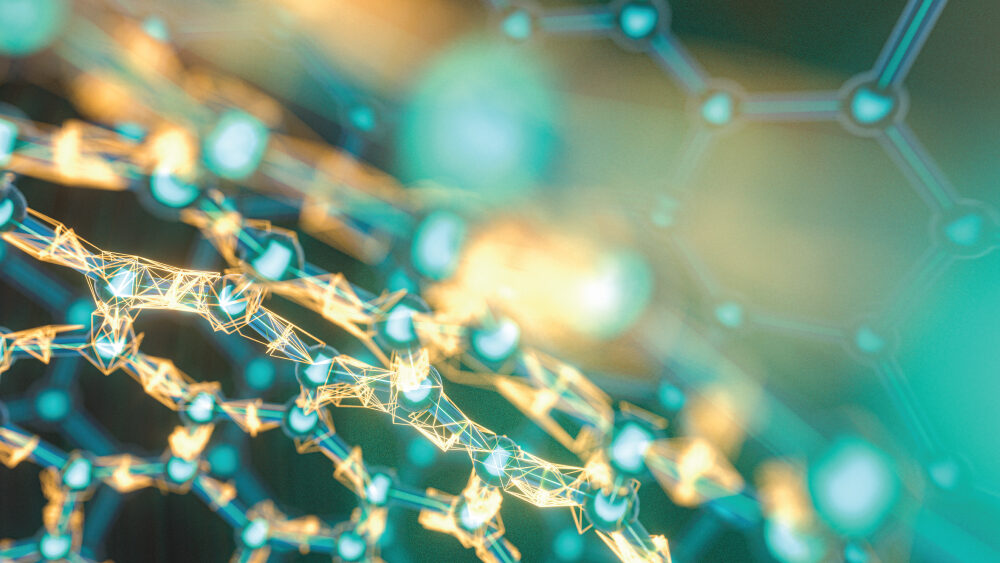
Synthetic data, as the name suggests, isn’t real. Instead, it is artificially generated. When first hearing the term “synthetic data,” it is easy to dismiss it as an unserious concept that couldn’t be useful in practice. However, synthetically generated data doesn’t mean randomly generated data. On the contrary, synthetic data is carefully engineered to mimic the real data it is based upon. In fact, today’s synthetic data capabilities have progressed to a point where such data has become a critical component of many real-world data science solutions. This was a focal point in IIA’s 2023 Predictions and Priorities webinar and associated research brief. I’ll dig deeper into the concept here.
Synthetic Traditional Data
Synthetic data technologies applied to traditional structured data sources like transactional data are carefully designed to maintain the statistical properties of the real set of data that is used as the basis for the synthetic data. After designing the process, new records are generated that, while not real, will mimic the real data across the dimensions that were specified as relevant for a given analysis. This means that analysis of the synthetic data focused on those dimensions will be useful to understand the dynamics of the real-world data on which it was based. Among other benefits, this enables small sample sizes to be expanded much larger so that analytic algorithms can more readily detect patterns in the data.
This statistical approach to synthesizing data is also invaluable when highly sensitive data, such as medical or financial records, are to be analyzed. By using synthetic data, valid patterns can be found while still protecting the privacy of those whose data was used to generate the synthetic data. If sensitive data is a large part of your organization’s focus, then synthetic data should be front and center in your data and analytics strategy. By helping avoid potential legal, ethical, and consumer perception issues, synthetic data can enable the analytical breakthroughs you need while avoiding some major risks.
Synthetic Non-Traditional Data
Another type of synthetic data arises from generative AI technologies. Facial recognition algorithms are notorious for inaccuracy and bias. Different skin tones, different angles, and different lighting can all impact the accuracy of a facial recognition process. Worse, if a database of faces is notably short of images of certain skin tones or other features, the algorithms won’t work as well for those skin tones or features. Synthetic data can come to the rescue by creating a sufficient sample of images (or text or audio or any other type of data) for each subpopulation that needs a boost in accuracy and a decrease in bias.
It would be prohibitively expensive and very time consuming to get 10,000 pictures of any given person with every combination of 10 angles, 10 skin tones, 10 lighting levels, and 10 hairstyles. A synthetic version of those same 10,000 images can be generated quickly and inexpensively. While those 10,000 images will be synthetic, today’s generative AI capabilities are able to craft images realistic enough that they are able to train a facial recognition model to recognize real images more effectively. It is a bit mind-bending to accept the fact that fake data can help real models do better with real data, but it is true!
Other Synthetic Data Approaches
There are other situations that make use of variations on synthetic data as well. The extensive work happening in the area of digital twins involves simulating a real-world object or environment and seeing how various scenarios impact it. This is extremely common in manufacturing, for instance. While a simulated manufacturing environment isn’t real, if it is designed well enough, the results of experiments in the simulated environment will provide insights useful about the real world. This is conceptually similar to how scientists make a lot of physics breakthroughs by simulating certain situations and “proving” a theory could hold before doing expensive real-world tests that formally prove the theory.
In other situations, data that was created for one purpose is useful for another when viewed as synthetic data. For example, autonomous vehicle systems have used games like Grand Theft Auto for years now to train models. While the roads rendered in such video games aren’t 100% realistic, they are realistic enough to help train an autonomous vehicle system on some of the basics of staying on a road, avoiding obstacles, and such. Certainly, it is much preferred to have early versions of autonomous vehicle models learning on fake roads in a video game where mistakes don’t matter than learning on real roads where they do.
Are You Using Synthetic Data Yet?
Synthetic data is going to become ubiquitous in the coming years. The way it can protect privacy and negate the need to use identifiable information in building models just isn’t available elsewhere. Also, by synthesizing data with precisely known distributions, tags, or attributes, data scientists can more accurately gauge how well models are performing. If your organization hasn’t yet started to utilize synthetic data, then make a New Year’s resolution to change that in 2023. There is an entire ecosystem of companies now addressing different aspects of the synthetic data space that are available to support your efforts. Synthetic data can save cost, protect privacy, and increase the speed of development all while making models more accurate and less biased. That’s a hard combination to beat and it is why synthetic data is a next big thing!