Looking to design a federated analytics model that sticks? You’re in the right place. Download our free Practical Guide to Building a Federated Analytics Operating Model to the right, or keep reading to explore key questions leaders are asking:
- What is a federated analytics operating model?
- Why are more enterprises shifting to federated analytics?
- What does a healthy federated analytics operating model look like in practice?
- What are the most effective ways to transition to a federated model?
- How do you design a federated ecosystem that actually works?
- What causes federated models to fail—and how can you avoid it?
A Practical Guide to Building a Federated Analytics Operating Model

What is a federated analytics operating model?
Most enterprises didn’t design their analytics function—they inherited it. Over time, analytics capabilities emerged wherever there was urgency: a team in IT to support reporting, another spun out of finance, others formed within business units to serve their own needs. These teams evolved independently, without a shared model or operating agreement.
The result is something we see often: a fragmented analytics ecosystem. Different teams define metrics differently. Analysts spend more time wrangling data than analyzing it. Insight delivery slows, while business leaders grow frustrated and skeptical of the results. Despite investments in tools and platforms, the value remains elusive—and the root cause is rarely technical. It’s structural.
To solve this, many organizations toggle between two well-known models. Some centralize analytics under a single enterprise team, hoping to drive consistency and control. Others distribute analytics to individual business units to boost responsiveness and relevance.
Each model addresses a pain point while creating new ones. Centralization often leads to bottlenecks. Distribution leads to tool sprawl, duplicated effort, and growing mistrust in shared data.
We call this the oscillation trap—the cycle of flipping between centralized and distributed structures, without ever addressing the deeper issue: the lack of an intentional operating model.
This resource hub explores a better alternative: the federated analytics operating model.
Federation isn’t a midpoint or compromise. It’s a distinct structure where centralized and distributed capabilities are designed to work in concert. Central teams provide shared infrastructure, enterprise-level governance, and scalable assets. Embedded teams retain autonomy (mostly) to deliver insights that align closely with business needs—while working within shared frameworks that support consistency and trust.
Federation works best for organizations that have experienced the tradeoffs of other models and are ready to build something more sustainable. It offers a way to organize analytics in line with the scale and complexity of modern enterprises—but it requires structure, community, and clarity.
In the sections that follow, you’ll find a practical exploration of what makes federation effective, how to assess your organization’s readiness, and what it takes to design, scale, and sustain this model in the real world.
Why Organizing Analytics Is Always a Moving Target
Your analytics model will shift—centralized, decentralized, hybrid. That’s not a problem; it’s a reality. Centralization can create consistency, but over time, functions will evolve on their own. Leaders who stay close and actively shape what gets built—and where—will drive more value. You’re going hybrid either way. The only question is whether you’ll lead the change or be forced to catch up.

Why are more enterprises shifting to federated analytics?
Federation is gaining traction for a reason: most enterprises have learned the hard way that their current model—centralized or distributed—isn’t working. Centralized analytics functions bring consistency but often become bottlenecks. Distributed models allow for speed and business alignment but quickly unravel into fragmentation, inconsistency, and duplicated effort. Organizations bounce between these two extremes, solving one problem only to create another. We call this pattern the oscillation trap.
A federated analytics operating model offers a more sustainable solution. It’s not a midpoint between centralization and decentralization. It’s a distinct, purpose-built structure that recognizes the realities of modern enterprises: no single team can meet the organization’s full analytics needs, and no business unit can succeed in isolation.
In a federated model, the central team manages the shared infrastructure, maintains enterprise data products, and leads governance, compliance, and advanced capabilities. Embedded teams stay close to the business and deliver insights at the pace of decision-making. Both are connected through shared standards, handoff mechanisms, and lightweight but deliberate governance structures that promote collaboration over control.
Organizations don’t usually start with federation. They arrive there after experiencing the limits of other models. Centralized teams struggle to scale and lose business trust. Distributed teams move quickly but create long-term risks. Federation provides a path forward—if it’s implemented with clear roles, deliberate design, and mutual accountability.
Federation isn’t easier. It’s more honest.
