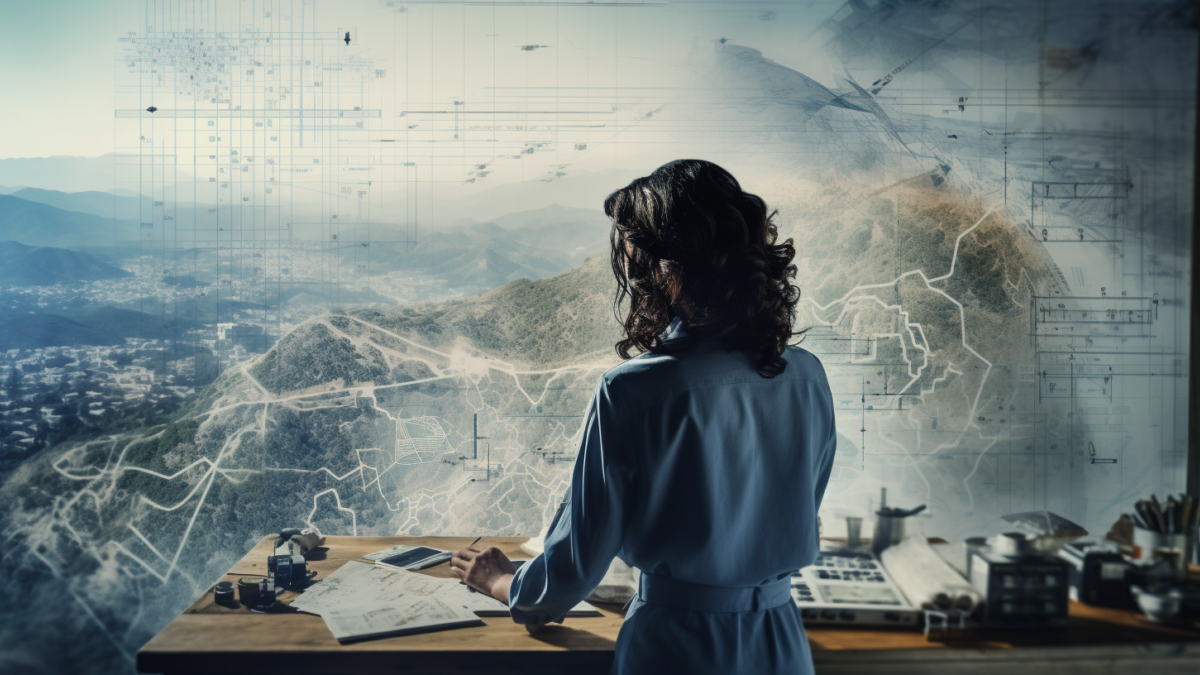
IIA has the fortune of working with some of the most interesting and recognizable brands in the world. As you might imagine, we get a lot of questions about AI strategy and one of the first questions we ask our clients is: Do you have a data strategy? A sound data strategy is the bedrock of a successful AI strategy, and our flagship data strategy framework has helped numerous clients set a vision, assess their current state, and build a roadmap for future success.
In this blog series, we’ll explore IIA’s 10-step data strategy framework. If you are a data and analytics leader building a program from scratch or re-seeing your data and analytics strategy, we encourage you to use this content and associated eBook to get started on your journey. For IIA Research and Advisory Network clients reading this post, you have access to the full framework and supplemental resources in the client portal. This article will cover setting a vision to characterizing the end state, with a follow-on post exploring building a roadmap and executing on your plan.
But first, let’s talk about what is at the center of a data strategy. There is a tendency to jump into something called a “data strategy” without understanding what it is at its core. In IIA's view, at the center of a data strategy is a schematic of an entity's information economy, with in-depth awareness of the constituent needs on the demand side of the economy—the data consumers. Because ultimately the primary purpose of a data strategy is to solve questions about how to improve the availability, timeliness, and quality of data, in that order of priority, for the people demanding it.
Although an understanding of supply-side abilities and willingness from the data providers is important, it must not lead in the process of discovery of demand's needs out of concern for the artificial limitations that may result. You need to start with a clear sense of the scope and depth of demand-side requirements, and not with "What do we have in the source systems?" or with "What kinds of intrinsic data issue—quality, risk, coverage—do we care most about?" or with technical issues about the storage or management of data sets.
The framework provided here explores the steps required to build a demand-intensive data strategy.
