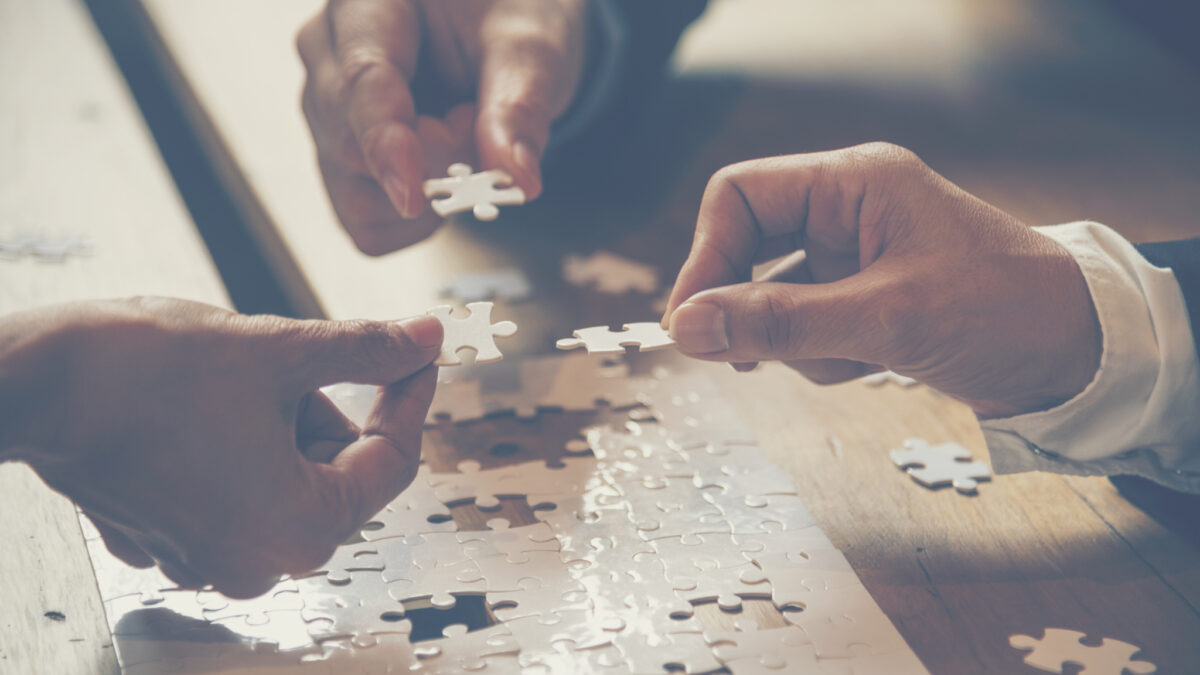
Five lessons in pragmatic data science
Background
Most analytics transformation efforts do not deliver a positive ROI for the enterprise even after several years. CEOs and their boards know they need to execute an AI-led differentiation strategy to either future-proof themselves, address an existential risk in the marketplace, or make operational improvements to their business. Yet, according to most estimates, 75–90% of digital transformations fail, and less than 1 in 5 companies have fully extracted value from their analytics journey. Most organizations fail on the last-mile delivery - in other words, front-line employees and decision-makers are not using the analytics tools and processes as intended or not using them at all.
Over the last decade of leading analytics teams (both general analytics and more specialized revenue management groups), I have succeeded and failed many times. Below are five lessons learned from these experiences. I hope it can serve as a helpful 10,000-foot roadmap for nascent or aspiring analytics leaders or seasoned business executives who want to build a sustainable practice that adds real, quantifiable value for their company and its customers.
Lesson 1: Define the Overarching Data Monetization Strategy
Analytics should be treated just like any other critical function in an organization that needs to support corporate strategy and a customer-centric vision. For companies serious about analytics, it should be the central enablement pillar of their strategic pyramid, permeating all functional areas.
I’m a massive fan of OKRs (Objectives and Key Results), which, when done right, hold the organization accountable to ambitious quarterly, semi-annual or annual goals (Objectives), with a clear roadmap of how those goals will be accomplished (Key Results). Your analytics team’s OKRs should directly support organizational OKRs and must focus on driving measurable customer outcomes — internal or external.
