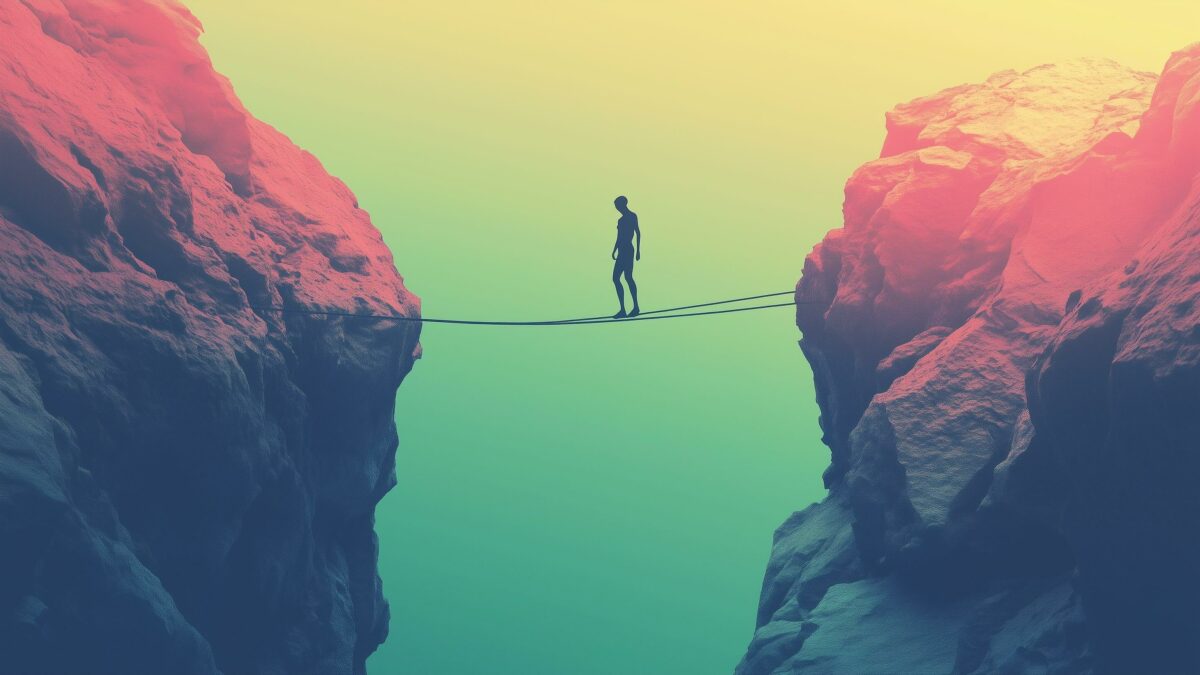
A client recently said to me, “I’m new to standing up an operating model for analytics, and I don’t even know if I fully understand what problem I’m trying to solve.”
It was such a good question, and so honest. And frankly, more D&A leaders should start there.
When organizations begin talking about changing or implementing an operating model, they often jump straight to structure: who should report to whom, where analytics teams should sit, what roles need to be centralized. But they miss the more foundational moment. Before you chart out a model, you need to ask:
- What’s not working?
- What are we actually solving for?
- And what kind of future do we want to build?
One leader I spoke with described inheriting a massive, fragmented enterprise. Fourteen hundred people. Functions spread across the globe. A marketing- and product-driven culture that outsourced most of its supply chain execution, but still had to deliver finished goods on time and across continents.
After a series of reorgs and layoffs, the org was unstable. Different teams were chasing GenAI use cases in every direction. There was no shared direction, no clear sense of identity. To put it bluntly, it was “choose your own adventure” chaos.
So they paused. Not to build another org chart, but to ask bigger questions:
- Do people understand where the organization is going?
- Can they see themselves in that future?
- Are we operating in a way that supports clarity, coordination, and business alignment—or are we optimizing in isolation?
These are operating model questions. And they’re essential questions to put on the table. They shape the day-to-day: how demand comes in, how teams collaborate, how priorities get set, how product development happens, and how value is defined and delivered.