Done well, federation surfaces the structural tensions most enterprises already face. It doesn’t eliminate them—it makes them navigable. Speed versus standardization. Autonomy versus accountability. Coordination versus agility. Federation doesn't pretend these tensions don’t exist. It builds the operating framework to manage them intentionally.
This shift requires leadership alignment, a foundation of shared infrastructure, and enough embedded talent to make distributed ownership feasible. But it also requires mindset change: collaboration must be designed for—not hoped for. Governance must clarify rather than constrain. And every team—central or embedded—must see itself as part of a system, not an island.
In practice, successful federated models resemble ecosystems more than hierarchies. They include central teams that curate shared data products and services, embedded teams delivering local insights, and cross-functional mechanisms to guide investments, tooling decisions, and talent development. When working well, this model enables analytics to scale without losing trust, and to adapt without losing coherence.
The next section breaks down what this looks like in operational terms—how responsibilities are distributed, how MVPs move from local to enterprise-grade, and how a federated model delivers real business value over time.
Federated Analytics Readiness Assessment
Use these five diagnostic areas and checklist to signal your likelihood of success in moving to a federated analytics model.
Roundtable Peer Insights: Building Successful Federated Analytics Teams
Download key themes and peer insights from an invite-only, peer-to-peer discussion on defining and designing federated analytics—and overcoming organizational resistance.

What does a healthy federated analytics operating model look like in practice?
Federation isn’t a loose structure or an aspirational concept. It’s a practical, deliberately designed operating model—one that defines how analytics work gets done, how teams coordinate, and how data products move from local experiments to enterprise assets.
At its core, a healthy federated model rests on three interdependent layers. The central analytics team owns shared infrastructure and governance, provides advanced capabilities, and enables consistency across the organization. Embedded analytics teams sit within business units, tailoring insights to functional needs and decision timelines. Between and across them exists a third layer: governance and community, which ensures clarity, alignment, and trust.
When these layers are clearly defined and well-connected, federation gives organizations the best of both worlds: enterprise coherence and business agility.
A federated model succeeds when responsibilities are not just assigned—but understood. The central team is more than a platform steward. It’s a service provider, standards bearer, and internal consultant. It manages the data platform, oversees shared assets, and curates the analytics community through onboarding, playbooks, and practice groups.
Embedded teams, in turn, serve business decision-makers directly. They build MVPs, shape business-specific metrics, and adapt shared tools to local needs. Their proximity to the business makes them fast. Their connection to the broader federation makes them aligned.
But this balance only works with intentional design. Shared operating procedures—like RACI matrices and handoff protocols—clarify who owns what. Advisory councils help resolve conflicts and shape platform evolution. Community infrastructure ensures analysts aren’t working in isolation, even if they’re seated in different parts of the business.
One essential mechanism that keeps the system working: the MVP handoff. Local teams often build fast, functional tools to solve immediate problems. In a federated model, these MVPs can be evaluated for broader use, then hardened and maintained by the central team. Without this pipeline, valuable solutions remain siloed—or get rebuilt from scratch elsewhere.
Culture matters just as much as process. Successful federated organizations invest in community-building—not as an HR initiative, but as a strategic enabler. They create opportunities for shared learning, secondments between teams, and common language across roles. Analysts see themselves not just as function-specific problem solvers, but as members of a coordinated enterprise system.
The payoff isn’t perfection—it’s productive friction. Teams operate with clarity. Innovation flows in both directions. Tools are used intentionally, with room for justified exceptions. New hires ramp faster. Analysts stay longer. And trust in analytics grows, because the system behind it is coherent.
In the next section, we’ll explore why so many organizations choose to pursue federation—not only to address current frustrations, but to reduce the long-term risks of staying in a model that no longer fits.
