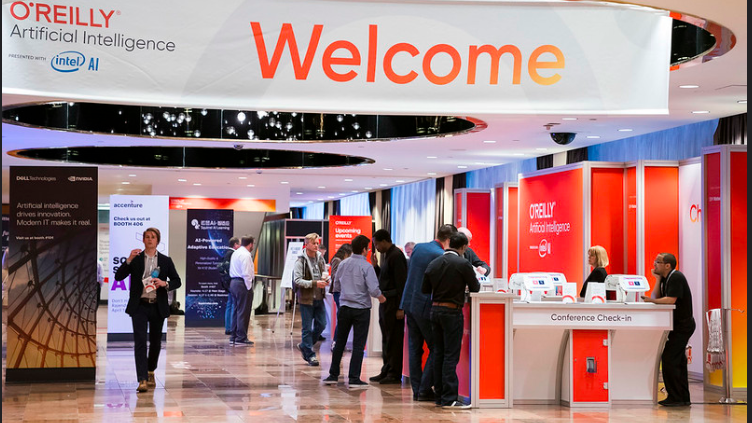
Last week, IIA attended the O’Reilly AI Conference (#TheAIConf) in New York City. The O’Reilly AI Conference continues to provide an informative and comprehensive overview of artificial intelligence and its accelerating transition from research to industrialization. Sessions covered a broad spectrum of AI topics, including cutting-edge research, open source tools, regulatory considerations, use cases and best practices for implementation. This conference continued to include the AI Business Summit, focused on exploring the AI challenges, opportunities and risks from the enterprise perspective. Over 2,000 people attended the conference.
The Wednesday and Thursday morning keynotes showcased all these themes and included the following highlights:
AI Adoption in the Enterprise
Conference chairs Ben Lorica (Chief Data Scientist, O’Reilly) and Roger Chen (CEO, Computable) outlined some of the findings from O’Reilly’s recently released “AI Adoption in the Enterprise” market study. This research divided companies into three stages of artificial intelligence (AI) adoption (“Not yet using AI,” “Evaluation Stage” and “Mature Practice”) and exposed some significant differences between AI leaders and AI laggards.
For example, 43% of AI leaders are investing more than 20% of their IT budgets toward AI projects, while 80% of laggards plan to invest at most 5% of their budgets toward AI projects. Leaders and laggards also face different bottlenecks when it comes to AI adoption. Laggards cite company cultures that don’t recognize the needs for AI (22%) and difficulties in identifying appropriate use cases (21%) as major bottlenecks. Leaders identify lack of data or data quality issues (26%) and lack of skilled people or difficulty hiring the required roles (24%) as major bottlenecks.
However, when it comes to machine learning (ML) and AI adoption skills gaps, all groups face similar challenges and the same top four skills gaps: lack of ML expertise, understanding business uses cases, data engineering and compute infrastructure. A complete copy of the market study can be downloaded here.
Computational Propaganda
In his keynote “Computational Propaganda,” Sean Gourley (CEO, Primer) discussed how advances in AI capabilities can be used to manipulate public opinion and threaten democratic institutions.
Sean began by noting that the financial services industry is being dominated by algorithms because algorithms are faster than humans. Although these algorithms have brought dramatic improvements in market efficiencies, they have also lead to unexpected events like the 2:45 Flash Crash. This paradox is summarized by Wiener’s Laws, which generally state that automation will routinely tidy up ordinary messes, but will occasionally create an extraordinary mess.
The significance of Wiener’s Laws will grow as we enter the world of creative machines. AI is moving beyond the ability to understand language, recognize objects and recognize emotions. AI now has the ability to create new and unique worlds.
As an example, Sean discussed how generative adversarial networks can be trained to create hyper-realistic images of imaginary celebrities that have never existed (see examples here). These abilities are also extending to natural language generation where advances in fact-aware language generation can automatically create factually accurate biographies of individuals and research papers including citations (see examples here).
All these advances are leading to the advent of Computational Propaganda where the ability of machines to generate images and language that we can’t tell from reality makes it increasingly difficult to know what is real and what is fake. To emphasize his point, Sean walked through several real-world examples of increasingly sophisticated applications of social bots by state and nonstate actors, including Russia. Russia has deployed bots to shape public opinion on Ukraine, Brexit and the 2016 U.S. presidential election where 400,000 bots were deployed in organized networks. This capability extends beyond Russia, and there are now 48 countries spending $500M+ per year on the ability to wage disinformation campaigns using these technologies. In conclusion, Sean states his belief that democracies will need to regulate the use of these technologies and will have to walk a fine line between censorship and freedom of speech.
