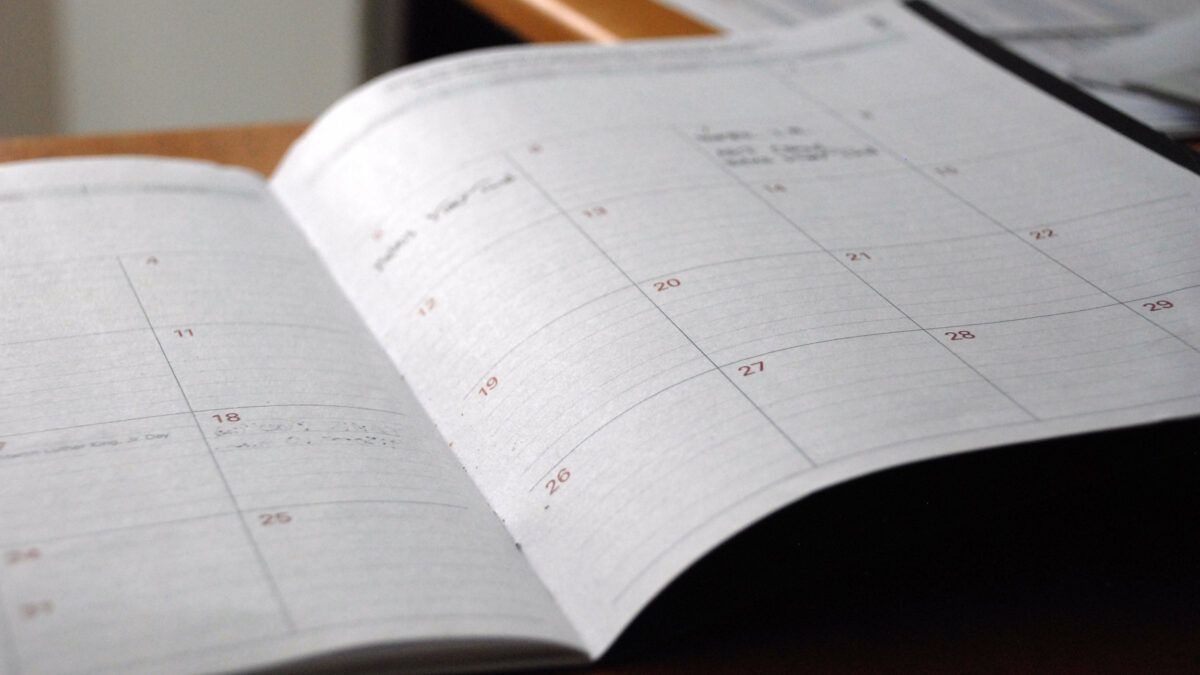
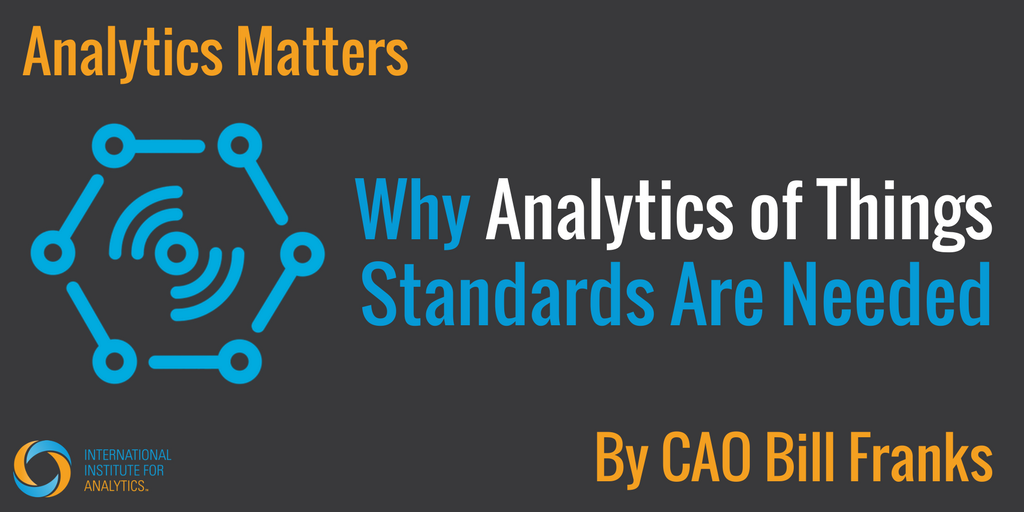
As the Internet of Things (IoT) continues to explode, so does the need for the analysis of IoT data. At IIA, we call the analysis of IoT data the Analytics of Things (AoT). There are many success stories from the world of AoT. However, without some attention to standards of both IoT data itself and the analysis of it, organizations will struggle to achieve AoT’s potential. In this post, we’ll dig into several different areas where standardization must be pursued.
