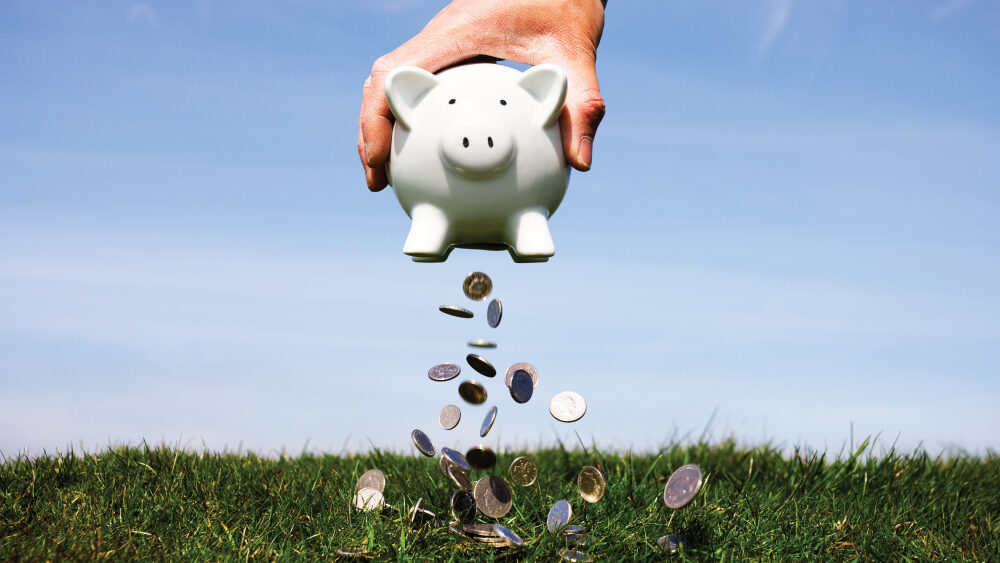
I’ve just finished interviewing a large group of chief data (and analytics in most cases) officers for a report I’ll have more to say about in a month or so. But one result really struck me, and it has a lot to do with analytics and AI.
The Perils of CDOs with no A
Chief data officers—those who don’t have responsibility for analytics, and are hence unlikely to be browsing the IIA website and reading this post—are noted for two things. They have high expectations in their jobs and short tenures in them. Why do they not stay in their jobs for long? I would argue that it is very difficult to provide visible value to a company from data management alone. A company’s data will never be perfect, and improvements in it are usually something of an abstraction that many businesspeople may not understand or appreciate. As one of the CDAOs I interviewed put it, “A CDO role without analytics is a two-year job. Maybe there are some pressing problems with data. Even if you fix them, it’s not enough. The company is looking for someone else in a couple of years.”
A former CDAO who has held a variety of those jobs and has just left one (voluntarily) said, “I get all these calls from headhunters. If they say they are trying to fill a CDO job without analytics, I tell them to look elsewhere. I view the role as primarily about business transformation, and you can’t do that without analytics and AI.” I see this as the primary reason why an increasing number of jobs are CDAOs, not just CDOs.
Perhaps I am preaching to the converted among IIA sponsors and followers on this topic. You probably already appreciate the value of analytics and AI in bringing measurable value to organizations. But there are other subtleties I noticed as I was doing these interviews.
Generating Value at Low Maturity Levels
One involves how best to generate value from analytics and AI, and it depends upon the maturity level of the organization with regard to those capabilities. Let’s say you are a CDAO and you have just joined an organization that is at level 2 or 3 in the IIA analytics maturity model, which originated in a book I co-authored called Analytics at Work (hurry, only one hardcover left in stock!). You need to quickly establish that analytics and/or AI applications can quickly move the needle on the company’s performance. You have no time to build infrastructure or—God forbid—to embark upon a master data management project. You need to get some important use cases underway ASAP.
One CDAO at a pharmaceutical company told me that’s the approach he took when he arrived at his company as CDAO. I make no judgment on its maturity level, but he deduced that he needed to quickly figure out a few important analytics projects that would demonstrate how valuable analytics (and he) were to the company. He interviewed a number of senior executives, found some who bought into sponsorship of key projects, and embarked upon three of them. This CDAO has been in the job for five years or so—double the average CDO job tenure—so he must be doing something right. And his pursuit of these key projects has provided some air cover for something he feels is very important but difficult to measure and value—changes in the company’s culture relative to data and analytics. He established a new group to build literacy and move the company toward a data-driven culture.
But what do you do if the data at your company aren’t up to the task of fueling those use cases? Again, this CDAO had the right approach. Most of his data management efforts were undertaken with regard to making possible those three prominent use cases. That way the organization can see and understand the value of better data initiatives, and eventually, have some economic returns to balance against their cost.
Generating Value for the Very Mature
On the other hand, if you check into a new CDAO job at a level 4 or 5 company, a different strategy for generating value is in order. Your new company knows the value of analytics and AI, and probably already has a number of successful use cases up and running. You need to accelerate that process by building data and analytics infrastructure. Even at this level of maturity, I’m still not a fan of master data management projects; they take too long and often fail. However, mature organizations can compile a set of reusable datasets that can power a variety of different analytics and AI projects.
You may have also heard about companies that are building feature stores (I guess if they are for traditional analytics we would call them “variable stores,” although it has never made sense to me that they are variables in analytics and features in machine learning). These ready-made predictive factors for inclusion in machine learning models are also very beneficial in speeding up model-building. They probably wouldn’t be appreciated by low-maturity organizations, but are a must for those at the high end of analytics and AI capabilities.
I recently spoke, for example, with the CDAO of a large telecommunications company. They were about as far along as anyone I have seen in terms of their data and analytics infrastructure. They have thousands of reusable datasets, feature stores chock-full of good predictors, and APIs that can call pre-defined machine learning scores when needed. If you need a quick “proclivity to churn” score for a customer, you can just call it with an API. All great stuff, but you wouldn’t want to build it at a company that is just getting started with analytics and AI.
So thank your lucky stars that you are involved in IIA and not some data management organization. You are possessed of the best means to achieve visible and measurable value to your organization. Just make sure that you choose the right approach to generating value with analytics and AI based on your maturity level, and you’ll probably be a CDAO for decades!