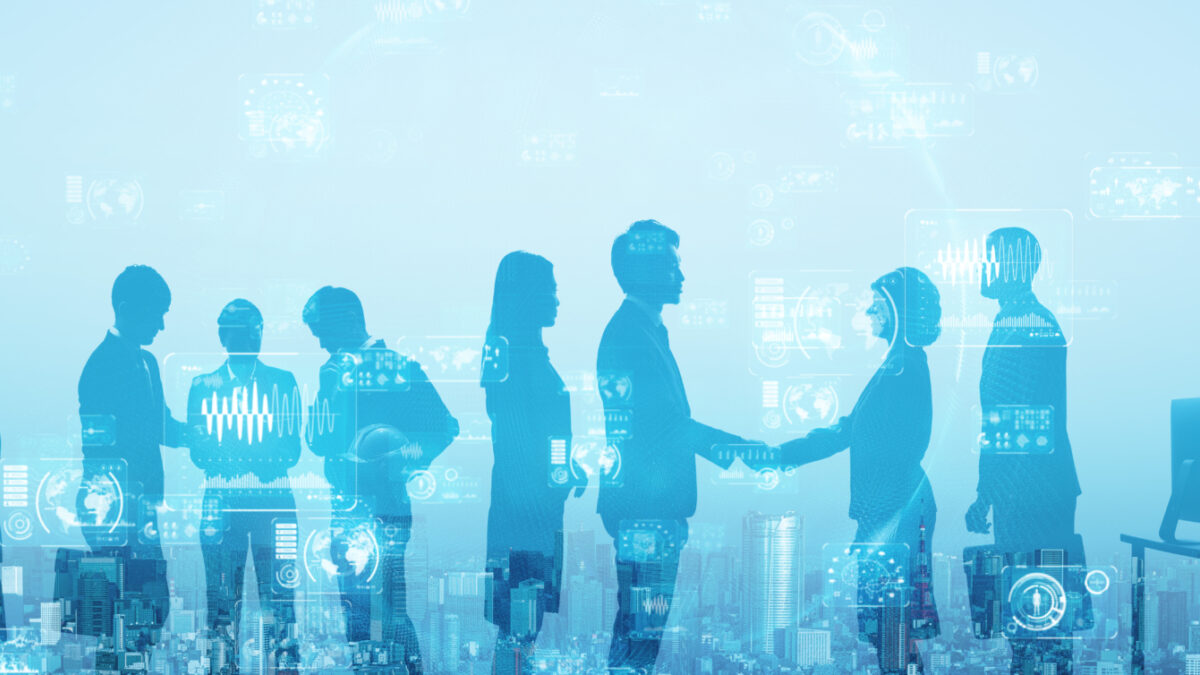
Read below for a roundup of interesting sites, resources, and articles from around the web, curated and contextualized by unbiased analytics experts at IIA. Highlights include articles about 20 questions to ask to self-analyze your data analytics strategy, how to unlock a better ROI on your AI investments, and a case study on how Mastercard quickly developed one of the best AI capabilities of any legacy company. There is also an entertaining article on how analytics were used to determine that this year’s Stanley Cup Final had the best matchup in 33 years. Follow us on Twitter and LinkedIn to receive daily updates on IIA content and curated content as it becomes available.
“Article of the Week” from IIA’s Normal Distribution
Each week, IIA’s Normal Distribution email (sent to anyone that has filled out a form on our website or subscribe here) features timely and relevant third-party articles. Here are the articles highlighted in the “Article of the Week” from the March Normal Distribution emails.
Your Data Initiatives Can’t Just Be for Data Scientists (Harvard Business Review)
"Without buy-in from your company’s rank and file, even the cleverest AI-derived model will sit idle and 'data-driven decision-making' will just go around in circles. Companies need to start seeing regular people as part of their data strategy. Data teams must work with regular people every day, develop a feel for their problems and opportunities, and embrace their hopes and fears surrounding data, then focus on equipping people with the tools they need to formulate and solve their own problems."
In addition to making the case for incorporating non-technical people into your data projects, this article also gives examples of roles laymen can take during each step of a project.
20 Questions You Need To Ask About Your Data Analytics Strategy (Medium)
What are you doing to improve your business data strategy today? To help answer this question, a data consultant team has put together a 20-question data strategy assessment that will help highlight where your team is doing well and where it can improve on its data strategy.
