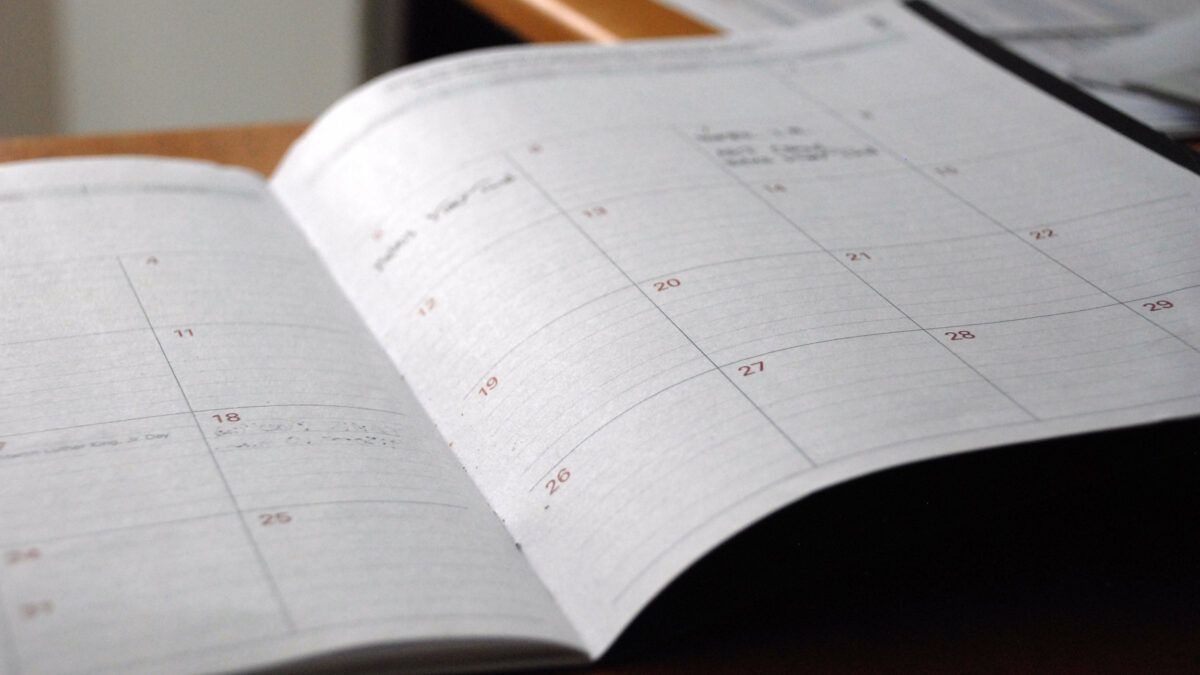

As a person who has been involved with analytics for a long time, I have historically considered analytics to be a huge differentiator while data was more of a table-stakes enabler. Several trends have come together to make me realize that the equation has been reversed in many cases today.
DATA AS ENABLER, ANALYTICS AS DIFFERENTIATOR
Before the era of big data, most enterprises captured similar data in similar ways. Every retailer captured the same transactional history, every bank had the same account activity history, and every telecommunications company had the same call detail records. The data itself was fairly standard and didn’t provide a competitive advantage on its own. By definition, if every competitor has the same data, then the data doesn’t differentiate them from one another.
Given the similarity of data assets, differentiation came from how different organizations analyzed the data that they possessed. In the retail space, for example, Tesco had a well-documented period where it got far ahead of the competition in the realm of customer analytics and reaped huge rewards as a result. Part of what drove the ability to differentiate with analytics was the fact that access to the algorithms needed for analytics was expensive and required specialized tools and skills. Even once an organization realized what a competitor was doing, building similar capabilities required an expensive effort to create similar analytics processes in-house.
ANALYTICS DIFFERENTIATING LESS
A few months ago, I wrote about how the post-algorithmic era has arrived. The point of the blog wasn’t that we didn’t need analytics and algorithms anymore; rather, because we need them so much and demand is so high, they have become pervasive and inexpensive. Virtually any required algorithm can be easily accessed for a reasonable cost.
Now that algorithms are so widely available, it is harder to keep a competitive advantage by simply applying algorithms to the same data that the competition also has. No matter how sophisticated an organization’s analytics processes might be, at some point someone is going to make a very similar process available. That will take away the competitive advantage that the analytics provide. The world of chatbots is a good example of this. While chatbots continue to rise in prominence, the reality is that chatbot functionality will largely be a utility that is rented. Unless an organization has a very specialized business where customized training to understand uncommon terms and themes can be an advantage, it will be better to rent a chatbot service than to try and build one. Standard chatbot functionality, while impressive, won’t differentiate.
ANALYTICS AS ENABLER, DATA AS DIFFERENTIATOR
One implication of this new world where everyone has access to algorithms is that a new arms race has started in the realm of data collection. If the power of algorithms alone is no longer a guaranteed long-term differentiator, then it makes sense to pursue novel data sources – and the analytics those data sources will enable – in order to differentiate. This is exactly what is happening today.
I had the pleasure of hearing several venture capitalists describe their view of the analytics and artificial intelligence market when they served as guest speakers for IIA’s Symposium and Analytics Leadership Consortium meetings in April 2018. Joanne Chen of Foundation Capital, Yujin Chung of SignalFire, James Hardiman of Data Collective, and Kevin Zhang of Bain Capital Ventures all pointed to this trend in their own way. When looking to invest, the venture capitalists look for novel data as a priority over novel analytics. The reason is that they fear the analytics will be fairly easy to replicate in today’s world whereas a differentiating data source can pose huge barriers to entry for the competition.
In the self-driving vehicle space, for example, there are several companies focused on providing training data for the vehicle manufacturers. The manufacturers all need the same training data, but it is expensive to create and collect. So, the manufacturers focus on the vehicles while outsourcing training data generation to vendors who specialize in it. After a few providers have huge libraries of training data, along with established relationships to sell that data, the cost to new market entrants will be formidable.
The holy grail is a situation where an organization has the ability to combine data beyond the competition with analytics that also go beyond the competition. For example, capturing telemetry data from a video game beyond what the competition captures, analyzing it, and then customizing each player’s game experiences is a powerful combination of cutting edge data and analytics coming together to jointly drive competitive advantage.
HOW TO RESPOND?
Once again, I’ll reiterate that analytics and algorithms are still a critical component of any plan to continue evolving a business in the coming years. But, the new reality is that the analytics themselves are becoming easier and cheaper every day to replicate. As a result, focus must also be put on identifying and analyzing novel data sources that others do not yet have. That then causes a two-layer barrier to entry. First, a competitor must collect the data. Then, they must build the analytical processes on top of it.
A major implication of these trends is that any data that comes with high cost and/or complexity to collect should be considered a valuable asset. It should not be shared without appropriate compensation for the unique value it may contain. In addition, focus should shift to differentiating an organization through a combination of novel data and novel analytics. The historical capability to differentiate purely based on better analytics against table-stakes data is rapidly disappearing.