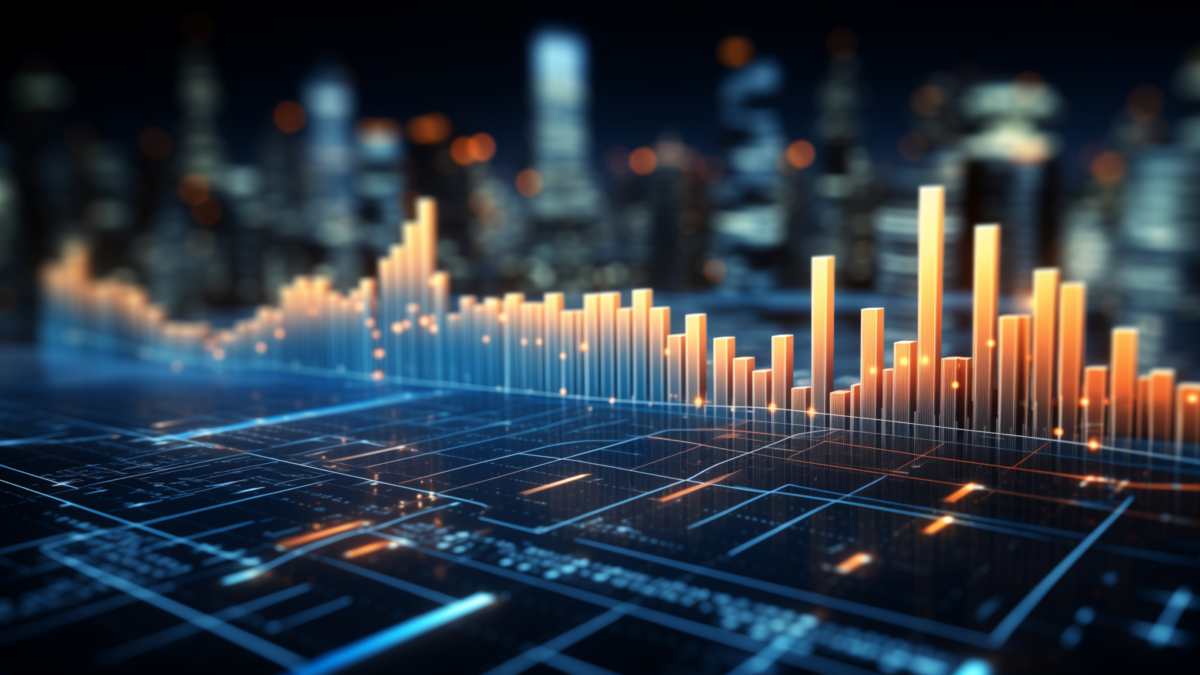
What are the common hurdles encountered when putting analytics to work in a business, both in developing analytical models and applications and in building enterprise analytical capability?
This question is central to IIA’s mission of helping organizations navigate the many challenges to achieving analytics maturity.
Succeeding with analytics, and sustaining that success, is complex. It requires both a multi-pronged approach and an awareness of the pitfalls that analytics leaders and teams often face along the path toward increased analytics maturity.
In the third installment on our series on some of the biggest obstacles data and analytics organizations face today and ways to overcome them, we discuss data and technology challenges.
1. Data Governance: Insufficient and Inflexible
Data-related challenges, spanning data quality, security, and lineage understanding, have elevated the importance of data governance. Large companies prioritize data governance to prevent chaos as data volumes, analytics deployments, and scalability increase. While data governance tools and policies have evolved, many companies lag behind. Some rely on inflexible systems that struggle to adapt, leading to costly re-dos when requirements change. Others implement partial governance environments, leaving gaps that invite security risks and data quality issues. While not the most captivating investment, robust data governance is essential for safer, cost-effective, and scalable analytics pursuits, akin to eating vegetables for a healthy analytics capability.
Warning Signs:
Some of the common warning signs in this area include:
- Users complain about the lack of ability to find and use data
- Allocating permissions and tracking user activities is difficult and time-consuming
- Enabling new requirements and use cases requires extensive governance work
Overcoming Data Governance Challenges:
It is critical to govern data from the moment it is ingested. This governance includes lineage tracing, data quality processing, application of security, and usage tracking and profiling.
- Elevate data governance to a tier 1 focus level, which includes investing in enterprise-level data governance solutions that assist with all aspects of data governance.
- Ensure that corporate leadership understands that proper governance is a necessary component of a successful enterprise analytics program. While it can bring substantive costs initially, the benefits that solid data governance provides will be worth it in the long run.
The ability to quickly identify the impact of a breach, or to flag an unexpected change in a data feed, or to deploy a novel process with minimal effort all make the investment worth it.