Becoming an ‘AI Powerhouse’ Means Going All In (MIT Sloan Review)
"There are plenty of organizations that are dabbling with AI, but relatively few have decided to go all in on the technology. One that is decidedly on that path is Mastercard. Employing a combination of acquisitions and internal capabilities, Mastercard has the clear objective of becoming an AI powerhouse." This article explores how Mastercard was able to elevate their AI capabilities and breaks down how they follow through on their 5 pillars of AI efforts:
- Powering products and services using AI
- Powering internal business operations using AI
- Supporting customers in their own AI journeys
- Pursing AI for good
- Prioritizing ethical AI
Why Hiring More Data Scientists Won’t Unlock the ROI of Your AI (InformationWeek)
It's common to focus too much on building up models and data science teams rather than operationalizing the models to drive the bottom line. This article walks you through striking the right balance between the two. The article explores the three main areas that hold back #AI scalability:
- The inability to hire #ML engineers fast enough
- The lack of a repeatable, scalable process for deploying models no matter where or how a model was built
- Putting too much emphasis on building models instead of monitoring and improving performance
A Better Way to Put Your Data to Work (Harvard Business Review)
We know that most companies struggle to capture the potential of their data. Often, they launch massive, overly ambitious programs that try to meet the needs of every end user or have individual application-development teams create singular use products and pipelines. This article instead suggests that companies adopt a data product approach which can deliver the ideal combination of creating near-term value and lay the foundation for future data use. The author explains, "when a company develops a product, it tries to maximize sales by addressing the needs of as many kinds of customers as possible with it, often by creating a standard offering that can be tailored to for different users."
After explaining the data product model and its various applications, the article walks through three aspects of adopting and maintaining a data product model:
- How to start adoption
- Managing and developing data products
- Tracking performance and quality
Featured Articles on Analytics Strategy
A Framework for Embedding Decision Intelligence into your Organization (Towards Data Science)
Deciding to be data-driven isn't enough anymore - it's too vague. Businesses ultimately need to make good decisions, not just have good data. This article discusses the topic of decision intelligence (DI) and walks through a framework of multiple decision-based exercises to complete at each step of the decision-making process in order to make more consistent, valuable business decisions.
10 Takeaways From the Harvard Business Review on Data Science (Medium)
Companies have long accepted the idea that they need to compete on an #analytics basis to gain leverage over competitors. Yet so many companies are still unable to take advantage of their #data analytics teams or aren't seeing a large enough ROI. To ameliorate this issue, the author of this article has compiled a list of their top 10 takeaways from Harvard Business Review on Strategic Analytics. Give the list a look over and see if anything's missing from your analytics approach.
4 Powerful Rules for Better Data Science (Towards Data Science)
Often, we get stuck in a work cycle that we know isn't as effective as it could be, but we also don't want to take the extra time to self-analyze and figure out exactly what we could improve upon. Luckily, #datascientist, Adam Sroka, has put together this short list of 4 rules that he found through his own self-reflection that have made him a demonstrably more effective worker.
Featured Articles on Analytics Leadership and Talent
5 Tips That Will Help You Become a Data Science Manager (Towards Data Science)
In this article, IIA blog contributor Tessa Xie gives her 5 tips to help you climb the corporate ladder and become a Data Science Manager. She outlines how to:
- Take initiative in your projects
- Mentor a peer
- Step out of your immediate scope
- Get involved in team-level activities
- Have open conversations with your manager
Six Things You Need to Know About Changing from Business Person to Data Scientist (Towards Data Science)
After spending 9 years in the world of sales and business strategy, Lucy Rothwell decided to jump into a data science career. This article details her unique experience through the first year in this new career and 6 realizations that have made her a better data scientist.
AI Reskilling: A Solution to the Worker Crisis (Venture Beat)
The world economic forum estimates that, by 2025, 97 million new jobs may emerge as a result of AI development, and recent McKinsey survey revealed that AI technologies could deliver $1 trillion of additional business value every year. But we are far from that potential right now. Businesses first need to reskill employees on basic AI technologies and adopt AI tools for simple business functions. This article is a great introduction to just that. In it you can find how to build out robust data literacy and AI learning pathways as efficiently as possible.
Stop Making the Business Case for Diversity (Harvard Business Review)
"Eighty percent of Fortune 500 companies explain their interest in diversity by making some form of a business case: justifying diversity in the workplace on the grounds that it benefits companies’ bottom line. And yet, in a recent study, the authors found that this approach actually makes underrepresented job candidates a lot less interested in working with an organization. This is because rhetoric that makes the business case for diversity sends a subtle yet impactful signal that organizations view employees from underrepresented groups as a means to an end, ultimately undermining DEI efforts before employers have even had the chance to interact with potential employees."
