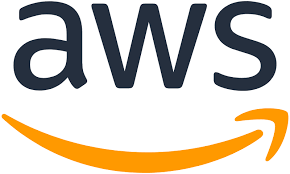
Amazon Web Services (AWS) has become synonymous with cloud analytics and data tools, and Amazon the e-commerce giant continually ranks at the top of IIA’s analytics maturity index. In this episode, I sit down with Rahul Pathak to talk about the evolution of AWS and the impact it has had (and will continue to have) on data science and analytics that are practiced and delivered at enterprises of all shapes and sizes around the world. You will hear quotes from Amazon’s founder Jeff Bezos about the company’s maniacal customer focus, and Rahul gives us a glimpse into the future of how cloud analytics will impact the data science role going forward.
Key Takeaways
Describe your title and role.
I'm the Vice President of Analytics at AWS. I lead product and engineering for a set of teams that build analytics capabilities for customers of all sizes. We have a full range of services like Amazon Redshift and Amazon EMR that companies use to acquire data and build good data foundations to power analytics and machine learning. What we do at AWS, across all of our services, is about how to take away what's undifferentiated from our customers—such as data prep tasks—so they can focus on the bits that really drive business value for them.
Where does the analytics function sit in the organization and who does it report into?
We report up to Charlie Bell, who's the Senior Vice President of AWS. He reports to Andy Jassy, our CEO.
Is that the right place for the analytics function to report?
Yes. We operate as a collection of businesses, so analytics is one set of services. We've got others, for example, in compute, business, storage databases, and so on. Each of these teams is organized independently with a single-threaded owner who's responsible for product and engineering in that area.
Describe the organizing model you have adopted for analytics.
My teams build technology that other teams use to power the analytics that drive their respective businesses. For example, Amazon and AWS are huge customers of Amazon Redshift and Amazon EMR for processing all the data that we generate across both our ecommerce and cloud businesses. Individual teams typically set up their own analytics and use the technologies that we offer. They subscribe to data sets that are kept up-to-date and are scoped down to be relevant for the individual businesses.
What are four or five important qualities and behaviors of analytics leaders?
- Truth seeking. One of our core leadership principles is customer obsession. Understanding what matters to our customers and measuring that and delivering against it. You can't just look for data that confirms what you believe. You have to look at the data honestly, see what it's telling you, and confront things that you don't want to confront in terms of how things are going.
- Curiosity. You have to be willing to drill into the data to understand why it looks the way it does. It's important to balance quantitative analytics with qualitative. We have a view that if the metrics tell you everything's fine, but the anecdotes say something's wrong, then your metrics are probably off. You've got to dig deeper until you understand the root cause of the negative experience that you're hearing about.
- Focus on continuous improvement. Don't be satisfied; let's make it better; and then let's make it better from there, and so on. If you're delivering on behalf of your customers, you're never done. Jeff (Bezos) talked about this in one of his shareholder letters: customers are divinely dissatisfied.
- Velocity. The velocity at which you can act on data matters. If you're getting real-time metrics, but you only meet to make decisions monthly, you're not operating in real time. Shortening that cycle time is important because it'll allow you to be more nimble and agile.
Following up on anecdotes: Occasionally I'll see a customer on Twitter who says something like, hey, I tried to use this, and I had a miserable experience. We try to get in touch with that customer to assess whether the problem is systemic. If it's systemic and our metrics didn't catch it, then we're missing something in how we're measuring the business. We don't want to wait until the signal gets to a threshold that is so large that it's obvious. We look for early signals that something might be off.
How do you measure performance and success when it comes to analytics?
We've seen an exponential explosion in the volume and variety of data that's being generated. So being able to process and analyze this at a performance level, and at a low enough cost, so customers can manage all of this data without having to throw it away is important to us. In each of the services in my area, we're looking at price performance and the maximum scale we can handle. We look at a set of metrics around quality and customer satisfaction, such as availability, response time, and customer experience day-to-day. We look at the metrics that matter to the businesses, whether it's adoption, revenue, growth, or profitability, and we also look at the health of the team—hiring, retention, sustainability.
There are a couple of wins for customers that I'm proud of. Moderna used AWS analytic services as they ramped up their vaccine delivery to get a better sense of the end-to-end supply chain and to iterate faster. That was a cost forward that we were proud to support. And another one in that space was a customer that had to increase ventilator production by about 30x in the early phases of 2020. By connecting the data silos across their supply chain, they optimized production yields for those much faster than they ever had before.
For more insights from Rahul, listen to the full podcast.