If you don’t answer those questions, the structure doesn’t matter. You’ll get meetings. You’ll get slide decks. You won’t get scale.
And in federated organizations—where dotted lines are the norm and no single function owns the entire delivery lifecycle—that lack of clarity becomes fatal. You get the appearance of alignment, but no shared outcomes. You get Centers of Excellence that don’t struggle to improve performance. You get Communities of Practice that are communities in name only.
That’s what my next blog series is about: helping analytics leaders stop the cycle of oscillating between centralized and decentralized analytics operating models—and start designing for how work really happens. A practical, no-nonsense exploration of what IIA believes is the optimal state for mature (or maturing) D&A organizations: the federated analytics operating model.
If the content in this series resonates with you, I encourage you to download our new eBook titled “A Practical Guide to Building a Federated Analytics Operating Model.” We’re proud of the finished product and eager to share it with the data and analytics community. This blog series is inspired by the content in this guide, where you’ll find a robust discussion of federated analytics, and tools and resources to help you plan, socialize, and act.
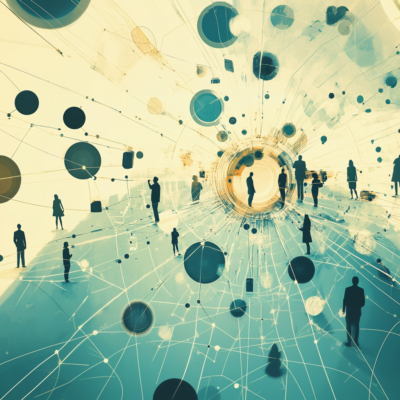
Struggling to scale analytics without sacrificing speed or consistency?
This practical guide explores how a federated operating model can help complex enterprises balance autonomy with alignment, unlocking sustainable, enterprise-wide impact. Discover actionable strategies, pitfalls to avoid, and real-world lessons from organizations that made it work.
The Pain of Organizing Analytics
At IIA, we’ve spent over a decade advising organizations—particularly those not born digital—as they attempt to escape the limitations of overly centralized or highly fragmented analytics ecosystems. The pattern is clear: the farther an enterprise is into its transformation journey, the more it begins to resemble a federated analytics operating structure.
For most enterprises, however, the structure of their analytics function wasn’t designed. It evolved. One team grew from within IT, another spun out of finance, a third was born out of urgent reporting needs during a crisis. Over time, these efforts expanded organically, often in isolation. The result is something we’ve seen repeatedly in our work: an unintentional analytics ecosystem—fragmented, duplicative, and difficult to govern.
When the business asks for insights, they’re met with delays. When data arrives, no one’s quite sure if they’re looking at the “right” version. Analysts spend more time wrangling than analyzing. Senior leaders wonder why, after all the investment, the value remains elusive.
Organizations typically react in one of two ways:
- Centralize analytics under a single enterprise function in search of efficiency and control.
- Distribute analytics back into business units to regain responsiveness and alignment.
Each approach solves some problems while creating others. Centralization often slows delivery and distances analytics from decision-makers. Distribution enables speed, but leads to tool sprawl, conflicting metrics, and rising support costs. We call this the oscillation trap—the tendency of enterprises to swing between extremes without ever solving the core issue.
Why Federation?
A federated model attempts to address this tension—not by choosing one side, but by designing a system where centralized and distributed capabilities work in concert.
In this model:
- A central team maintains shared infrastructure, platform governance, and enterprise-level capabilities
- Embedded teams are empowered to serve local needs while operating within shared frameworks and standards
- Governance, funding, and community development are treated as first-class design considerations
Federation is not simply decentralization with more meetings. It’s a purposeful structure that aligns autonomy with accountability and enables analytics to scale with trust and speed.
The Analytics Operating Model Continuum
No enterprise sets out to build an inefficient analytics organization. Yet many find themselves with exactly that: a sprawling ecosystem of teams, tools, and data assets that doesn’t quite work together. The root cause, more often than not, lies in the operating model.