The authors of this HBR article go on to discuss their findings that suggest the best employment results come about from not justifying their commitment to equality at all - neither on moral nor statistical grounds. After all, businesses don't usually need to justify their other values like innovation and integrity.
AI Can Hurt Worker Morale When Managers Don’t Have These Specific Skills (Fast Company)
Many companies are looking into augmenting work feedback by implementing AI systems that can provide highly-structured and insightful feedback. However, Fast Company's research shows that while AI can beat human managers in generating high-quality structured feedback, human managers handily trump AI when it comes to gaining employee trust and buy-in. So, what's the ideal balance? This article discusses how companies can take advantage of AI feedback without hurting their workforce.
Featured Articles on Analytics Uses and Case Studies
How AI Helps Coca-Cola boost Supply Chain Procurement (VentureBeat)
"According to Brett Fultz, director of global analytics, global procurement and supply chain at Coca-Cola, artificial intelligence (AI) and machine learning tools have become indispensable in order to boost procurement and sourcing efforts at the Atlanta-based global beverage leader." This article discusses how Fultz' group uses #AI to control over $25 billion in spend across 50 logistics procurement areas
Featured Articles on AI
AI on the Front Lines (MIT Sloan Review)
"End users often resist adopting AI tools to guide decision-making because they see few benefits for themselves, and the new tools may even require additional work and result in a loss of autonomy." This MIT article details the woes of typical end user-AI conflict and explains 3 roots of end user resistance and 3 ways to encourage front-line adoption.
The Promise of AI Maturity - Theory vs. Practice (Inside Big Data)
Is there a discrepancy between how enterprise companies view their #AI maturity and how their companies rank on a maturity assessment? This article compares self-reported survey results from large enterprises to their ranking on enterprise AI maturity assessments and found that while many enterprises are investing massive amounts of capital into their AI capabilities, 60% of companies are still characterized as experimenters with AI.
The AI Ethics Boom: 150 Ethical AI Startups and Industry Trends (Open Data Science)
The demand for ethical AI services (including terms like “explainable AI” or “responsible AI”) has skyrocketed, in part due to some of the troubling practices employed by large technology companies. Everyday media is full of news of privacy breaches, algorithmic biases, and AI oversights. This article breaks down 5 key subcategories of ethical AI and explains the current and upcoming trends in the areas.
Why AI Customer Journeys Need More Friction (Harvard Business Review)
"Friction isn’t always a bad thing, especially when companies are looking for responsible ways to use AI. The trick is learning to differentiate good friction from bad, and to understand when and where adding good friction to your customer journey can give customers the agency and autonomy to improve choice, rather than automating the humans out of decision-making."
This HBR article covers 3 things companies should do during AI rollout
- When it comes to AI deployment, practice acts of inconvenience
- Experiment (and fail) a lot to prevent auto-pilot applications of machine learning
- Be on the lookout for "dark patterns"
Machine Learning Product Management (Medium)
Continuing the recent trend of applying product thinking to analytics projects, this article explores how to apply product thinking to #machinelearning projects and covers:
- The difference between a project and a product
- Why machine learning should be considered a product
- The Software Product Lifecycle
- The Investment Horizon
- The importance of building a machine learning team to support a product
If you would like to learn more about why you should adopt product thinking or how to do so, you can check out Tom Davenport's two articles on the topics - Why Adopt a Product Orientation for Analytics and AI? and Developing a Product Orientation for Analytics and AI: Assumptions and Capabilities
Entertaining Articles Featuring Analytics
We've Been Waiting 33 Years For A Stanley Cup Matchup This Good (FiveThirtyEight)
This is an interesting article on how analysts determined that this year’s Stanley Cup Final was the best matchup in 33 years.
About IIA
IIA is the industry’s leading source of insights and advisory services for companies transitioning to data-driven decision-making and advanced analytics. IIA continuously seeks out insights, information and experts to elevate our client’s and our community’s analytics expertise through two service lines. IIA's Research and Advisory Network (RAN) provides clients with access to the world's largest analytics-focused expert network; a resource designed to accelerate analytics teams' progress against their projects and initiatives. IIA’s Analytics Leadership Consortium is a closed network of analytics executives from diverse industries who meet to share and discuss best practices, as well as discover and develop analytics innovation, all for the purpose of improving the business impact of analytics at their firms. IIA’s family of analytics assessments provide actionable, diagnostic insights for organizations looking to maximize their analytics performance.