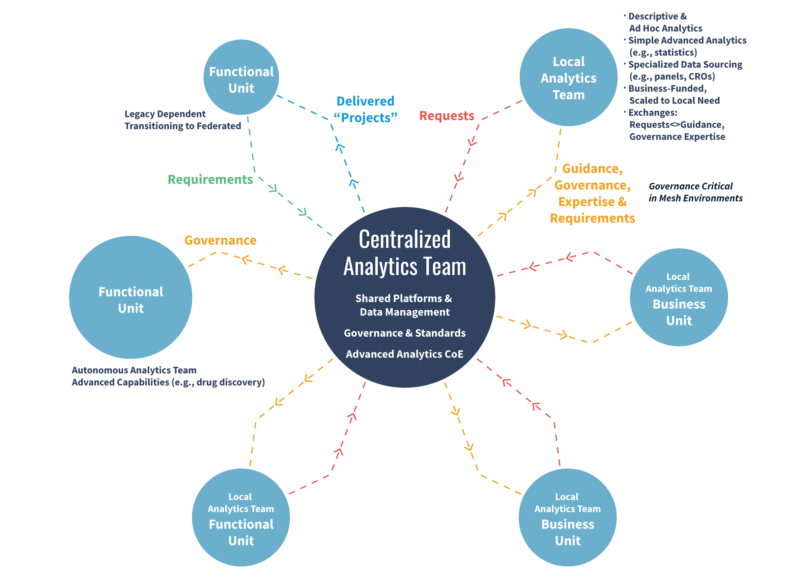

What are the most effective ways to transition to a federated model?
Recognizing that your current model no longer works is an important step. But moving toward federation requires more than insight—it demands a transition strategy that fits your organization’s realities. Culture, leadership alignment, platform maturity, and team structure all shape what kind of change is possible and how quickly it can take root.
Through our work with complex enterprises, we’ve seen three primary strategies used to initiate federation. While the end goal is similar—structuring analytics to scale with both autonomy and alignment—the path you take should reflect where you’re starting and what your organization is ready to absorb.
1. Formalize and Reorganize
This is the most direct—and most disruptive—approach. It begins with intentionally designing the federated model: defining roles, documenting processes, redrawing team boundaries, and establishing how work will be coordinated. The organization then realigns resources to support that design.
This strategy tends to work best when executive leadership is already aligned, the need for change is urgent, and previous coordination attempts have fallen short. Teams are reorganized, responsibilities are reassigned, and federation-wide policies—like handoff mechanisms and governance expectations—are rolled out in parallel.
The tradeoff? Resistance is high. Embedded teams may worry about losing control or relevance. Business stakeholders may see reorganization as bureaucracy. But with clear rationale and strong leadership, this strategy delivers the fastest path to long-term clarity.
2. Serve the Underserved and Build Community
Not every organization is ready for a top-down redesign. In more distributed or politically sensitive environments, a lower-friction approach often works better: focus first on delivering value where analytics support is weakest. These teams often welcome help and become early champions of the model.
As the central team earns trust, it can introduce lightweight governance, shared onboarding materials, and internal forums that reinforce federation as a community—not just a structure. With time, these norms spread to other teams, supported by documented success stories and growing peer influence.
This strategy is slower, but often more sustainable. It prioritizes service over enforcement and gives local teams space to opt in. The key is to treat each pilot not as a siloed success, but as part of a larger, evolving model.
3. Leverage Platform Retirement as a Forcing Function
For some organizations, the trigger isn’t strategy—it’s infrastructure. When legacy systems are being retired or modernized, the transition can serve as a powerful catalyst for structural change.
Instead of treating a platform migration as a technical upgrade, organizations use it as an opportunity to redefine how work is organized. Teams migrating to the new environment are also introduced to federation expectations—whether that’s clarified ownership, new collaboration processes, or shared governance routines.
This strategy works best when there’s urgency to migrate but also openness to rethink roles and responsibilities. The risk is rushing governance decisions or overlooking the cultural work required to support the model. But when paired with careful planning, this moment of transition can become a structural reset.
4. What Every Strategy Needs to Succeed
Regardless of which transition path you choose, successful federated models share several enabling factors.
You need clear documentation—especially RACI matrices, SOPs for data and tooling, and a community charter to support shared behaviors. Funding must shift from project-by-project requests to roadmap-aligned investments, with a clear plan for long-term maintenance. Embedded teams need time, incentives, and leadership support to participate in design and governance efforts. And the central team must reposition itself—from a gatekeeper enforcing rules to a strategic partner enabling scale, reuse, and delivery.
