over whether or not to sample in today’s world. If the systems and tools in use can handle it either way, then I’ll let you have it your way!
One last unrelated note…if you think that you or someone you know might be an analytic superhero, be sure to check out the Analytic Superheroes site!