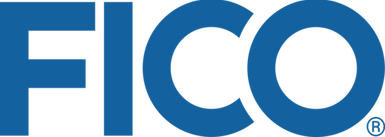
Scott Zoldi is the first CAO we’ve spoken with on the podcast whose company’s product is actually data and analytics. Today, FICO is a household name among consumers, but is also critical to the way enterprises of all sizes conduct business. Over 19 years at FICO, Scott has built a truly world-class data and analytics organization grounded in science and ethics. Yes, ethics. In our conversation, Scott shares the essentials of how analytics is organized at FICO, but also shares how important the proper and ethical use of the data they control is to the company. Stewardship is a growing topic of importance when it comes to all things data, and Scott characterizes the responsibility he and FICO have in very clear terms.
Key Takeaways
Describe your title and role.
As Chief Analytics Officer, I am responsible for machine learning (ML) innovation, analytic IP creation, model development, governance standards, and the development of our analytic products.
Where does the analytics function sit in the organization and who does it report into?
I report into the Chief Technology Officer (CTO). Analytics is core to everything that FICO does. By sitting in the CTO organization, we get an understanding of how analytics needs to integrate with the software related to our human- and machine-interfacing technologies.
Is that the right place for the analytics function to report?
In the past, the function stood alone beneath the CEO. However, as we looked at our business and how analytics and decisioning were merging into one function, we understood that there's a natural interplay between our software and our analytics. We want to ensure that we can deploy analytics solutions properly and efficiently so that they get used. And that's why my organization sits where it does today.
Describe the organizing model you have adopted for analytics.
I have over 100 scientists who are focused on research and development. Beyond that, we have a larger analytics team of roughly 300 scientists who are focused on areas such as analytic services and scores development. What we don't want is 400 data scientists coming up with their own data science twist artistry around how to develop models. We rely on a corporate-wide model development governance process to stay aligned. It is a corporate standard for how models should be developed. It includes standardized IP assets, the model development process, steps for ensuring responsible AI standards, governance around developing models responsibly and ethically, sanctioned variables and features, model architectures that are allowed for certain types of problems, and enforcing things like data and model explainability, including ethics and model monitoring.
What are four or five important qualities and behaviors of analytics leaders?
- Innovation driver. We have a rapidly evolving space with complex data scientists on their own merits, so to be an effective leader, you need to be a practitioner, even at my level, to ensure that you are driving new IP development and innovation.
- Business focus. Data scientists want to see their models integrated into improving business processes. A successful analytics leader needs to be a strong partner with the product and business sides to make sure they're solving the right business problems and, thus, ensuring that the data scientists' efforts are recognized and realized.
- Standards focus. Building models correctly and responsibly is complicated and cannot be left to data scientist artistry. Industry-wide challenges around ethics and explainability must be addressed and iterated out at a corporate level as a set of standards.
- Teaching focus. There's so much that we need to continually learn, whether it be new algorithms or how to develop models that customers can use. This requires a constant focus on the broader picture of how analytics is used in an enterprise framework.
- Curiosity. By looking at data science in a more thoughtful way, we dig deeper in terms of our understanding of how algorithms work and what may go wrong. Curiosity inspires people to think differently and to challenge norms.
How do you measure performance and success when it comes to analytics?
At the team level, performance comes down to adherence to the model governance processes for well-built, explainable, ethical, and stable models. Beyond that, we develop around an Agile process. We have continual reviews, and we focus on model quality and performance.
For external benchmarks, we look at community. Are we part of a dialogue about building models effectively and responsibly? Can we share some of our knowledge with others? Are we a trusted source of advice? We also focus on patent generation, and I'm proud to say that in 2019 I crossed a personal threshold of more than 100 patents. We look at whether the patents uniquely solve certain industry problems, such as financial crimes. For us, patents mean innovation. Ultimately, are we focused on the right things from an innovation perspective, and are our efforts appreciated by our customers?
For more insights from Scott, listen to the full podcast.