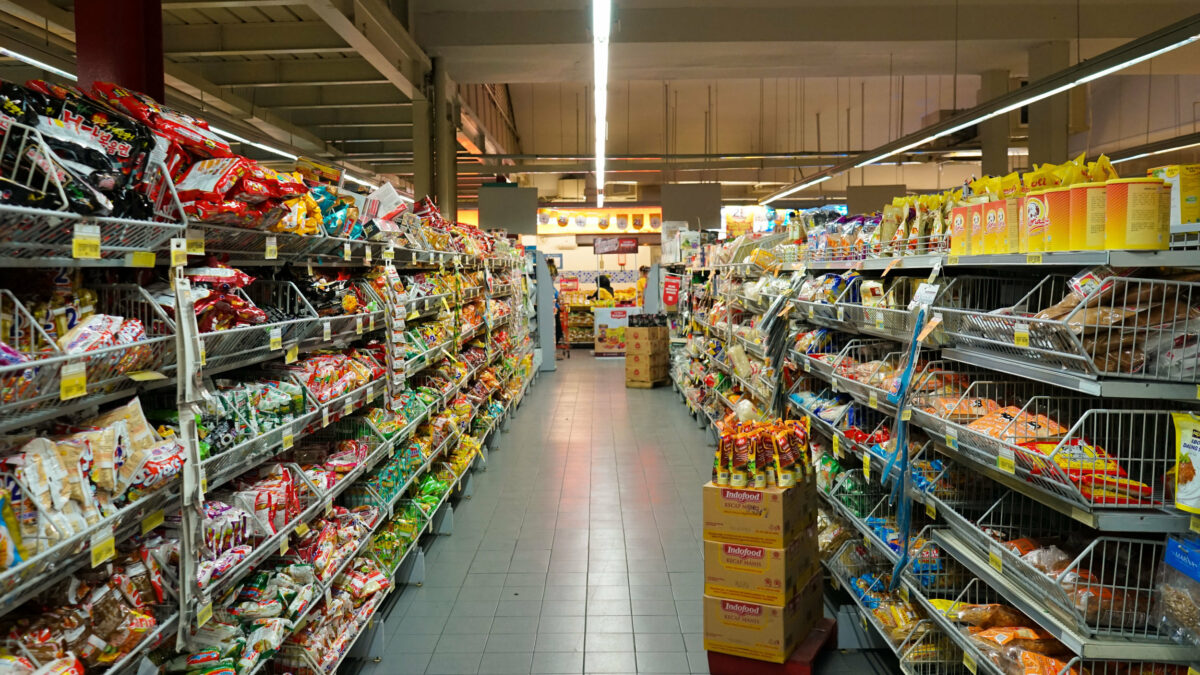
Many organizations today are wondering how to get into machine learning, and what it means for their existing analytics operation.
There are many different types of machine learning, and a variety of definitions of the term. I view machine learning as any data-driven approach to explanations, classifications, and predictions that uses automation to construct a model. The computer constructing the model “learns” during the construction process what model best fits the data. Some machine learning models continue to improve their results over time, but most don’t.
Machine learning, in other words, is a form of automating your analytics. And it has the potential to make human analysts wildly more productive.
To illustrate the movement from “artisanal analytics” to “autonomous analytics,” I’ll provide an (anonymous) detailed example. The company involved is a large, well-known technology and services vendor, with over 5 million businesses as customers, 50 major product and service categories, and hundreds of applications. Each of its customer organizations has on average four key buyers. The company needed to target sales and marketing approaches to each company and potential buyer. To do this, it created a score for each customer executive, reflecting their propensity and ability to buy the company’s offerings, so that sales and marketing approaches could be more effective.
This approach is called “propensity modeling,” and it can be done with either traditional or autonomous analytics approaches. Using traditional human-crafted modeling, the company once employed 35 offshore statisticians to generate 150 propensity models a year. Then it hired a company called Modern Analytics that specializes in autonomous analytics, or what it calls the “Model Factory.” Machine learning approaches quickly bumped the number of models up to 350 in the first year, 1500 in the second, and now to about 5000 models. The models use 5 trillion pieces of information to generate over 11 billion scores a month predicting a particular customer executive’s propensity to buy particular products or respond to particular marketing approaches. 80,000 different tactics are recommended to help persuade customers to buy. Using traditional approaches to propensity modeling to yield this level of granularity would require thousands of human analysts if it were possible at all.
There is still some human labor involved. Modern Analytics uses fewer than 2.5 full-time employees to create the models and scores. 95% of the models are produced without human intervention, but in the remaining cases people need to intervene to fix something. The technology company does have to employ several people to explain and evangelize for the models to sales and marketing people, but far fewer than the 35 statisticians it previously used.
If your company already has some analytical skills, it may be able to do machine learning models by itself. Cisco Systems, for example, went from doing tens of artisanal propensity models to tens of thousands of autonomously generated ones. A small group of analysts and data scientists in a group called Global Customer Insights generates these models each quarter.
Turning your current analytics operation into a machine-learning-savvy “model factory” requires some changes, of course. First of all, your analytics experts are going to need some new skills. Instead of painstakingly identifying variables and constructing models, machine learning analysts or data scientists need to focus on assembling large volumes of data and monitoring outputs for relevance and reasonability.
They may also need to work with some new tools. Vendors of proprietary analytics software are rapidly adding machine learning capabilities, but many algorithms are available in open source formats that provide less support to users. And since machine learning models typically operate on large amounts of data and are computationally-intensive, it’s important for analysts to employ in-memory or elastic cloud (infinitely expandable) hardware environments. These technologies can handle the largest datasets and can dramatically accelerate computation speeds.
If there is already a central analytics group or center of excellence in place, it probably already has the statistical expertise in place to interpret machine learning models to some degree. But full interpretation is very difficult. When there are thousands of models in place to address a business process, it may be impossible to interpret each one. And some variations on machine learning—neural networks and their more detailed cousin, deep learning—are virtually impossible to interpret. We end up knowing which variables predict an outcome, but we don’t know why.
The lack of transparency from machine learning is one of the greatest cultural and leadership challenges to overcome with the technology. Managers must learn to trust models that they don’t fully understand. The key is to be vigilant about whether the models are actually working. If, for example, they no longer do a good job of predicting lift from a marketing program or sales from concerted sales force attention, it’s probably time to revisit them.
The world is a big and complex place, and there is increasingly data available that reflects its size and complexity. We can’t deal with it all using traditional, human-crafted analytical methods. Organizations with some familiarity with those methods, however, will have an easier time transitioning to more autonomous approaches involving machine learning. The time is now to begin such a transition.
This article was originally published in Harvard Business Review