How analytics is structured—where people sit, who owns what, and how decisions are made—shapes everything from delivery speed to data trust to how effectively insights influence outcomes.
Centralized vs. Distributed: A False Binary
Most organizations default to one of two poles:
Centralized
In a centralized model, analytics is managed by a core enterprise team—often under the CIO, CDO, or CAO. This team is responsible for everything from data engineering and dashboard delivery to platform governance and tooling strategy.
Centralized models often emerge during periods of transformation or after failed attempts at decentralization. The rationale is sound: reduce duplication, create consistency, and consolidate scarce talent.
And for a while, this works. Standards improve. Visibility increases. But over time, new problems surface:
- Business teams grow frustrated by long delivery cycles
- Shadow analytics teams begin to emerge
- Tooling decisions feel disconnected from business needs
- The central team becomes a bottleneck
Distributed
In response, organizations often swing to the other end of the spectrum: embed analytics directly into business units.
Here, teams operate independently, using their own tools, definitions, and data pipelines. They move faster, align closely to functional priorities, and often gain credibility within their domain.
But the speed comes at a cost:
- Multiple versions of the truth proliferate
- Enterprise data strategies fracture
- Support costs escalate
- Cross-functional analytics becomes difficult, if not impossible
In our experience, neither model is sufficient on its own. Each solves one set of problems by creating another.
Introducing the Federated Analytics Operating Model
A federated analytics operating model seeks to blend the strengths of both centralized and distributed models—while mitigating their respective downsides.
At a high level:
- Central teams provide shared infrastructure, common data products, governance, and advanced capabilities
- Embedded teams remain close to the business, delivering insights at the speed of decision-making
- Governance and funding models are redesigned to support collaboration rather than control
Federation is not a compromise. It is a structure built around the realities of large enterprises—where no single team can meet the entire organization’s analytical needs, and where consistency and autonomy must coexist.
In a federated model, autonomy is preserved, though not unbounded. Governance is enforced and not suffocating.
Why So Many Enterprises Get Stuck
Organizations often oscillate between these models—not because they’re indecisive, but because each model addresses pain points the other creates.
- When centralized models slow to a crawl, business units break away.
- When distributed models spiral into inconsistency, leaders push for consolidation.
- And so the cycle repeats.
This oscillation is understandable. But it’s not sustainable.
What’s missing is a deliberate model—a framework that defines roles, expectations, and operating norms across both central and embedded teams.
Federation is that model.
But federation is not just a midpoint between two extremes. It’s a distinct structure that requires design, governance, community curation, and investment. It brings with it new challenges: how to resolve disputes over ownership, how to coordinate investments, how to align measurement across distributed teams. But when done well, it unlocks scale, trust, and speed.
A Note on Readiness
Not every organization is ready for federation. Some are too early in their analytics maturity journey. Others are in the midst of rebuilding foundational capabilities like data quality, governance, or cloud infrastructure. Federation works best when the organization has a baseline platform in place, growing business demand, and enough distributed capability to make coordination worth the effort.
In our next article, we’ll look at the design principles that make federated analytics work, and the tensions you’ll need to manage along the way.
Until then, remember to always begin with these fundamental questions: What’s not working? What are we actually solving for? And what kind of future do we want to build?
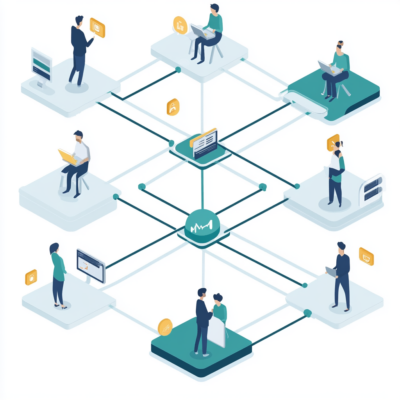
Everything You Need to Know about Federated Analytics
Our hub for all things related to federated analytics models. Read on to see why more enterprises are shifting to federated analytics and how to do so successfully.