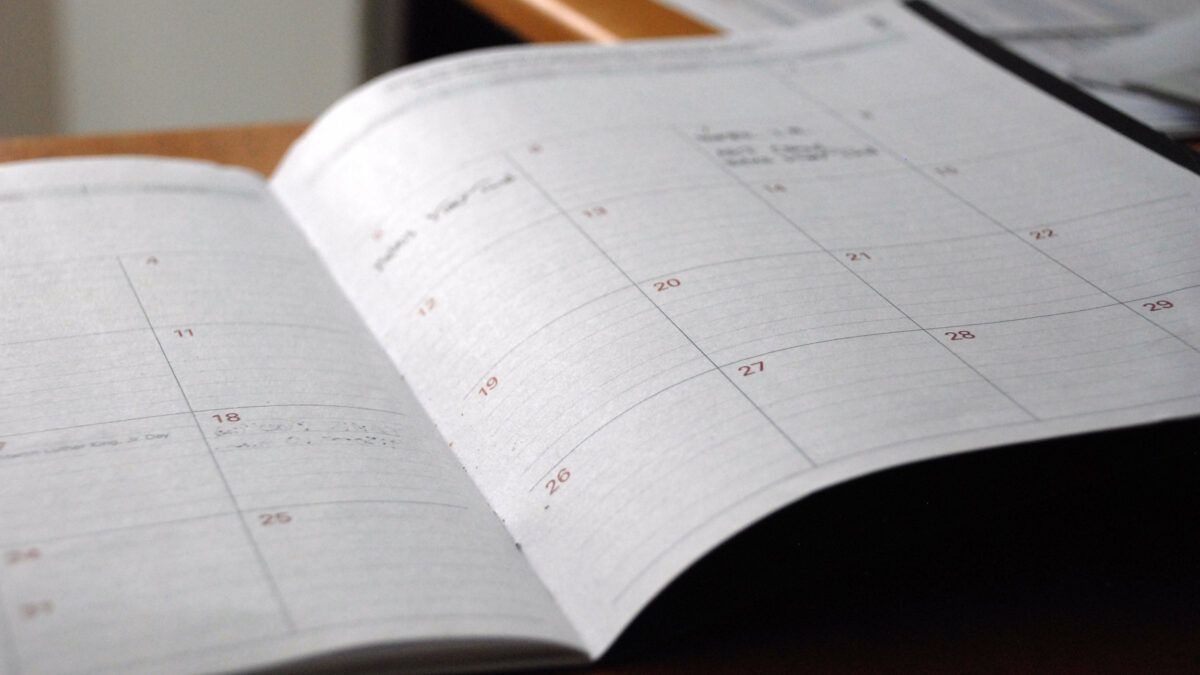
I wrote a blog for Harvard Business Review recently titled To Succeed With Big Data, Start Small. I argued for the need to take small steps with big data rather than going big (pun intended) from the start. I want to expand upon those thoughts here.
At first glance, the idea of starting small with big data sounds like an oxymoron. It just doesn’t sound right, does it? I believe that if you take the time to think about it, you’ll realize that not only is it the way to go, but it is simply an extension of a method that has been successful in working with new data sources for many years. I will illustrate with a few examples.
When I first started doing customer analytics, all we had was a household name and address file. We thought we were pretty cool when we first appended demographic data to that customer list. The size of the data file seemed huge in those days and was quite difficult to deal with. Did we overlay all of the tens of millions of customers as a starting point, however? No, we did not. First, we sent off a sample to be overlaid. We then explored that sample and assessed exactly which data elements were worth the cost and effort based on how they enhanced our analytics. After that assessment, we proceeded with a full overlay. In other words, we started small.
Fast forward a few more years to the late 1990’s and early 2000’s. During this period most organizations first pondered making all of their transactional data available broadly for analysis. Did they get started by buying systems, developing processes, capturing all their transactional data, and then analyzing it? No, they did not. Or, at least the smart ones didn’t. What most did was to capture and make available a subset of their transactional data. Prototype reports and analytics were created against those subsets. Perhaps the subset of data contained one geographic region, or one division, or just one month of data. The prototypes helped the organization prove the value of the data and how it could flow into analytic processes. Equally important, it also enabled them to better understand what it would take to make it available in full on a regular basis. In other words, they started small.
So now we arrive at today. Big data has suddenly risen in profile and popularity. Everyone feels pressure to hop on the big data bandwagon. There certainly is a lot of value to be captured, as I’ve discussed in past blogs. The one flaw I see, however, is that for some reason many organizations are forgetting to apply the simple approach they applied to new data sources in the past. Instead of starting small with big data, they are jumping in all the way from day one. When the historical path to success was not to jump in with both feet from the start, why would you now do so with big data? People need step back, push the hype from their minds, and think things through.
I suspect that part of the problem is the fact that the name big data gets us in a mindset of “big” and we just can’t get out of it. Add to that the fact that many examples that we hear of in the press may not say much about how the organization got started. Therefore, we assume they started out with all the data from day one. I have seen multiple organizations realize terrific success by starting small with big data. As they have moved to capture more of it and put it to further use, they continue to increase the value they are driving. I have also seen multiple organizations struggle as they focus on capturing all the data right away. This takes a lot of time and investment in advance of any value being demonstrated. As roadblocks come along, it only puts the hypothesized benefits further into the future and today’s costs more into focus. It isn’t a recipe for success.
When your organization decides to tackle big data, consider being the champion of the contrarian and somewhat odd sounding view that you should start small.