Federation can’t be imposed. It has to be co-created.
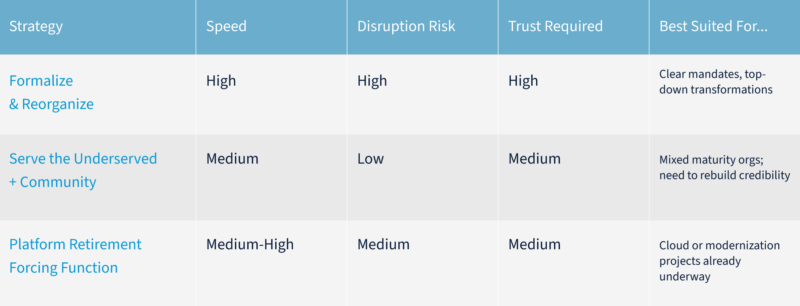
Each of these strategies can lead to a healthy federated model—but only if the organization is honest about where it stands today. Federation isn’t just about where you’re going—it’s about how you get there.
In the next section, we’ll look at what a federated ecosystem actually requires to function: the systems, norms, and decisions that enable distributed teams to work in concert rather than in conflict.

How do you design a federated ecosystem that actually works?
Federation isn’t a theory. It’s a system of structures, agreements, and practices that must work in sync. While the concept is easy to understand—shared responsibilities across central and embedded teams—making it work at scale requires operational clarity, cultural investment, and design discipline.
In this section, we break down what high-functioning federated ecosystems look like in practice, drawing from IIA’s work with large, complex organizations across industries.
1. Start with a Platform That Enables Autonomy
Federation relies on autonomy, but autonomy doesn’t happen in a vacuum. It happens on top of shared infrastructure. A centralized platform—built for distributed use—is the backbone of any federated model.
To support autonomy without chaos, your platform should do more than serve data. It should:
- Provide high-trust, one-version-of-the-truth data products for enterprise-wide use
- Support secure, role-based access with governance guardrails built in
- Make lineage, semantic metadata, and documentation visible and actionable
- Enable handoffs: MVPs built by embedded teams must flow easily into enterprise-grade assets
When these foundations are weak or unclear, embedded teams either build their own systems—or stop trying. That’s not autonomy. That’s fragmentation.
2. Clarify What Gets Governed—and By Whom
Many organizations assume federation weakens governance. In reality, it demands a more sophisticated version of it. Not more control—just more clarity.
Effective models define:
- What gets governed centrally (e.g., enterprise metrics, high-risk data, security protocols)
- What can be governed locally (e.g., domain KPIs, exploratory dashboards)
- How shared definitions are created, reviewed, and maintained over time
A tiered governance model makes this possible. It distinguishes between assets that require rigor and those that benefit from speed and context. The goal isn’t uniformity—it’s traceability and trust.
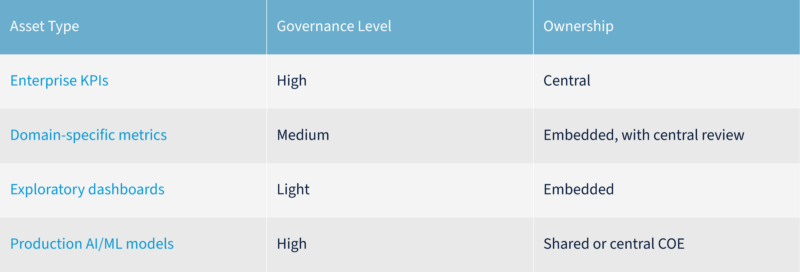
3. Build Community Like It’s Infrastructure
Federation is just as cultural as it is structural. It succeeds when analytics professionals feel like part of a broader system—not just members of their own team.
That sense of shared identity doesn’t emerge on its own. It must be curated.
Leading organizations invest in:
- Onboarding that introduces all analytics hires—regardless of role—to the federated model, shared tools, and common principles
- Rotational programs that allow embedded analysts to work in central teams (and vice versa), building empathy and fluency
- Recurring forums where wins, challenges, and reusable solutions are shared
- Shared taxonomies and methods so teams speak the same language when they collaborate
The most effective federated ecosystems treat community infrastructure with the same seriousness as data pipelines.
