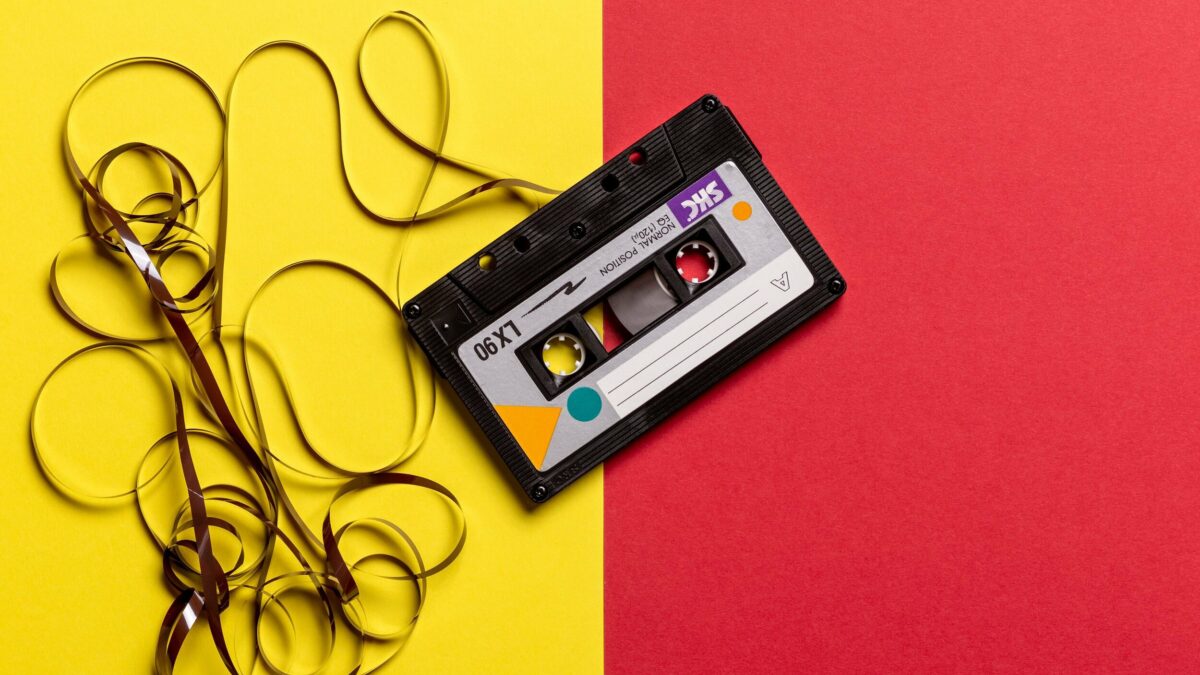
That title probably got your attention and now you think I have some explaining to do! The key word in the title is the word “A”. Self-service analytics isn’t a thing if “a thing” means a single, distinct corporate initiative or set of requirements. Many companies make the mistake of assuming self-service is “a” thing. In reality, it is many things to many people, depending on their skill level and role. In other words, self-service is actually many things. Let’s discuss why!
The Typical Narrow Definition Of Self-Service Analytics
Many organizations think of self-service analytics as being the enablement of business users to handle more analytics on their own. Even within this narrow view, self-service really isn’t “a” thing. It could be argued that companies are pursuing the single, consistent concept of “enabling business users to handle more analytics” . . . and at a high level that is true. However, different business users have different levels of skill and different needs, which leads to different self-service functionality for different people. Enabling business users to have self-service capabilities will require multiple different deployments with varying levels of functionality and complexity. Let’s look at what this means through a couple examples.
Self-service analytics for a marketing manager might mean having access to the ability to report on churn and marketing campaign results without assistance. It might also mean an ability to ask for updated churn or response model output to be generated for an upcoming campaign and for the results of those efforts to be automatically tracked. Self-service analytics for a manufacturing plant supervisor might mean being able to drill into the performance statistics of the equipment in the facility, view and update predictive maintenance scores, and identify patterns that appear abnormal. In both the marketing and manufacturing examples, the same underlying tools and technology might be used, but the specific self-service analytics, reports, and user interfaces created with those underlying tools and technologies will be very different.
Once you think about what was stated in the prior paragraphs, it seems pretty obvious. However, it is not uncommon for organizations to discuss self-service capabilities as though it is a single set of needs until well into the planning process. If expectations and budgets are set under the assumption of a single self-service effort, then one of two things is likely to happen. Either:
The budget and timeline are greatly exceeded once all of the different requirements are finally understood and implemented, or
What is achieved within the allocated time and budget is very much incomplete and underwhelming to the targeted user communities
In either case, it is a black eye to the analytics and IT organizations.
Self-Service Is Broader Than Business Users And Requires IT Partnership
Even sophisticated users want the ability to have self-service, though it will mean different things to them. For example, data scientists want self-service capability when it comes to accessing data to feed their models and push out results. They don’t want to have to rely on IT to execute these core functions. Data engineers want self-service capabilities to access, ingest, and process a wide range of data on their own. They similarly don’t want to have to rely on IT for these basic functions.
Almost everyone across the entire supply chain of analytics wants self-service capabilities that make them more efficient in getting their work done. Users must actively partner with IT to work out the details. It isn’t fair to expect IT to have all the answers about what users need. Rather, users of all skill levels and roles need to let IT know what they are looking for so that IT can implement the right tools to enable that functionality. Only by accurately capturing all of the requirements, and related investment needed to meet those requirements, can realistic costs and timing for self-service implementations be determined.
The ability to have self-service efforts viewed as a success will also increase as people become comfortable their needs have been heard and will be met, even if it will occur over time and in phases. Solid partnering with IT is as important as ever when it comes to self-service.
Self-Service Is Still Not Full Self-Sufficiency!
Another mistake to avoid is the assumption that once self-service capabilities are implemented, the need for support will drop substantively. The reality is that someone will still need to provide training and ongoing technical support for the self-service environments. It is also necessary to have resources available for the inevitable changes and additions that are needed once the environments are rolled out. Aside from that, many issues will arise that simply can’t be addressed solely through a self-service environment. The need for custom and / or more complex processes will always be there.
With a robust self-service infrastructure, more and better analytics can be produced. However, it is unrealistic to expect that everything can be handled through self-service. Neither the users nor their IT support resources should make the mistake of thinking that self-service and self-sufficiency are one and the same.
In summary, self-service is not “a” thing but a collection of things. Self-service does not equate to self-sufficiency. Self-service can only succeed through a strong user base partnership with IT. In the end, by keeping realistic expectations and working together, organizations can achieve a significant degree of progress toward creating more and better analytics through multiple, user-targeted self-service initiatives.
Originally published by the International Institute for Analytics
Bill Franks, Chief Analytics Officer, helps drive IIA's strategy and thought leadership, as well as heading up IIA's advisory services. IIA's advisory services help clients navigate common challenges that analytics organizations face throughout each annual cycle. Bill is also the author of Taming The Big Data Tidal Wave and The Analytics Revolution. His work has spanned clients in a variety of industries for companies ranging in size from Fortune 100 companies to small non-profit organizations. You can learn more at http://www.bill-franks.com.
You can view more posts by Bill here.