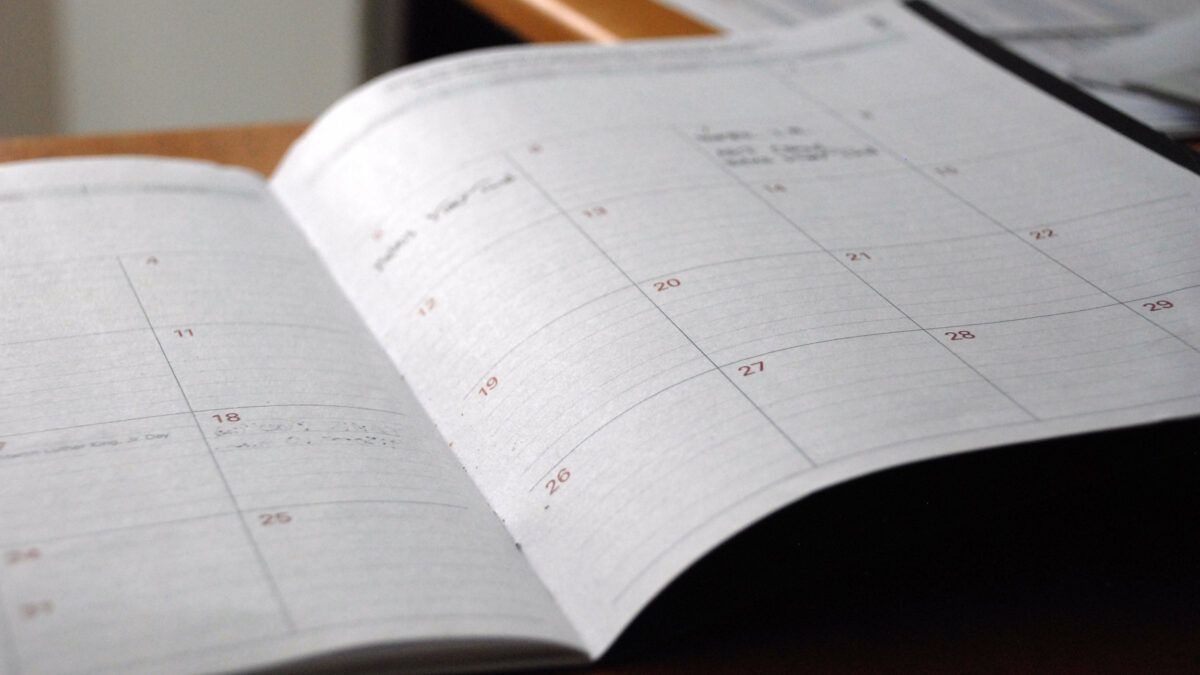
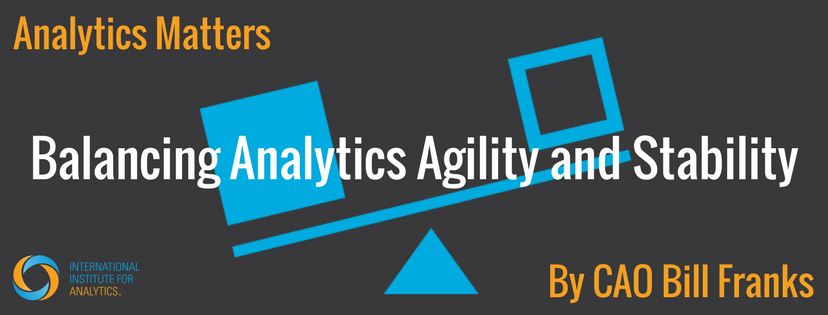
There have been many science fiction stories (as well as video games!) that revolve around the tradeoffs between powerful, strong, hard to harm combatants and those that are small, nimble, but easy to harm. Both have their merits and both can be useful in different situations. However, the same profile doesn’t work best in every situation.
There are situations in a fight where being fast is more important than being strong. There are also cases where being able to stand your ground is more important than speed. The same is true with analytics. At times, agility is more important. At other times, stability is more important. The key is to know when you need which option
THE FLAWS OF TARGETING ONLY STABILITY
Many organizations struggle to progress effectively in their analytics journeys because of too large a focus on stability. During the exploration and discovery process, nearly all the stringent rules applied to a mission-critical production process are enforced from the start. This makes it very difficult to go in new directions and drives costs up much higher than they need to be. After all, if I just want to quickly see if an idea has merit, I don’t need my process to be fully vetted and hardened. The time for that is later.
THE FLAWS OF TARGETING ONLY AGILITY
It is entirely possible to go too far the other way. Especially in a smaller organization, it is possible to get into a situation where there are virtually no governance processes or other controls in place. If someone has something they think works, it is deployed with little thought to ongoing support or how stable the process is. This, too, leads to a mess because invariably something will break and a mad scramble to fix it before too much damage is done will ensue.
After years of organizations leaning way too far and too early towards stability, today many analytics teams are over-reacting and leaning too far toward agility.
LEARNING A LESSON THE HARD WAY
One of my colleagues worked with an organization recently to assist them in implementing some new analytics. Historically, IT had placed very firm constraints on the client’s team and how it built processes. To compensate, the team deployed an open source data repository and loaded all of the data they used into it so that they would have full control of their own destiny. Up to that point, there is no problem. The problem arose when the team started deploying processes out of the same platform.
The client boasted to my colleague about how many new processes they had deployed and how much faster they were deploying. He also mentioned how his latest process required a different format type for some of the data than was currently applied to the data. Luckily, he said, all he had to do was change the data type and he would be off to the races. No pesky IT team telling him he couldn’t change the data type!
My friend then asked the very simple question, “So, if you change that data type for this new process, what will happen to all of the previous processes you already built against that data which are expecting the current data type?” The client had a blank stare for a few seconds and then had the “aha” moment that he hadn’t considered. Namely, that all those processes would break. There was a need for some level of stability across processes even while there is a need for agility for his new process.
STRIKING THE BALANCE
Usually in life, and analytics, you’ll find that if there is a long-standing tradition or rule set then there likely is some reason for it. Perhaps the rules need tweaking or the tradition needs to be updated a bit. But, it is rare that they exist without some logical root reason. The same is true with strict IT process controls.
When deploying processes at an enterprise scale, stability is critically important and thus some rigidity and strict oversight is warranted. At the same time, when discovering new insights and developing new processes, agility is critical in order to validate that the new idea can succeed and to find the best way to do so.
However, it is a fatal flaw to either always enforce stability or always allow agility. There is a time for each. As the client in the above example realized, it can sound great to quickly make changes to data to fit your current needs. But, that can’t be done without considering what else is impacted.