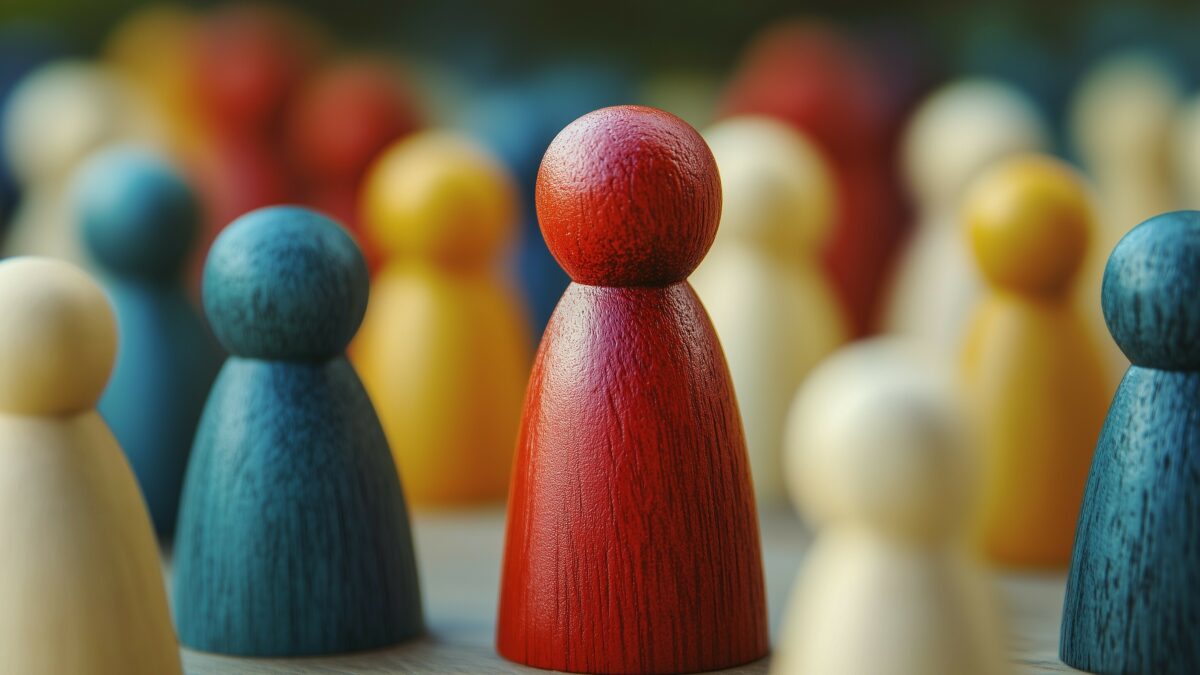
IIA clients leverage analytics maturity assessments, battle-tested frameworks, and cross-industry collaboration to prioritize and execute strategic enterprise data and analytics initiatives. We regularly check the pulse of trending topics for our community and facilitate critical conversations in virtual roundtable format for peer-to-peer exchange.
In a recent IIA roundtable discussion, data and analytics leaders from diverse industries explored the challenges and best practices of federated operating models. Participants shared insights into structuring federated teams, overcoming governance hurdles, managing change, and striking effective collaboration across business units. Below are the key takeaways from the conversation:
1. Defining the Federated Model: A Spectrum of Approaches
Federated models in data and analytics vary widely based on industry, organizational maturity, and strategic objectives. Some companies operate fully centralized teams, while others adopt a more decentralized approach with embedded analytics functions across business units. The range of models is vast, and there is no single correct approach. Instead, organizations must tailor their federated model to their specific needs, balancing efficiency and flexibility.
One leader described their company’s journey from a fragmented, decentralized model to a structured hub-and-spoke system. Initially, their analytics efforts were spread thin across business units, with inconsistent methodologies and duplicated efforts. To address this, the company consolidated core analytics functions into a central team while maintaining subject-matter expertise within business units. This approach allowed them to standardize practices without losing the specialized knowledge required for different business domains.
Another participant emphasized that federated models should not follow a rigid structure. Instead, organizations must allow for differences in business function maturity. Some teams, such as those in supply chain or manufacturing, may have decades of experience with analytics and require minimal oversight. In contrast, functions like HR or finance may need more structured support. This tailored approach helps business units operate effectively while benefiting from an enterprise-wide strategy.