As an analytics leader, I recommend you align with your CXO and leadership team and choose a time-bound analytics strategy to focus on. Time-bound and dynamic, your analytics strategy must adapt to the evolving marketplace, technology, and customer expectations. I posted an excellent research article on this in a previous blog, in which researchers at MIT describe four primary data monetization strategies for organizations:
1. Operational Optimization: think process automation, pricing optimization, and, more generally, any data-driven solutions and process improvement efforts that take costs out of the system. Most companies start here, especially those from non-tech industries or with a nascent analytics acumen.
2. Customer Focus: deploying customer-facing analytics solutions. It could be augmenting core product offerings with unique insights or actioned analytics for the customer or simply delivering data science solutions that improve your customers’ lives. After a proven impact on your gross profit and EBITDA through operational optimization efforts, this is the logical next step for companies and one with the greatest medium- to long-term payoff.
3. Direct Revenues: this is about creating analytics products and charging money for them or some other value exchange between the company and its customers, suppliers, or other industry partners. It could take the form of analytics SaaS products to help solve key customer pain points or build an information business from de-anonymized and aggregated data.
Tech companies are the best positioned to undertake this analytics strategy, given the importance of best-in-class data science, engineering, data governance, and customer understanding foundations, along with a fast-paced, innovative product development model. Customer co-creation and data quality will be of utmost importance here, lest risking alienating your customer base with products that don’t deliver on their promise and miss the mark on adoption (and revenues!).
4. Future Ready: while admirable and undoubtedly ambitious, this is the riskiest strategy, particularly for companies that have not yet successfully executed the above three analytics strategies or whose DNA is not inherently high-tech, digital, or data-driven. This strategy requires the most investment, with a multi-year roadmap, and it’s not uncommon that organizations don’t see a positive ROI until years four or five.
While tempting to engage in a do-it-all, future-ready analytics strategy, I caution those who want to build or transform analytics organizations to choose intentionally between the first three strategic paths. Ideally, engage with operational optimization or customer focused initiatives for the first two years. It is a marathon, not a sprint.
By successfully demonstrating that your analytics teams have generated substantial cost savings for your company and delighted your customers (supported by increased NPS and CSAT scores and increased revenues and profitability), you will have more organizational trust and investment to carry out more complex and risky data monetization strategies.
Lesson 2: Structure Your Analytics Team the Right Way
The best model I’ve seen is a Center of Excellence (COE) deployment, which combines the positive elements of having a central analytics organization with the benefits of having data scientists and analytics product managers closely embedded with the various business units (your internal customers). Your team structure will primarily depend on the size of the company and your budget, the stage of the analytics journey, and transformational intent.
Larger companies making substantial investments in analytics teams – or striving toward a “future ready” strategy – should consider a centralized data science team reporting to a CXO or CEO. It grants you and your team the autonomy to manage and deploy your budget, prioritize enterprise-wide analytics projects according to effort and impact, and, if needed, maintain independence from the IT organization. Having an elevated, centralized COE structure also enables you to garner the necessary senior executive support to ensure that you can be impactful across various business functions. It also drives top-down support for your analytics agenda from second- and third-layer business unit leaders and managers.
For smaller companies focused on specific analytics improvements (e.g., improve sales or marketing returns), embedding analytics within those respective business units is most practical. This approach has obvious benefits, primarily if key company pain points are concentrated in one to two functional areas or the leadership team is not yet ready to engage in an enterprise-wide analytics transformation. After delivering real, tangible value for those essential functions (e.g., building a promotional effectiveness platform, helping marketers understand ROIs, deploying a real-time sales effectiveness tool), you can make the case to expand and level up to broaden your analytics impact in the organization.
In small to mid-size enterprises, you may have more autonomy to structure your teams and cross-functional rhythms in a way that more closely aligns with your vision and experience. In larger enterprises, the decision may have already been made for you before being hired (i.e., as an output of a prior strategy consulting engagement by the company). Regardless of the analytics organization structure, be sure to consider three things as you are building your new team — or transforming an existing one:
1. Spend the first 90 days studying your company and industry in-depth, visit field leadership and various operators, and develop a strong understanding and articulation of key business pain points.
Learn how to frame those business problems well. Instead of making templated hiring decisions based on some formula around total company size, employees, and the number of business functions to support, start with the customer. Be clear about what internal and primarily external customer outcomes you want to drive toward, which will inform the projects, analytics skillsets, and headcount you need.