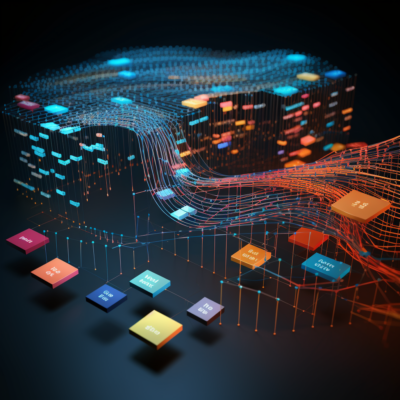
Creating A Data Strategy eBook
The growth in the demand for better decision-making with data means that a comprehensive data strategy is no longer a nice to have. You need a data strategy.
Download this eBook now to get actionable recommendations, on topics including context and vision for data strategy, creating proper objectives, developing a framework to evaluate where you are now and qualitatively assess your end state, and tips to plan your architectural moves and modify business benefit-delivering use cases.
Step 1: Set Context and Vision
Implementing a data strategy begins with asking yourself, "Why are we doing this?" To answer this, you must understand how the data strategy initiative links to your corporate strategy. If it does not tie directly to corporate strategy, then it must be in service of (that is, subordinate to) your analytics and AI strategy. If the corporate strategy doesn't provide obvious points of connection with "analytics" and/or “AI” or "data," and you don't have an analytics and AI strategy, then you probably shouldn't be doing a data strategy.
After establishing the context, the second step is to describe your vision: the end state to which you aspire, as seen by the more important stakeholders, and in particular by the demand-side of your information economy. Drawn from scenario planning disciplines, your aspirational end state should address what data management and data use deliver to the organization—and perhaps, to an extent, how that is experienced by the organization. At this stage, be aware that you may fail to uncover one or more stakeholders, and new stakeholders will probably grow over time.
Whatever concrete future-state description you choose, that description should be [a] benefits-oriented and [b] tied to corporate strategy and priorities, rather than the intrinsic state of data itself, or the technologies and processes for managing the data.
Step 2: Develop Strategy Summary
With the context and vision in mind, move on to summarizing your strategy at a high level.
- Objective: What you are seeking to accomplish, such as reducing costs, improving operational control, generating revenue, and managing risk.
- Scope: What you will do and what you will not do.
- Rationale: Why you've chosen this objective and scope.
- Expected benefit: Who will benefit from achieving the objective, and how they will benefit.
Now, you are ready to broadcast your plans outside the senior executive and CDO/CDAO teams. Socialize now, so that if you're off-base or incomplete, you get corrected before drafting presentations.
Don't move on until you've got that engagement, and addressed all feedback you've received, even if the answer to the feedback is the explicit exclusion of a given element of feedback. Business partners want to be heard and want to know they've been heard.
Step 3: Establishing the Core Information Model and Principles
The core information model and principles are the guiding “commandments” used to govern the resultant environment created by a data strategy.
A core information model is a definition of how your company chooses to treat its data. How you implement your data strategy depends on the way you think about data, so you need to settle on a core information model.
- Information as an Asset: Many companies treat data as an asset that must be carefully safeguarded and kept perfect at all times. Organizations that think data is an asset tend to adopt a finance-like, governance-heavy strategy.
- Information as Currency: Some companies treat data like currency in that it all has perceived value, so they want as much of it as possible with no necessary regard to how it could be used. Organizations that think data is currency will develop repository-heavy strategies—it's all about the safes into which we put our money.
- Information as Raw Material: The third option—viewing data as a raw material primarily for analytical work—treats data as useful only to the extent that you can transform it into an analytical (or in some cases transactional) product. Organizations that think about data as raw material for analytics will build flow and pipeline-oriented models: It's about processing, distribution, and delivery.
The distinction between asset, currency and raw material is important because the next step is defining the principles by which the organization will characterize important aspects of your data management model. In IIA's view, the closer you can be to managing your information as raw material the better — that viewpoint has the greatest tendency to reduce waste in resource allocation while maximizing effort against the most important business needs. However, we understand that this model cannot be implemented in every situation and perhaps should not be in certain others (e.g., IP-sensitive and trade secret data treated like an asset).
Your principles must be aligned to support the model and should be used to guide the behavior of everyone who operates in the data management environment, from source system owners through to human analysts.
Without a set of guiding principles, organizations often find themselves tackling the same issues with every use case, repetitively adjudicating the same kinds of conflicts and disputes, with little consistency across the use cases. Define principles around the following areas.
Access to Leverage:
Under what circumstances should you allow your expensive collection of data to be operated on and how do you want to encourage or ensure that usage of the data is done so in a way that benefits the organization.