Real-World AI Success Stories
Several keynotes highlighted successful, real-world implementations of ML and AI technologies. These presentations demonstrated the maturation of these powerful technologies while also showing that their mastery takes considerable engineering capabilities and investment.
Mastercard
During his keynote, Nick Curcuru (VP of Data Analytics and Cyber Security) discussed MasterCard’s growing investment in AI. Mastercard has 2.5 billion customers that process 74 billion transactions per year. As fraud threats have become more sophisticated, Mastercard is turning to AI to protect customer data. Mastercard uses AI to provide real- time credit intelligence using hundreds of data points, to provide passive biometric identification for additional security and in its anti-money laundering monitoring. As an example, Mastercard has used AI to increase the number of data points used in card charge authorization from 15 to over 150 in five years.
Intuit
Desiree Gosby (VP of Identify and Profile) discussed how Intuit’s TurboTax unit is using advanced text analytics and image recognition to automatically generate tax returns using mobile phone pictures of tax documents.
Her keynote highlighted both the ability of AI to create disruptive new services and the practical challenges and sophistication required to successful deploy AI into production, which often requires the ability to combine multiple, advanced techniques.
For example, it is difficult for consumers to take high-quality, easy-to-analyze images. Intuit had to deploy a variety of image techniques (edge detection and brightness/focus/ contrast detection) and Convolutional Neural Networks (CNN) models (foreground detection and image-quality threshold) to provide feedback and get usable images. Next, they had to implement multiple techniques (document classification using CNN models and layout matching using Recurrent Neural Networks (RNN) models) to determine the type of tax form and manage the format variability of many forms. Finally, they had to extract the tax information using traditional optical character recognition coupled with natural language processing, entity recognition modeling and contextual random field modeling.
Kim Hazelwood (Senior Engineering Manager, AI Infrastructure) presented how Facebook uses AI and ML to create highly customized experiences for its 2.8B subscribers. Facebook deploys a variety of ML models across its services and features. Multilayer Perception models are used for search ranking, news feed ranking and advertisement displays. Support Vector Machines and CNN are used for automatic facial recognition and image tagging while RNN are used for language translation, speech recognition and content understanding. All these algorithms are deployed at a massive scale. Facebook does over 200 trillion predictions per day and does over 6 billion language translations per day.
To support ML operations at this scale, Facebook invests heavily in infrastructure, platforms and frameworks. It has developed custom CPU, GPU and storage servers for data manipulation, training and inference, which it has open sourced through Open Compute. Facebook has developed an internal platform called FB Learner, which facilitates feature engineering, training and deployment. From a framework perspective, Facebook has developed (and open sourced) PyTorch 1.0, which combines features of Caffe2 and the original PyTorch.
Netflix
Tony Jebra (Director of Machine Learning) from Netflix discussed how his company uses ML to personalize its service for its 140 million subscribers. ML is deployed to optimize almost all the customer-facing experience, including ranking, page generation, promotion, image selection, search, messaging and marketing.
Tony demonstrated how Netflix uses contextual bandits, instead of batch machine learning, to interleave learning with data collection and to personalize content artwork for each subscriber. A separate ML system selects the content that should interest a subscriber. For each piece of content, Netflix has multiple artwork options that highlight unique elements of the content (genre, themes, actors, age, etc.). Subscriber information, including viewing history and country, is used to choose which artwork to display for a piece of content. As an example, Tony showed how artwork displayed for the Netflix show “Stranger Things” is personalized based on your interest in horror films, sci-fi films or teenage dramas.
Contextual bandits differ from supervised learning techniques in that the system is never given the correct answer, it just knows if an answer is right or wrong and adjusts accordingly. This allows it to be deployed into production environments and iterate toward the best option based on user response to the image.
Conclusions
Many AI technologies are rapidly maturing, and the pace of innovation continues to accelerate. These technologies can be deployed into production environments to generate real differentiation and competitive advantage. However, enterprises must be prepared to invest and develop new capabilities if they are going to successfully deploy them. As companies struggle with these decisions and to keep pace with these developments, events like the O’Reilly AI Conference can provide analytics leaders with valuable insights and an understanding of where the market is going.
O’Reilly AI Conference Resources