2. Prioritize your analytics projects based on development effort and organizational impact. It is a fundamental concept but is often missed in planning sessions. Here’s a framework to help prioritize:
Low Effort, High Impact (Low-Hanging Fruit): Prioritize immediately.
High Effort, High Impact (Strategic Enabler): Plan meticulously and prioritize next.
Low Effort, Low Impact (Potential?): Consider for future.
High Effort, Low Impact: Disregard these projects.
3. When building analytics solutions and products for your internal stakeholders or customers, have a product management mindset.
Depending on your analytics team’s structure, scope, and strategy, this can materialize differently. You can achieve this by assigning dedicated analytics product managers for each internal business unit (e.g., supply chain analytics PM, marketing analytics PM) or by structuring it around customer segments or solution categories.
The importance of a product manager mindset is often missed but has garnered some traction in recent years, especially in larger enterprises. It is essential if you want to build dynamic, sustainable, and scalable analytics solutions instead of just engaging in static analytics project deliverables. Even then, having a dedicated product manager, project manager, or product owner makes a stark difference. In larger, more complex corporations, I've seen a separation of roles: the product manager focuses on customer and market strategy, while the product owner deals with development and technical aspects.
In either case, having an analytics (or artificial intelligence or machine learning) product manager on your team will be a critical asset in ensuring you are working on the right things in the right rhythm and cadence. Most importantly, depending on your organization structure, these product managers can also serve as critical data translators between the analytics team members and the functional end users to maximize product acceptance and adoption.
Lesson 3: Proselytize and Democratize
Having top-down support from your CEO and senior leadership team is critical and is a prerequisite for achieving a data-driven transformation. Equally important is that you, the analytics leader, build a strong community of analytics supporters and champions across the organization. Tactically, this means that you should spend the first 12 months engaging in the following:
1. Please get to know vital organizational stakeholders personally and professionally and understand (and dissect) their significant business challenges that data and analytics can solve. Supporting functional leaders in supply chain, pricing, sales, finance, marketing, and IT will be essential. You must make analytics believers out of your functional peers (and functional superiors), so they can effectively champion and support your analytics initiatives.
2. Conduct frequent roadshows with functional teams and front-line operators so they understand the analytics journey at a high level and can tie it back to the specific domain areas. Helping the rest of the organization learn about vital statistics or machine learning concepts (in simple, non-technical language) and how it helps the company accelerate toward its goals is equally important. It could be carried out through analytics webinars, lunch and learns, or through special breakout sessions during functional all-hands meetings (i.e., an hour-long analytics breakout during the quarterly sales managers’ meeting).
3. Gain organizational commitment to investing in upskilling analytics translators for your enterprise. These SMEs will be critical to bridging the gap between your data science teams and your internal customers. The analytics translators will also help shape project requirements, provide input to machine learning models, and help with user adoption. While you will have your business conduits (usually the analytics product managers or owners), having manager, director, or VP-level analytics translators from the functional business units will be crucial. They will have the best of both worlds: deep domain expertise and a fundamental understanding of ML/AI can add value to their business.
4. Help democratize access to non-confidential data and descriptive or diagnostic analytics across the organization and develop citizen data scientists. I suggest you do the latter by partnering with your chief people officer and her learning and development department and procuring course offerings from a reputable analytics certification provider (e.g., Udemy, Coursera, Udacity, Datacamp).
Why is this last piece critical? As much as we’d like to deploy large-scale, complex, or transformational analytics initiatives, most companies have hundreds, if not thousands, of business problems that advanced analytics can solve more efficiently and effectively. Analysts and managers with solid domain expertise in key functional areas are best suited to attack these problems. Usually, these business problems will be small or medium in scope and can be effectively solved with data visualization, diagnostic analytics, foundational predictive analytics, or basic optimization methods.
Over 90% of data-driven business problems can be solved more effectively by these upskilled domain expert analysts versus relying on the data science COE.
Lesson Fourth: Parsimony Matters
It’s easy to become enamored with the latest technology or “flavor of the month” (generative AI anyone). As Richard Heimann describes in his book Doing AI, one common cause of failure is trying to find problems for analytical solutions rather than addressing genuine business needs. As suggested earlier, start by solving your organization’s “low-hanging fruit” (low effort / high impact). It is how you will begin to build trust, organization-wide acceptance, and, most importantly, drive immediate, measurable value to your customers. Even once you move on to strategic business problems, ensure you understand the situation well and break it down into smaller, more manageable chunks to work in iterative sprints.
