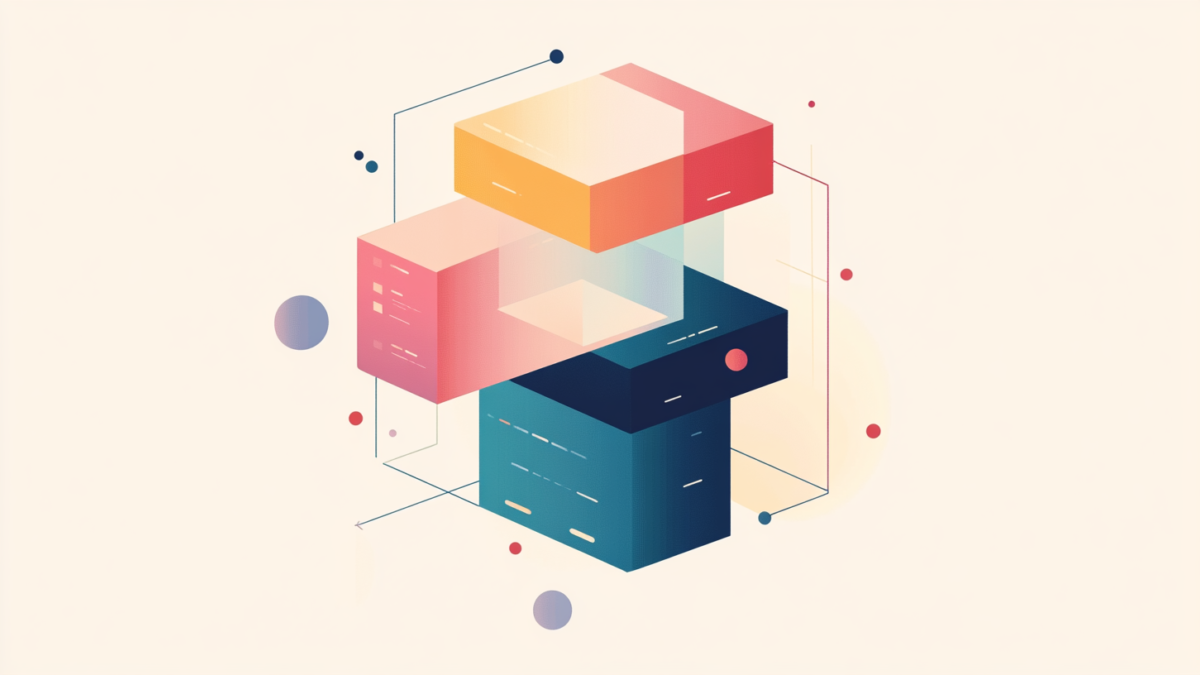
I have been reading a lot of different literature on this topic, and something has made me wonder: The terms data strategy, data governance, and data management are not used consistently, even sometimes used interchangeably. Understanding the distinct roles and relationships between these is I think crucial for organizations aiming to leverage data effectively. The role of data governance in this is particularly interesting.
On the one side, data governance is described as “what makes strategy tangible.” On the other side, especially through concepts like data mesh and computational governance, the lines between data governance and data management are blurred.
Maybe the overlap between these three is not the problem itself, but the structural focus that aligns these concepts? The lines between data strategy, data governance, and data management are not clear, yet the intent, mission and interplay can be. So distinguishing data governance as a tactical decision layer can emphasize its importance in translating strategic intent into actionable operations, while also create understanding of the concept of oversight for data management actions.
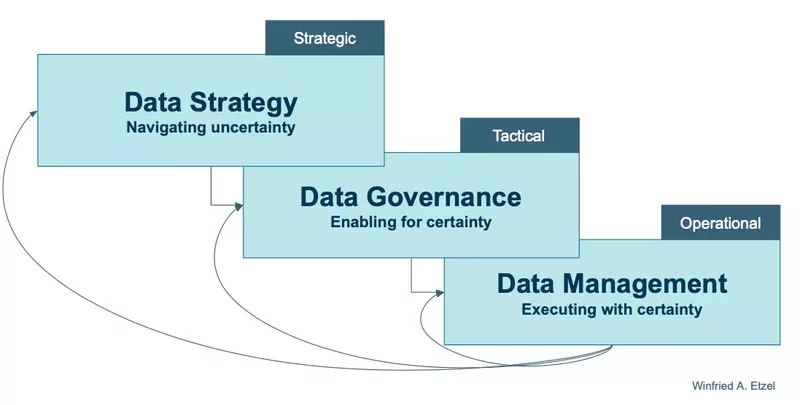
The Strategic Level: Data Strategy
Data strategy operates at the strategic layer of an organization. It involves designing a logical way through uncertainty, guiding the organization toward its goals despite unpredictable conditions. As Roger Martin put it, “Strategy is not a plan.” This is important to emphasize, because planning is done with resources, capital, market conditions, and so on—factors that can be predicted with a level of certainty. Instead, it is a logic that outlines how an organization aims to achieve its objectives within an uncertain environment.