4. Operationalize Reuse and Productization
In a well-run federated model, innovation doesn’t live or die in silos. Local MVPs become enterprise assets. But this doesn’t happen by accident—it requires process.
Embedded teams should be empowered to build scrappy, business-aligned tools. But once those tools demonstrate value, they need a pathway for broader use. That means:
- Clear criteria for identifying reusable assets
- A structured transition process (documentation, ownership change, platform support)
- Feedback loops to improve and evolve shared data products
Federation works best when teams know they can create locally—but also contribute back to the enterprise ecosystem.
5. Make the Operating Model Tangible
You can’t scale what you can’t describe. That’s why a federated model needs to be codified—not to create bureaucracy, but to enable continuity and trust.
Every federated organization should have:
- A federation playbook that documents roles, responsibilities, handoff rules, and escalation paths
- A living data product catalog that identifies owners, usage patterns, and product status
- SOPs for how decisions are made: tooling selection, data promotion, metric conflict resolution, and more
These documents aren’t just for onboarding. They’re reference points for day-to-day decision-making. And they should evolve as the model matures.
6. Design for Flexibility—Without Losing Coherence
Not every team in a federated model will operate the same way. Some are advanced. Others are just getting started. That variation is healthy—but it must be supported without sacrificing the system as a whole.
Organizations do this by introducing mechanisms like:
- Pod models, where central experts are embedded part-time in high-need areas
- Tiered services, where support levels match team maturity
- Bid processes, where embedded teams request resources aligned to enterprise priorities
Flexibility isn’t a concession in a federated model—it’s a design principle. But it has to be structured.
7. Align Roadmaps, Not Just Teams
Federation requires more than a well-drawn org chart. It requires shared visibility into what’s being built, when, and by whom—across all layers of the model.
Leading organizations maintain coordinated but not monolithic roadmaps:
- A data product roadmap showing what’s being built centrally and what’s in the promotion pipeline
- A tooling roadmap tracking adoption, retirement, and experimentation
- A community roadmap guiding learning initiatives, hiring priorities, and governance evolution
These roadmaps don’t need to be perfect. But they must be visible—and reviewed regularly across teams.
Webinar: The Federated Analytics Operating Model
Watch this webinar for a deeper dive into why centralized and distributed analytics operating models often fail to scale, core design principles and starting blueprint for federated analytics, and common pitfalls and key considerations.

What causes federated models to fail—and how can you avoid it?
A federated analytics model can deliver enormous value—but only if it’s implemented with care. In our experience advising enterprise leaders through this transition, the greatest risks aren’t technical. They’re structural, behavioral, and cultural.
Organizations often embrace federation in theory, but carry forward old habits in new packaging. What results is a partial implementation—one that lacks cohesion, trust, or traction. This section outlines the most common pitfalls that undermine federation efforts and offers practical guidance to help you avoid them from the outset.
1. Mistaking federation for informal decentralization
One of the most common failures is mistaking federation for “decentralization with some extra meetings.” In this scenario, teams are granted autonomy without the infrastructure or operating norms that enable it. There’s no data catalog, no shared standards, no visible governance. Local teams make their own tooling decisions and deliver value—until scale reveals the cracks.
This happens when federation is seen as a political compromise, not an intentional design. Leaders worry that too much structure will alienate the business, so they settle for informal alignment. But without documented agreements—SOPs, RACIs, shared definitions—confusion and redundancy grow.
To avoid this, federation must be positioned as a system: freedom within a framework. Even early iterations need enough structure to clarify expectations and build momentum.
2. Over-centralizing under the banner of governance
On the opposite end, some organizations use federation as a justification to extend control. The central team retains ownership of nearly everything—platforms, data products, tooling decisions—while embedded teams are expected to comply without influence.
Here, governance becomes more about restriction than enablement. Embedded teams are nominally empowered, but lack the access or authority to act independently. Often, this stems from previous decentralization failures, which breed fear and rigidity.
