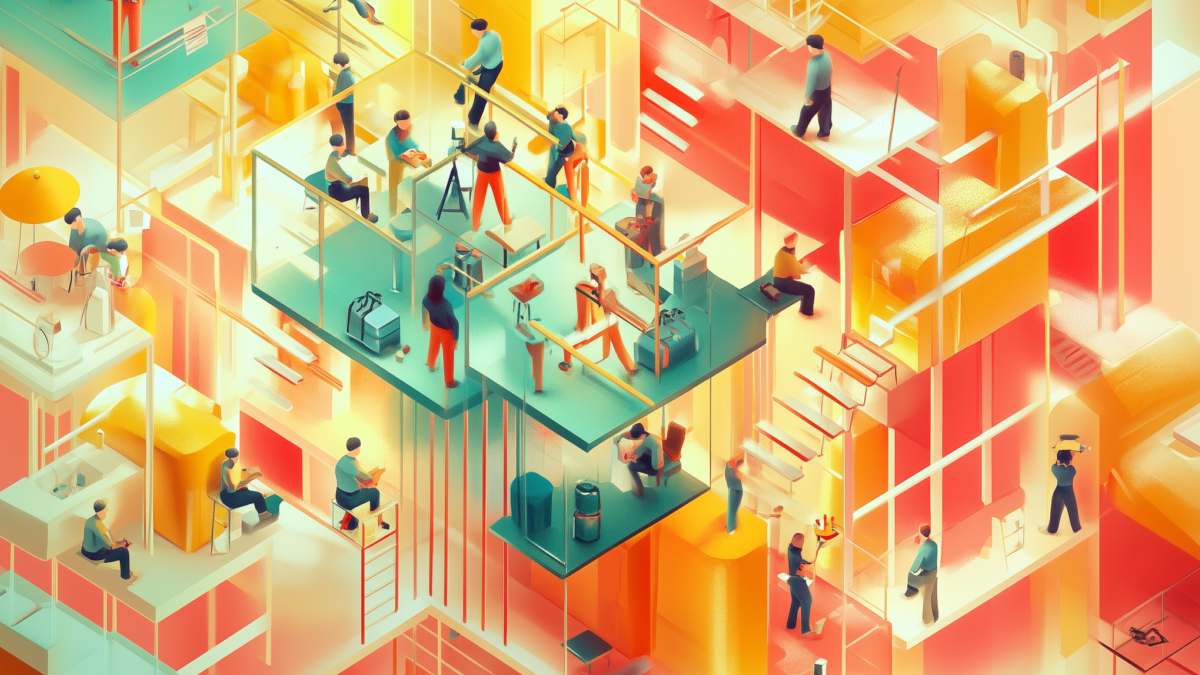
IIA clients leverage analytics maturity assessments, battle-tested frameworks, and cross-industry collaboration to prioritize and execute strategic enterprise data and analytics initiatives. We regularly check the pulse of trending topics for our community and facilitate critical conversations in virtual roundtable format for peer-to-peer exchange.
In a recent IIA roundtable discussion, data and analytics leaders from diverse industries demystified agentic AI and explored practical use cases. The discussion covered a wide range of topics, from challenges with integration to evaluating the effectiveness of agentic AI experiments.
This discussion was moderated by IIA expert Yousef Mohassab, CEO and co-founder of facilis.ai.
Here are the key takeaways:
1. Evolution and Broad Application of Agentic AI
Agentic AI is redefining the scope of automation by achieving complex task automation at a human-like level, which significantly enhances both customer service and backend operations. Unlike traditional AI that focuses on specific, rule-based tasks, agentic AI incorporates advanced decision-making capabilities that mirror human cognitive processes. This advancement is largely driven by recent breakthroughs in language model technologies, allowing for more nuanced and sophisticated applications.