For most strategic analytics problems, simple, parsimonious models are best. They may not be the most accurate, but they get the job done with the least amount of complexity and, more importantly, provide needed explanatory power to senior executives, functional leaders, and end users.
Of course, if maximum model accuracy, precision, or recall are paramount (i.e., life and death scenarios or if there’s significant economic value at stake), and if compute costs or interpretability are less of a concern, then more complexity and a black box approach are fine.
Regardless of how you carry out your analytics project, incorporate front-line employees (or customers, if you are externally facing) in developing your data product, from ideation to requirements gathering, testing, and ongoing refinements.
Developing a customer- and results-driven mindset early on will enable you and your team to deploy analytics solutions that address critical problems the customer cares about. Furthermore, incorporating customer co-creation in the development process will significantly improve the chances that your analytics product will be used, leading to value creation for your customers and your company.
Lesson Five: Focus on Retaining Your Analytics Talent
For most of us, work is not just a paycheck. Maslow’s hierarchy of needs also applies to our workplace, and most data scientists and engineers I’ve worked with have strong cognitive, self-actualization, and belonging needs. At a minimum, I encourage you to build a team culture and cadence that encompasses the following:
1. Give a choice to spend up to 10–20% of data scientist time on side projects. Let your talented team experiment and explore new opportunities. They will uncover new insights or build an analytics product that will generate substantial returns later down the road, or it will yield no financial benefits and will remain a healthy intellectual exercise. Either way, give your team members the flexibility to be creative and innovative and to satisfy their innate need for experimentation and learning. Google has famously espoused this concept which yielded substantial cultural and economic benefits to Google and other companies.
2. As the leader, be relentless about building a data-driven culture and ensure that your data scientists are embedded in essential business functions. It essential not only to sharpen their domain expertise for driving more significant analytics ROI but also to ensure that your analytics team members feel like integral parts of the organization.
3. Provide a platform for continuous learning in AI/ML courses, industry certifications (e.g., Google Cloud, AWS, Azure Cloud), and the option to attend 1–2 key AI/ML conferences a year. Challenge them to present at these conferences, which not only bolsters your company profile and helps with recruiting efforts but also contributes to greater learning.
4. Eliminate corporate bureaucracy as much as possible, empower your most talented data scientists with work flexibility and autonomous decision-making, and celebrate the small wins with them.
We’re still seeing a shortage of solid data talent in the market. With the success and continued acceptance of remote work, particularly in tech-related roles, your analytics talent is undoubtedly being tempted each week by competitors or firms from other industries. If you don’t keep your data scientists, data and full-stack engineers, or analytics product managers happy, someone else in your city or country will jump at the opportunity.
Summary
The terms digital transformation, artificial intelligence, machine learning, and, indeed, data science have been hyped and often overhyped over the last five years. Most digital or analytics transformation efforts have failed, partly due to hubris, over-investment, or a hyper-focus on finding business problems to advanced analytics solutions versus the other way around.
But they mostly failed as organizations have been too eager to jump on the AI bandwagon to differentiate themselves from the competition. They did so without a clear understanding of current capabilities, without building a pragmatic data strategy that aligns with their organizational goals and vision, and without transparent measurement systems in place that tie analytics investments (human and tech capital) to corporate KPIs.
There have been many positive aspects for companies in their attempt to monetize their data. They have attracted fantastic data talent and, to some extent, influenced their legacy workforce to be more data-driven. They have uncovered new business opportunities or fixed existing problems while upskilling their employees to be analytics translators or citizen data scientists. But most importantly, like me, these companies’ leadership and analytics executives have learned a great deal about what it takes to leverage data as a sustainable competitive advantage.
Ultimately, it’s never about the technology, the algorithm, or the AI hype. To succeed in your analytics journey, you must focus on people — your team, key stakeholders, internal collaborators, and customers — and solve business problems that make your employees’ or customers’ lives quantifiably easier. If we can do that through a KPI heatmap, a correlation matrix, or a simple regression, then so be it.
This article was sourced from Medium and originally published in Revology Analytics Insider.