2. Governance: The Critical Foundation for Federation
A well-defined governance structure plays a crucial role in maintaining consistency, data quality, and collaboration in federated models. However, governance remains a common pain point for organizations attempting to implement federation. Without a clear framework, decentralized analytics efforts can quickly lead to fragmentation, conflicting data interpretations, and a lack of trust in enterprise-wide insights.
One participant shared how their organization struggled with governance in the early stages of adopting a federated model. Analysts across different business units were working independently, leading to significant discrepancies in reported metrics. To resolve this, they established a governance council responsible for defining enterprise-wide standards, improving data consistency, and fostering alignment between business and technical teams. This governing body played a crucial role in strengthening collaboration and building confidence in data-driven decisions.
Another participant described how governance must include both policy frameworks and enforcement mechanisms. Their organization initially implemented governance guidelines but lacked the ability to enforce them, leading to continued inconsistencies. They are now taking a more structured approach by integrating board-level policies that hold business leaders accountable for governance adherence. The aim of this shift is to establish stronger compliance and create more structured expectations across teams.
3. Balancing Centralization with Business Unit Flexibility
Striking the right balance between centralized oversight and decentralized execution remains a fundamental challenge for organizations adopting federated models. While centralization allows for standardization and efficiency, excessive control can stifle innovation. Conversely, too much autonomy at the business unit level can lead to siloed efforts that diminish the value of enterprise-wide analytics.
One participant described how their organization took an iterative approach to balancing centralization and flexibility. They started by centralizing data science and advanced analytics capabilities while allowing business units to retain control over operational analytics. However, this led to challenges in defining what belonged in the central hub versus what should be managed by business teams. To address this, they developed a framework that categorized analytics initiatives based on complexity, business impact, and required expertise. This helped clarify ownership and prevented unnecessary duplication of efforts.
A leader from a smaller organization highlighted how limited resources made a federated model the most practical option. Instead of trying to build a large central analytics team, they focused on developing semantic models and high-ROI data products that empowered business units to perform their own analytics. By demonstrating quick wins and delivering measurable business value, they gained traction for the federated model without requiring significant additional investment.
4. Change Management: Overcoming Resistance to Federation
Shifting to a federated model often requires a cultural shift, and resistance from both leadership and frontline teams is common. Change management efforts must be intentional, structured, and aligned with business goals to build long-term adoption.
One organization implemented a structured change management blueprint, engaging stakeholders at all levels. They conducted data hackathons to highlight the value of the federated model, integrated analytics training into leadership development programs, and embedded analytics adoption into competency models. These initiatives helped shift the organization’s culture, fostering a greater appreciation for data-driven decision-making.
Another participant shared that their organization initially faced significant pushback when transitioning to a federated model. Many business leaders resisted the change, fearing a loss of control over their analytics capabilities. To address this, they adopted a “coalition of the willing” approach, focusing on engaging early adopters and demonstrating tangible benefits before expanding the model enterprise-wide. Over time, as the value became evident, more teams opted into the federated framework voluntarily.
5. Measuring Success in a Federated Model
To make federated models effective, organizations must develop meaningful metrics to track progress. Traditional analytics delivery metrics are often insufficient; instead, organizations need to assess how well the federated model supports business outcomes.
Time-to-insight emerged as a key metric in the discussion. Many organizations are shifting their focus from measuring the number of reports generated to evaluating how quickly business teams can access actionable data. One participant described how they implemented SLAs for analytics delivery, ensuring that critical business functions like supply chain and operations received timely insights. These SLAs helped drive accountability and improve business decision-making.
Another organization linked analytics performance to financial outcomes, tying bonuses and incentives to P&L impact. By embedding analytics-driven decision-making into performance management, they created stronger alignment between business and data teams. Additionally, adoption rates of analytics platforms and adherence to governance frameworks were highlighted as critical indicators of success, reinforcing a commitment to data-driven decision-making across the enterprise.
IIA virtual roundtables are exclusive, invite-only discussions designed to promote peer-to-peer exchange on pressing challenges in the data and analytics community. Seats are limited and reserved for senior data and analytics leaders at mid- to large-sized enterprises. Conversations are geared toward non-digital native companies. If you meet these criteria, contact us for more information.