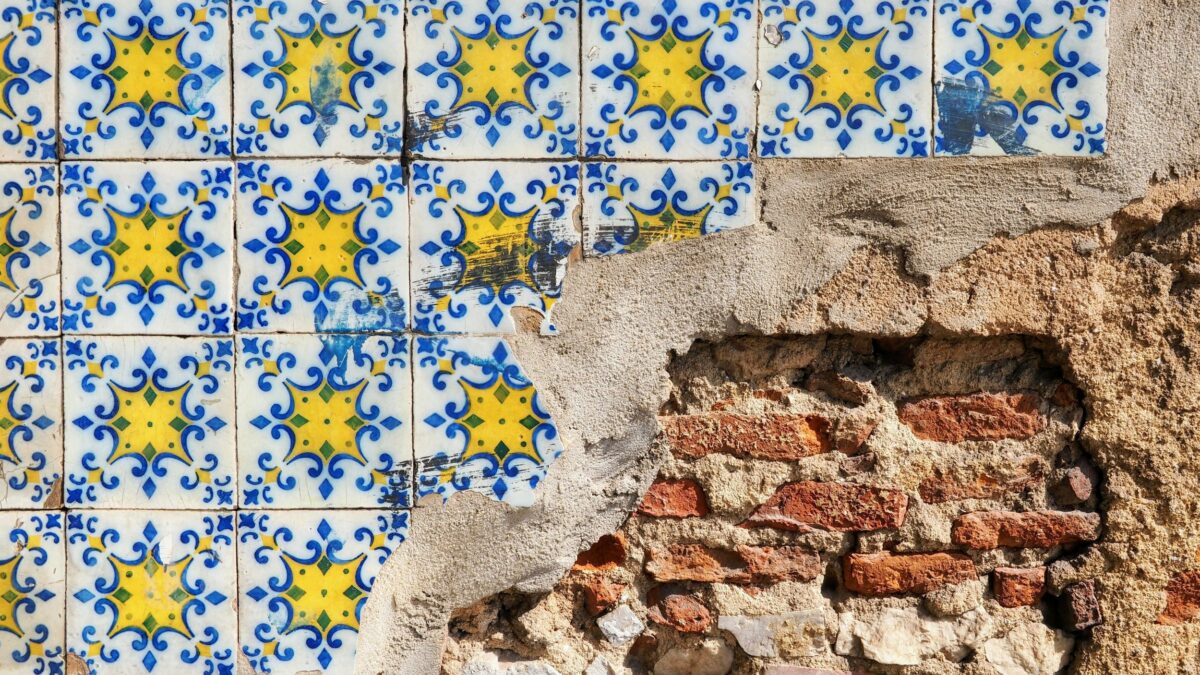
The vast majority of people building analytics and data science processes have every intention of being good and ethical. As a result, most potentially unethical and evil processes arise in situations where that wasn’t the intention. The problem is typically that proper focus and governance is not in place to keep analytics and data science processes on the side of good. On top of that, what is good and what is evil isn’t nearly as clear cut as we’d wish it to be.
There Are No Absolutes with Ethics in General
Ideally, what is good and ethical would be clear. Unfortunately, things are rarely totally clear in the real world. Let’s start by considering an ethical guideline that has likely been accepted by every culture over human history. Namely, the guideline that we should not kill another person.
It is almost unanimous that killing people is evil and unethical. However, nearly everyone will hedge their commitment to not killing others in multiple ways.
Is it ok to kill someone in self-defense if you are about to be killed by them?
Most, but not all, people will say yes.
Is it ok for a soldier to kill an enemy soldier on a battlefield during a war?
Most, but not all, people will say yes.
Is it ok to execute someone convicted of killing others?
Many people say yes and many say no.
We could come up with hundreds of scenarios. The point is that while people will almost universally affirm that a policy of not killing others is ethical and good, people will also quickly acknowledge various exceptions. Once the door to exceptions is opened, then it can be expected that more will be found. While not killing others is the ideal ethical policy, in reality few agree with following that policy to the letter.
There Are No Absolutes with Ethics in Analytics and Data Science
Even as we develop policies to help keep our data science and analytics processes good and ethical, we will find situations requiring an exception. Let’s consider the example of a customer who asked to be put on an organization’s “do not contact” list. Are there cases where the organization could ethically still use the contact data to contact the person in spite of the do not contact request?
What if the company uncovers a potential risk the customer faces using its product?
Most people will agree it is ok to contact and alert the customer.
What if the customer’s family member has a medical emergency at a company location?
Most people will say it is ok to contact the customer.
Is it perhaps the good and ethical choice to contact the customer in violation of the do not contact request and the unethical choice to leave them in the dark about the emergency?
What if a security breach compromised the customer’s data?
Most people will agree it is ok to contact the customer. And, as above, might it be more ethical to violate the do not contact instruction than to honor it?
These examples illustrate that what is good and ethical is much blurrier than it seems. After all, a do not contact flag seems black and white. However, many exceptions can be found. Such ambiguities make it difficult to stay on a good and ethical path.
Ensuring Ethical Data Science
I’ve previously discussed the need for intentionality to guide an organization to ethical policies surrounding data science and analytics. Clear policies that take a firm stand are also required.
A few years ago, Apple took a firm stand and refused to create a tool that would allow the United States government to unlock a terrorist’s phone. The stance was based on consideration of both the immediate request, but also the long-term implications of creating an unlock capability. The point here isn’t to judge the position. The point is that it is good that Apple took a clear position. Regardless of whether we agree or disagree with Apple, we all know where they stand.
Whatever ethical lines your organization wants to draw around analytics and data science, it is better to have the lines clearly drawn and transparent than to leave employees and customers guessing. Plus, the more lines that are clarified up front, the less often that those in the data science trenches will be on their own to make the right decision due to the lack of guidance.
The end goal should be a clear set of ethical guidelines for the data science team and an ongoing effort to identify any exceptions, special cases, or totally new scenarios that must be addressed. Throughout the process, organizations must be prepared for the fact ethical positions will always be fraught with gray areas and exceptions, which will make it impossible to satisfy every viewpoint in every case. Those challenges can’t preclude the pursuit of clear guidelines that will keep data science and analytics practitioners and processes in the realm of the ethical and good.
Originally published by the International Institute for Analytics
Bill Franks, Chief Analytics Officer, helps drive IIA's strategy and thought leadership, as well as heading up IIA's advisory services. IIA's advisory services help clients navigate common challenges that analytics organizations face throughout each annual cycle. Bill is also the author of Taming The Big Data Tidal Wave and The Analytics Revolution. His work has spanned clients in a variety of industries for companies ranging in size from Fortune 100 companies to small non-profit organizations. You can learn more at http://www.bill-franks.com.
You can view more posts by Bill here.