Transparency, Quality, Fitness for Purpose: The role of a source system in a modern analytics environment is to make data available in as close to real-time as possible, at the lowest available grain, and to describe in as much detail as possible everything known, by the source system, about the semantics and quality of the data being provided (metadata). Distinguish between the needs of information consumers (singular truths like gross revenue) versus advanced analytics practitioners (state-of-nature data with signal and noise).
Lineage: The increasing use of third-party data and crowd sourced data increases the need to have a clearly defined point of view on the importance of data lineage.
Governance, Compliance and Control: Define under what circumstances governance, compliance and control will trump access and leverage.
Records Management, Retention and Destruction: Define when destruction trumps access or analytical requirements for longitude in a data set and define an adjudication process for handling conflicts. If your company does not have records management professionals to seek counsel on these principles, your chief legal officer should be able to help.
Privacy: Beyond PII and data obfuscation, think about privacy when it comes to analytical platforms being used by different constituencies. In our view, users of an analytical platform shouldn't have the expectation of privacy on that analytical platform. From the governance and security perspectives, everything done on the analytical platform should be visible, logged in exhaustive detail and available for ad hoc analysis by the team members responsible for curating the analytical environment.
Security: Define your authentication and authorization model for data access mechanisms, as well as security principles around data transparency, ease of access, and speed of resolution to access requests.
Self-Service: Your data strategy needs to address at least two aspects of self-service: 1) BI queries and reports, and 2) data preparation tasks. Further, it needs to decide what kinds of governance to apply to novel data sets created by self-service tools.
Managed Experimentation: At the experimental stage of analytical application development, failure rates are expected to be high, and therefore, the documentation of advanced analytics and AI experiments must be meticulous, well-archived, and easily searchable and recoverable in the future, whether by auditors, investigators, or other analytics teams, seeking to avoid past dead-ends and missteps.
Step 4: Current State Assessment
After you have reached agreement on core principles, perform a current state assessment. How does your operating environment today stack up against your aspirational end-state and your principles? You can't proceed until you have a clear idea of your capability and maturity in various areas, have communicated this with the stakeholders, and have reached agreement that the assessment reflects where you are now.
Assess areas such as:
- Inventory of internal and external systems of record or sources
- Data integration and metadata management
- Data modeling and architecture
- Data governance
- Decision-making processes and culture
- Business alignment and enablement
Include the following three groups in discussion:
- Functional and business owners who are the business beneficiaries, and likely the bill payers for internal transactional systems.
- Demand-side groups that have legitimate business needs for consuming data from particular analytical systems. For example, finance would be a legitimate constituency for Salesforce.com data because it is responsible for the pay cycles.
- IT system operators, who typically shoulder the run-and-maintain burden for both transactional and operational applications.
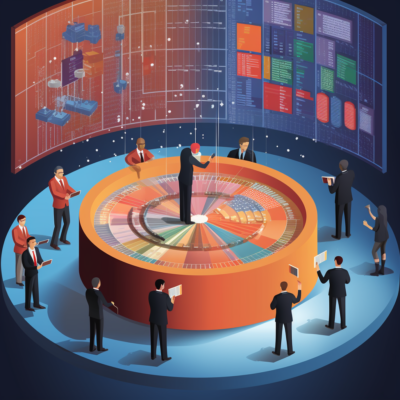
Analytics Maturity Assessments
Get in-depth diagnostic insights, recommendations, and benchmarking on your enterprise analytics maturity, your analytics organization capabilities, and the skills of your individual contributors.
Step 5: End-State Characterization
A good end-state characterization answers the question, “Where do you want to be by a defined time?” These days, a time horizon more than three years in the future is probably unrealistic; the rate of change in most business environments is simply too high to support five- or ten-year planning horizons.
Using the same criteria that you used for the current state assessment, score where you need to be along all the dimensions in the desired future state, which needs to be directionally aligned with your aspirational vision.
Be sure that your end-state description is more than an inventory of information. It should include considerations such as how fast data can be accessed, how accurate the data is, how easy it is for users to find the data they need, how straightforward it is to repurpose the data, how you will import data from outside the organization, and what correct data management will look like.
Conclusion
In this post we’ve explored the essence of a demand-intensive data strategy, and the steps required to set a clear vision that aligns with corporate objectives and assessing your current state. In our next featured blog, we’ll discuss how to take the vision you’ve set, the principles you’ve defined, the assessment you’ve undertaken, and then build and execute on a plan.