Client Inquiry:
How do we develop a culture that will run the business by the numbers, from descriptive and diagnostics through predictive and prescriptive analytics?
Expert Takeaways:
Big Picture
Depending on the type of analytics you’re doing, you’ll need different operating models and org designs.
- Federate descriptive and diagnostic analytics to the business units (BUs). Provide them with self-service technologies and data access so they can answer their own questions.
- The centralized enterprise analytics group is not in the business of helping folks do reporting, with one caveat: when BUs engage on the data science front, you have to meet them where they are, which means if they're still in the BI world, you’ll still need to do some of that.
- For predictive and prescriptive analytics, you need a centralized data science model.
Federated Model
Ideally, every major BU uses the same data, tool stack, or enterprise data warehouse, and the same enterprise self-service tool stack that IT manages. The self-service tool stack, such as Alteryx, Tableau, or PowerBI, allows data analysts to produce their own data pipelines and visualizations.
- Walk along this journey with IT, because you have to manage licensing and build a governance practice. When you allow people access to data, you must govern that access and what can be done with the data.
- This process still begins with the central team—embedding your analysts in the BUs, teaching local analysts how to fish, and working with leadership to staff their own teams that will use the centralized set of infrastructure and data.
- Starting out, engage with a senior leader in a BU. Send in one or two analysts to work on projects that drive a lot of pain for the leader. These analysts still report to you.
- You’ll need a strong data engineering/data analyst skill set on your team that you can embed in other organizations. You also need a strong partnership with the group in charge of your enterprise data warehouse or data assets.
Data Science Pods
Do not federate predictive and prescriptive analytics because the data science skill set is expensive. Deploy and maintain it strategically.
- Create pods that consist of a consultant, data scientist, and analyst. From the IT side, include a data engineer and platform engineer. It’s helpful to have a matrixed (dotted-line) team within IT that is dedicated to data science.
- Data science consultants are former data scientists who excel at understanding business needs and translating that into data science products. Some data scientists prefer heavy quant development; others may move into the consultative role.
Deploy the pod in three different ways: project-based, partnership, and embedded.
- Project-based engagement: A BU has a one-time data science project. You bid on the project the same as any third-party vendor. Once you’ve completed the data science product, you get it into production and move on.
- Partnership engagement: Some BUs may have data scientists, but they don’t have consultants or data engineers. Supplement the necessary resources from the central team. The BUs still manage their own work.
- Embedded engagement: For BUs that have many years of work from reporting to advanced machine learning and AI capabilities. Build them a roadmap strategy and hire their analytics staff that you deploy into the BU. The BU manages the day-to-day work aligned to the roadmap.
Expert Network
IIA provides guided access to our network of over 150 analytics thought leaders, practitioners, executives, data scientists, data engineers with curated, facilitated 1-on-1 interactions.
- Tailored support to address YOUR specific initiatives, projects and problems
- High-touch onboarding to curate 1-on-1 access to most relevant experts
- On-demand inquiry support
- Plan validation and ongoing guidance to advance analytics priority outcomes
- Monthly roundtables facilitated by IIA experts on the latest analytics trends and developments
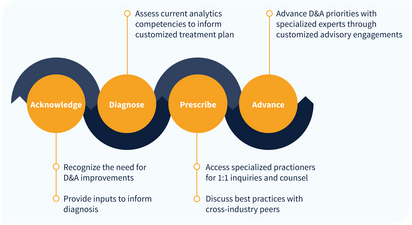