These AI systems are increasingly being deployed in targeted, industry-specific contexts where they can provide the most value—ranging from customer engagement models in retail to operational workflows in manufacturing. For instance, in customer service, agentic AI systems are used to manage and respond to customer inquiries, learning from each interaction to improve both the accuracy and relevance of their responses over time. In marketing and content generation, these systems are creating dynamic content that adapts to user interactions, providing a more personalized experience.
2. Integration Challenges and Strategic Implementation
The integration of agentic AI into existing business processes presents several challenges, notably in technical implementation and strategic alignment. Data and analytics leaders emphasized that the success of agentic AI initiatives hinges not just on the deployment of technology but on its integration into the broader business strategy. This requires a clear understanding of the business processes that would most benefit from AI enhancement, coupled with a strategic approach to technology adoption.
Best practices identified during the roundtable include starting with narrowly defined applications to manage the complexity of integration effectively. For example, one leader mentioned beginning with automating simple yet time-consuming data entry tasks as a way to introduce agentic AI, gradually scaling up to more complex processes such as inventory management and customer relationship management as the system's capabilities are proven.
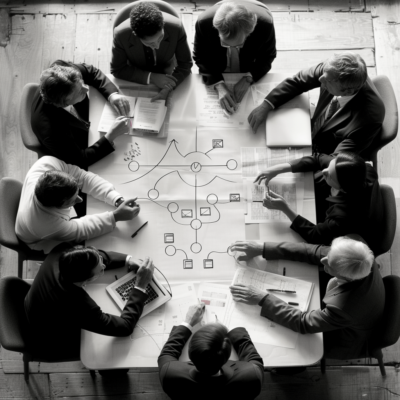
Analytics Leadership Consortium (ALC)
Leading analytics in a large organization is a daunting challenge. The Analytics Leadership Consortium (ALC) empowers analytics leaders to navigate the challenges of building enterprise-wide analytics capabilities. Through objective insights, peer collaboration, and expert guidance, ALC provides the tools and confidence needed to scale beyond proofs of concept and deliver impactful, enterprise-wide solutions.
3. Security, Governance, and Compliance
As agentic AI systems are increasingly applied in external-facing operations and critical internal processes, robust governance frameworks are essential to manage security and compliance risks effectively. The discussion highlighted the importance of developing these frameworks to ensure that AI applications comply with existing laws and maintain high ethical standards, particularly in sectors like finance and healthcare where data sensitivity is paramount.
Participants shared insights into the need for dedicated resources to address the heightened security risks associated with customer-facing applications of agentic AI. Developing these frameworks involves not only technical measures to secure AI systems but also organizational policies that govern their use and ensure they are in line with ethical standards.
4. Future Directions and Ethical Considerations
Looking ahead, the roundtable participants recognized the critical need to address the ethical implications and societal impacts of agentic AI. As these technologies continue to evolve, their impact on employment and organizational dynamics remains a significant concern. The leaders discussed the importance of ethical guidelines and regulatory oversight to manage these changes responsibly.
The conversation also touched on the importance of strategic planning for the future integration of agentic AI, emphasizing the need for companies to remain adaptable and forward-thinking. This involves not only keeping abreast of technological developments but also preparing the workforce for changes in the types of skills and roles that will be required in an AI-enhanced future.
5. Impact Measurement and Value Realization
Finally, establishing clear metrics for success is crucial for assessing the impact of agentic AI projects and aligning them with business objectives. Participants noted that while agentic AI can streamline operations and enhance decision-making processes, the primary metrics for evaluating its success should include improvements in operational efficiency and customer satisfaction, rather than just cost savings.
This rich discussion provided valuable insights into the strategic value, practical applications, and integration challenges of agentic AI. As these systems become more sophisticated and widespread, the insights from this roundtable will undoubtedly help shape strategic decisions and implementation approaches in various industries.
IIA virtual roundtables are exclusive, invite-only discussions designed to promote peer-to-peer exchange on pressing challenges in the data and analytics community. Seats are limited and reserved for C-suite data and analytics leaders or equivalent at mid- to large-sized enterprises. Conversations are geared toward non-digital native companies. If you meet these criteria, contact us for more information.