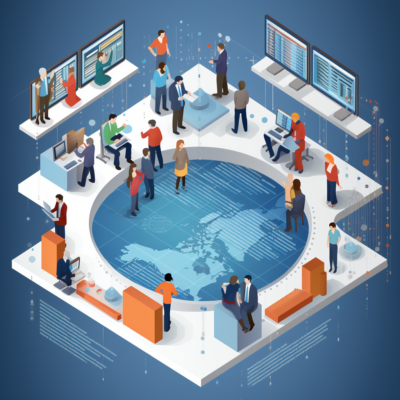
A Comprehensive Guide to Responsible Analytics Governance - Complimentary Research Brief
In order to guide you on your digital ethics journey, experts at IIA have crafted this complimentary version of our research brief, "A Comprehensive Guide to Responsible Analytics Governance." This brief lays the foundation for operationalizing analytics governance across all business functions in the enterprise and will help you:
- Frame analytics governance within the context of your business model and values
- Build out an analytics program that is a natural part of digital ethics
- Consider the dimensions of process, people, and technology when creating your analytics governance program
2. Analytics Platform: Cloud Adoption Struggles
The cloud has undeniably cemented its place in enterprise computing, even within traditionally cautious sectors like healthcare and finance. Despite its widespread adoption, migrating to the cloud poses significant challenges. Companies grapple with retiring legacy systems, prioritizing data migration, and adjusting analytical processes to fit the new cost structure. While some anticipate and adapt to these changes effectively, others find themselves unprepared, facing surprise setbacks and the need for recalibration as they navigate the complexities of the cloud environment.
Warning Signs:
Some of the common warning signs in this area include:
- No thorough and comprehensive migration plan, yet lots of “let’s get the migration started” pressure
- Users have not been given training on how to best utilize and optimize a cloud environment
- There is limited focus on, and acknowledgement of, the substantive effort a cloud migration will require
Overcoming Cloud Challenges:
A variety of threads need to be pursued in order to have a successful migration:
- Create a logical prioritization of data sources and analytical processes to be migrated.
- Undertake substantial planning for user readiness and training to ensure that people know how to optimize processes in the new environment.
- Third, a plan for detailed testing of cloud processes to enable decommissioning of current systems is necessary to avoid paying for multiple platforms longer than necessary.
- Last, the organization should expect issues to arise and progress to be slower than desired at times. There will always be unexpected complications in an undertaking as complex as a cloud migration.
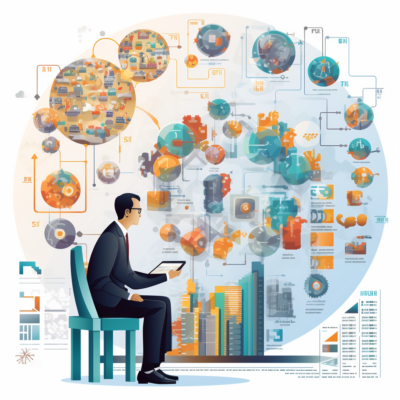
Analytics and AI Strategy eBook
Unfortunately, even in 2023, we're still seeing analytics leaders struggle to deliver measurable returns on analytics investments. To deliver value on your analytics and AI efforts, D&A leaders must start with a robust strategy, and we can help you get started. Our free Analytics and AI Strategy eBook is an easy-to-follow guide that takes you through the entire process of creating an effective strategy - from identifying key areas to address to crafting a comprehensive plan and disseminating and monitoring your strategy.
3. Inertia: Failure to Embrace New Algorithms and Approaches
Analytics organizations need to constantly look for new algorithms and analytical approaches to lay on top of their corporate platforms. Perhaps no analytical trend has been as big as the rise of artificial intelligence (AI). Just a few short years ago, only cutting-edge organizations were experimenting with AI. Today, cutting-edge organizations have a lot of AI processes deployed and many mainstream companies are also actively experimenting with, and beginning to deploy, AI processes.
While AI certainly won’t apply to every business problem, organizations shouldn’t fail to add AI capabilities to their arsenal of options. The top reasons some companies fail to pursue new approaches like AI include:
- A company may still be struggling with enabling traditional analytics, let alone trying to move into AI
- The lack of transparency within AI algorithms can pose an issue, especially in regulated industries
- Finding people with the right skills and experience to build and deploy AI is challenging
Many of the challenges aren’t as specific to AI as they are to the pursuit of any novel analytical approach. It takes concerted effort, planning, and focus to incorporate new algorithms into the organization’s analytic arsenal.
Warning Signs:
Some of the common warning signs in this area include:
- There is interest in AI but no firm plans or strategies to pursue it in earnest
- Analysts want to focus on using just the algorithms and approaches they know best
- People are impressed with what other companies are doing with new algorithms but there is no action to mimic that success
Overcoming AI Challenges:
Like the cloud, AI is here to stay. Every organization needs to explore where AI fits within its analytical requirements and then develop plans and timelines to incorporate it.
- Success will require both upskilling and training for current employees as well as the hiring of new employees who bring the right skills with them.
- Don’t let resistance to change derail progress. Don’t think of AI as a one-off. It is yet the latest round of new algorithmic approaches going mainstream. In the past, basic predictive modeling and then machine learning challenged analytics organizations. Today it is AI. Tomorrow it will be something else.
There must be a generalized strategy to encourage pursuit and implementation of new algorithms and approaches as they become available. AI just happens to be today’s example.