Data strategies tend to become outdated or unused. This often results from outlines that are too theoretical. Yet, that is what a strategy should be. To make it tangible, you need to follow up with an operating model that translates strategy into action.
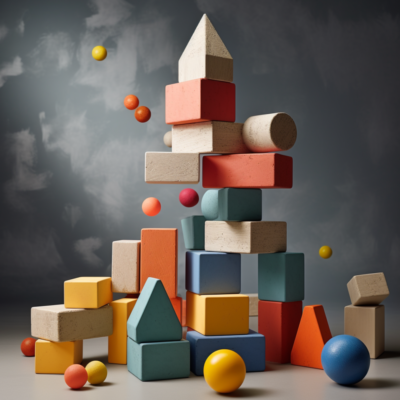
Creating a Data Strategy eBook
The growth in the demand for better decision-making with data means that a comprehensive data strategy is no longer a nice to have. You need a data strategy. It needs to address questions about how to improve the availability, timeliness and quality of data, in that order of priority.
The Operational Level: Data Management
Data management exists at the operational layer, focusing on the execution of daily tasks to ensure data is used efficiently, effectively, reliably, and at scale. It encompasses a wide range of practices and knowledge areas, making it inherently cross-functional. In other words, there are many touchpoints within data management at the operational level that are not illustrated in the graphic above.
This layer is all about the practicalities of handling data—from data quality management to data integration and security—ensuring that the data is ready and available for use in decision-making processes.
And it is also at this layer where operational models take effect.
The Tactical Level: Data Governance
Data governance functions at the tactical layer, between data strategy and data management. It translates strategic business direction into specific actions by aligning people, processes, and technology with accountability, value proposition, and strategic logic.
At the operational level, data governance oversees and guides data-related decisions to ensure alignment with the organization’s strategic goals. Although it is technically a part of data management, its role is unique in that it serves as the bridge between the strategic vision and operational execution.
In Data Management Association (DAMA) language, data governance is placed at the center of the data management wheel, a notion that still stands, yet in a three-dimensional model, it will be at the center, but elevated.
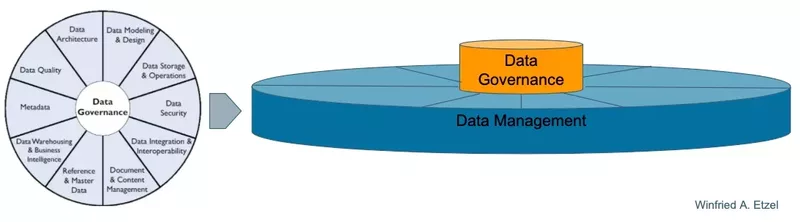
Data governance is where strategy becomes tangible. You can call it the mechanism through which an organization’s data practices are aligned with its business objectives. The Data governance function can vary significantly in approach, ranging from enforcement and compliance-driven methods to enablement and business-value driven. Regardless of the approach, the “how” of data governance should always be aligned with the “why” and contribute to clear the way for the strategy to come in effect.
The Overlap
While data governance and data management are closely intertwined, it’s essential to differentiate between them to provide needed oversight. Data strategy feeds into data governance and, in turn, data governance structures data management practices. This interplay ensures that the data organization is guided according to strategic intent. Ideally this creates a cohesive data ecosystem across all layers.
The Feedback Loop
A cohesive data ecosystem across all layers incorporates a set of robust feedback loops. Internally, within data management tasks and deliveries, the plan-deploy-monitor-act cycle, commonly used in data quality management, can be adapted for all data management practices.
Results and learnings from decisions made at the operational layer need to feed into the tactical layer to adjust tactical decisions and refocus oversight. This feedback allows for refining and adjustment.
In addition, there is the concept of a “double feedback loop,” which aligns closely with Henry Mintzberg’s idea of emergent strategy. It suggests that strategy should not be static but should evolve based on learning and changing circumstances. These changes should be communicated to the strategic layer through a feedback loop, enabling adjustments to the strategic logic based on these insights.
Heinz von Förster and Niklas Lehmann coined the term Second-order Observation—it’s about learning how to observe observers, that has later been developed into the double-loop learning model. Think of first-order observations as best practices; second-order observations, then, are about questioning if the best practices at play are effectively contributing to the anticipated goal or are in line with underlying values.
Conclusion
Viewing data governance as a tactical decision layer can help us understand the interplay between data strategy and data management. It ensures that strategic goals are translated into actionable operations, aligning data practices with business objectives. I think that by clearly defining and differentiating these concepts, organizations can utilize their data practices more efficiently and effectively. I would also recommend having a clear notion of how to utilize the principles of feedback and adaptive learning. What is interesting is that through this perspective, data governance enables organizations to remain agile and responsive to evolving challenges and opportunities.
Originally published on Medium.