The antidote is shared governance. Embedded teams must co-create standards, participate in roadmapping, and influence tooling decisions. Governance should feel like support, not surveillance.
3. Underinvesting in community and shared identity
Federation depends just as much on informal collaboration as formal processes. But many organizations skip the cultural work, assuming community will emerge on its own.
Without a sense of shared identity, analysts revert to silos. Wins aren’t shared. Teams solving similar problems remain disconnected. Onboarding is inconsistent. Local work becomes invisible to the broader ecosystem.
Community-building must be funded, facilitated, and treated as strategic infrastructure. Forums, cross-team summits, shared onboarding experiences, and recognition programs all help foster a sense of alignment. Federation without community is just fragmentation with a friendlier name.
4. Ignoring the MVP-to-product handoff
A major strength of federation is its ability to let innovation emerge locally—but that’s only valuable if there’s a way to scale it.
Too often, embedded teams build excellent MVPs that never progress beyond their original use case. Without a defined handoff process, those assets become technical debt or die when their creators move on. The central team, unaware of these MVPs, may rebuild them from scratch.
Federation requires a pipeline: criteria for productization, documentation standards, transition support, and incentives for reuse. Innovation must be seen as part of a system—not a side effect of individual initiative.
5. Running federation on outdated funding models
You can’t sustain a new operating model on old financial habits. If the central team lacks funding to support delivery, and embedded teams are stuck lobbying for headcount, federation stalls.
Too often, data products are funded once, with no budget for run-and-maintain. Or platform investments are decoupled from the needs of business teams. Federation becomes symbolic: a new name, same constraints.
To fix this, finance leaders must be brought into the conversation. Portfolio-based funding, pooled budgets, and service-level agreements can help align dollars with responsibilities. Run-and-maintain must be baked in—not treated as an afterthought.
6. A mindset shift: from reactive to resilient
At the heart of each of these pitfalls is a misalignment of mindset. Federation isn’t just a structure—it’s a way of working that requires new assumptions, new behaviors, and sustained leadership support.
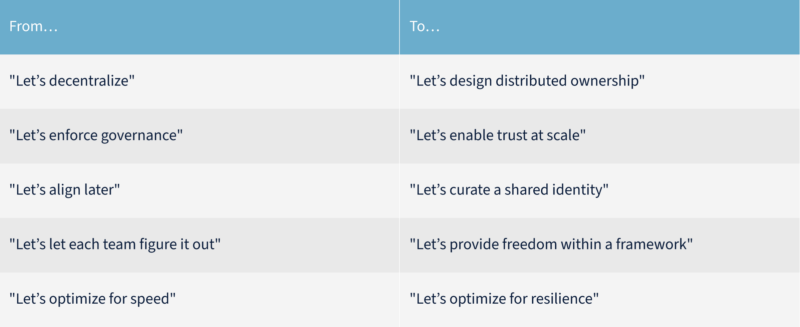
Avoiding failure in federation means treating it as an operating system—not a reorg. One that makes ownership visible, trust scalable, and friction productive.
Looking for more?
Realize analytics advancement with IIA
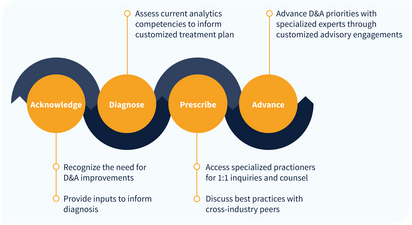

What top organizing model questions are IIA Experts fielding right now?
Here’s what your peers are struggling with and how IIA’s Experts helped them make progress:
- We’re mapping out our five-year data science roadmap. How should we start building the team—centralized or federated, generalists or specialists?
- How do you embed analytics teams in business units while maintaining their allegiance to the analytics function—without letting them go native?
- What does it take to establish a culture that runs the business by the numbers, from descriptive insight to prescriptive action?
- We’re starting a CoE model with a central team and dotted-line analysts. Any early lessons on how to make it work?
- How do we transition from a centralized analytics team to a federated model—without losing trust, alignment, or speed?
- How do we allocate resources and plan support as we shift from centralized analytics to a federated, self-service model?