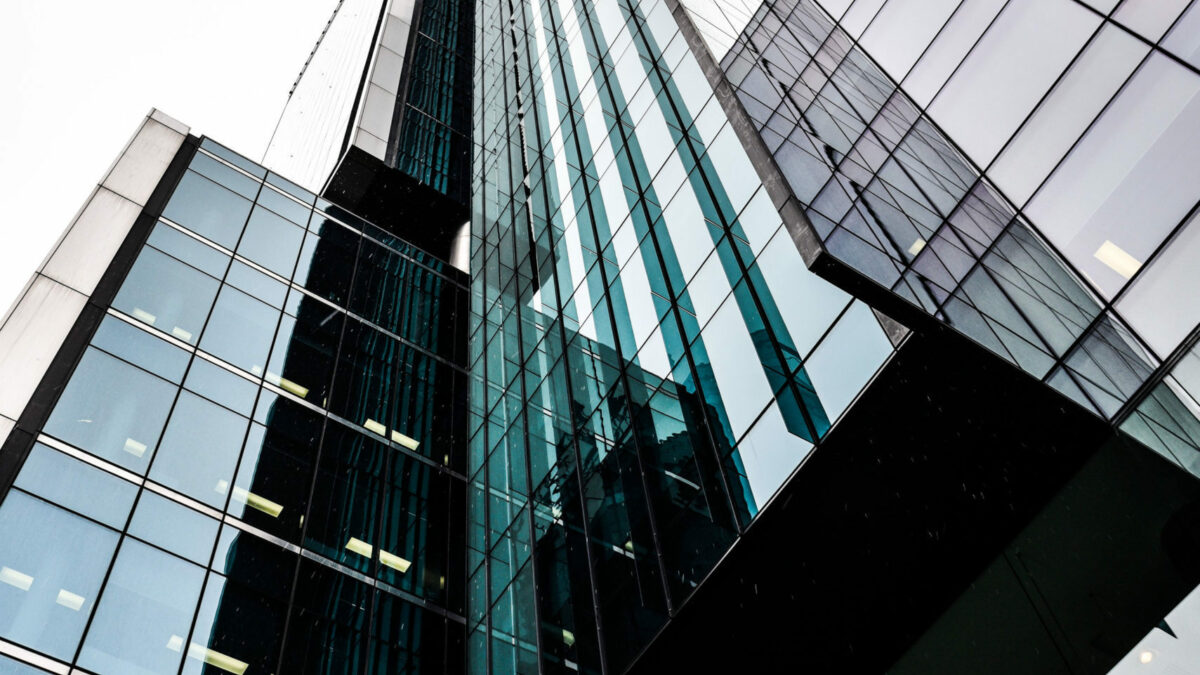
For about a decade now I have been observing – as a researcher, not a consultant – the analytics and automation efforts at Schneider, where these fields have been at the forefront of operational improvements. Schneider is one of North America’s largest truckload, logistics and intermodal services providers. It’s a complex business, moving close to 18,000 daily loads, utilizing over 13,000 drivers and 50,000 trailers/containers.
To manage and optimize this complexity, Schneider has been implementing various forms of analytical decision-making for a couple of decades. The analytics are increasingly automated as well, from guiding order acceptance decisions to recommending optimal appointment times to automatically matching loads with drivers. Each hour, Schneider’s planning systems evaluate millions of potential driver tours over a multi-day horizon. Future plans include enhanced use of weather and traffic data.
Zahir Balaporia, who until recently directed Advanced Planning and Decision Science for Schneider, led the operations research group that develops the optimization systems. He says that his team thought in terms of automation because it forces them to push the limits of their systems and process capabilities. In principle, the ultimate degree of efficiency comes when no human intervention is required. However, uncertainties in the data results in a process that often cannot be fully automated, but can be significantly augmented.
The analytical systems have certainly changed the jobs of Schneider’s people, but it hasn’t eliminated them. Overall it’s made them more productive and able to coordinate more activity. And thanks in part to Schneider’s growing business, it hasn’t led to any layoffs.
Travis Torrence’s job is a good illustration of what is happening to jobs with more automated systems in the workplace. He’s an Atlanta-based “Intermodal Dispatch Analyst” at Schneider and has had the job for a couple of years. During his time, Schneider introduced a new version of its dispatch optimization system, Short Haul Optimizer (SHO), which matches container loads to available drivers. It’s Mr. Torrence’s job to work with the SHO system to dispatch the markets he manages. He is also required to monitor its capabilities and encouraged to recommend ideas to the operations research group that works on improving the SHO algorithms. Doing this is well within his capabilities, however; he has a degree in business with a focus on logistics and information systems.
So does Mr. Torrence just sit back and watch the machine work? Hardly. While it has definitely made him more productive in his job – he can dispatch about 75 drivers a day using the systems, which is about double what he could do previously – there are numerous tasks that still require a smart human.
One of the biggest issues is data quality for the information in the system. The optimization system usually comes up with great results, but it requires correct data about when drivers are actually available, how much time they have to drive, when trains are actually arriving, ramp congestion and so forth. There are systems for all of these bits of information, but reality is sometimes different from what the system says it is.
On a typical day, for example, Mr. Torrence might start by taking a look at what the SHO system is recommending for the day. He would then check the quality and currency of the data, particularly with regard to the drivers. He might contact some drivers to nudge them into adjusting their available times in order to make for a better fit with available loads. He might contact Customer Service to see if there is any customer flexibility on delivery windows. He might monitor traffic information and weather to see if driving times are reasonable. He might examine the total set of loads going out to see if congestion at the ramp will be a problem.
Mr. Torrence had the job before the new SHO system was put in place and it’s been a dramatic change in his role. Even though some key decisions – at least the initial cuts at them – are made by the system, he says the job is definitely more interesting than it was before SHO was implemented. “You’re not bogged down in covering one load,” he commented. “It gives me an opportunity to explore other areas of the process, and to learn more about how the data is coming in and make it more accurate. Since I’m not looking at every single load anymore, I can focus on the bigger picture now.”
Mr. Balaporia noted that his team had often concluded that augmentation – combining smart humans and smart machines – is a more pragmatic approach than automation. “The original strategy on SHO was an automation strategy,” he said. “As we got into the implementation, the operations research team got closer to the operational details and data quality issues by working closely with folks like Travis. Understanding those details made it more practical to pursue an augmentation approach. But [they] continue to look for ideas to automate, which will allow the dispatch analyst to focus on broader systemic issues.”
I believe that Travis Torrence’s job is typical of what will happen in many companies with increased analytics and intelligent technology. The jobs of knowledge workers will not be automated, but augmented. Increased productivity may well mean that employment growth in many jobs slows, but relatively few knowledge workers will lose their jobs entirely. Work will change in many ways, but those who take on new tasks are likely to retain their jobs, and even find them more stimulating and satisfying. Schneider even added more analytical requirements to the position and changed the job title to Intermodal Dispatch Analyst.
It won’t be a picnic, however. In order to succeed, the workers in these new types of jobs will have to work to understand how intelligent technologies work and adapt their performance to them. As Mr. Torrence did, many will have to get formal training in information systems and learn about new technologies that augment their capabilities to perform their jobs. Smart machines will be an integral component of many jobs in the future. The successful knowledge workers of the future will have made them their close colleagues.
Originally published in WSJ’s CIO Journal.