IOT COMMUNICATIONS STANDARDS
Many devices need to communicate with one another. Sometimes, this communication is a simple exchange of sensor readings, while in other cases it is the passing of complex analytics results. Without standards around how these communications occur, issues will arise. Some scenarios are fine with localized standards, while others require global standards.
To illustrate a local standard, consider a smart kitchen. That kitchen might have a wide range of appliances that all communicate to help keep everything running smoothly. As long as each brand of kitchen appliance has a standardized protocol, my kitchen works perfectly as long as I stick to a single brand. The fact that my neighbor has a different brand with a totally different set of standards doesn’t matter since our kitchens have no need to communicate with each other. In other words, localized standards are fine.
Global standards will be necessary for situations like autonomous vehicles. Having all the cars from one manufacturer able to communicate together, but unable to talk to other brands, would be a disaster. To maximize traffic flow and efficiency, cars will eventually need to communicate both with each other and with a traffic management system so that optimal routes can be determined, cars can allow others to merge, etc. The only way to make this work is to develop global standards for communication that are followed by all car manufacturers.
ANALYTIC DATA STANDARDS
There are reasonably well-established protocols for how raw sensor data is generated. To ingest raw sensor data is not difficult. However, the raw data requires a lot of manipulation in order to get it ready for analytic processes and there are numerous challenges when attempting to analyze IoT data. As these challenges are addressed, it is important for an organization to develop standards that are followed moving forward.
By documenting the right cadence, aggregation level, and other details required to enable the AoT analytics an organization must do, it will make it much easier to expand and scale. For example, adding additional predictive maintenance processes is far easier if standard metric definitions and data formats are established. This is no different than any other type of data. But since IoT data is new, it is common that multiple teams in an organization have been experimenting independently and diverging in approach. It is important to align those approaches once the analytics are proven effective.
AOT ANALYSIS STANDARDS
Another type of standardization that will help organizations scale AoT capabilities is to standardize analytic approaches; this means agreeing what algorithms and toolsets will be used for what problems. It also means agreeing to the output structures that the models will populate. In other words, the output from predictive maintenance models should have a predictable (pun intended) and consistent set of information. Regardless of what maintenance issue is being predicted for which equipment, key information includes an equipment identifier, an issue identifier, and a probability of a problem, among other factors.
By standardizing the output structures, it is far easier to feed predictions into downstream applications. Interface developers will have clear protocols for accessing and making use of AoT model outputs. That will make it easier and cheaper to build AoT results into business processes, which is the end goal.
CONCLUSION
The prior points really come back to classic best practices for analytics, but it is always worth remembering and applying those old lessons in new contexts. Whenever there is a new data source or a new type of analytics, it is important to recognize when the value has been proven enough that there will be a need for much more of the same in the future. At that point, the standardization effort must be made. The longer standardization is put off, and the more processes built as one-offs, the more painful it will be to implement and retrofit standards later. While these challenges aren’t unique to the Analytics of Things, the AoT is at the point that it is time to address them.