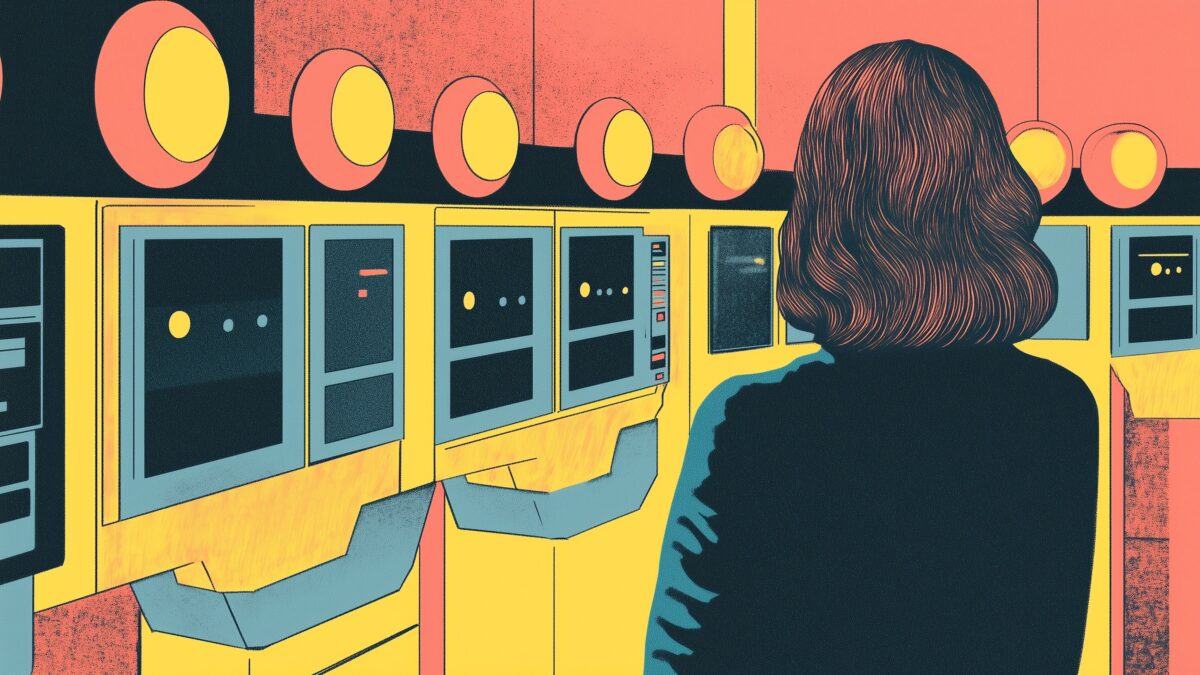
As I think about our year ahead, I am reminded of my start in healthcare nearly ten years ago. Having led data, analytics and AI initiatives at leading organizations across numerous competitive industries, nothing could have prepared me for healthcare.
Much like being dropped off on the shores of a brave new world, it was exciting—new adventures, aspirations and opportunities to help people live healthier lives. At the same time, it was complex, unfamiliar and somewhat terrifying—new languages and acronyms, cultures, organizational dynamics and politics, partnerships, regulations, bureaucracy, business models, tools and technology, and so on. Fortunately, many people came forward to help me on my journey, and I eventually found my place and thrived, for which I will be eternally grateful.
Fast-forward to today. We can only wish that the healthcare ecosystem was so simple—as we discussed last month, it is rapidly becoming more complex and chaotic by the day. The stakes couldn’t be higher for the healthcare systems and the patients they care for. Fortunately, the fundamentals that helped us achieve success over the past decade leveraging data, analytics and AI are the same ones that will help us navigate this rapidly evolving landscape. Many of these topics have been covered in this blog series.
It isn’t about having data or cool new technology (analytics, AI, chatbots)—though we gave AI much of our attention last year. The focus is on innovating with data at scale, using these technologies to shift from reactive to proactive workflows, automate manual processes, improve health equity, reimagine care delivery, enhance employee and patient experiences, and accelerate research.
This involves moving away from transactional relationships between business, clinical and technology, and partnering closely on agile, self-organizing delivery teams. It involves shifting away from a plan-and-implement to a do-and-adapt mental model for delivery—delivering incrementally and often demonstrating value along the way. More importantly, it involves doing this at scale, where everyone in the enterprise participates in data innovation leveraging data, analytics, and AI.
Data fluency is the catalyst that can be either the headwind or a tailwind on this journey. Let’s look at a framework you can use to understand your data fluency and guide your journey to becoming a data-driven organization. Here is a personal story on data fluency’s evolution and impact at a data-driven organization that transformed the retail landscape.
Data Aspiring to Data Fluent Story
An early experience in the shift from data aspiring to fluent was at Amazon in the late 1990s. E-commerce websites were a new canvas that everyone was trying to figure out how to use. Website design was more art than science, and website changes were based on qualitative and anecdotal information. Decisions were primarily based on who could make the best argument or pitch—sometimes, it got quite political. It was like trying to agree on what was good art, based more on public opinion than facts. Sound familiar? Not unlike our experiences in healthcare.
Unsatisfied with the status quo, a few statisticians and engineers came together to develop a more quantifiable, consistent, scalable, and accessible way to assess website changes. They implemented an A/B test capability with standard measures and reports that anyone could use to quantitatively determine the impact of website changes. This was a big step forward for data fluency. However, more needed to be done.
Though there were standard, trusted measures, and people had learned how to apply basic statistical concepts, some people used this capability to argue (wrongly) that their website change was successful. A memorable example was when a change caused online orders to drop dramatically. This would typically be a clear signal to stop the test ASAP. Losing money is generally not good. However, the test owner claimed the change was successful because browse activity increased. They argued convincingly (and wrongly) that this created long-term value not captured by A/B testing. You have to love creative-thinking people.
To address this gap, the A/B test team formalized the process of designing the experiment before running it, setting objectives and measures of success from the onset. This made it very easy to determine (once statistical significance was achieved) whether the test was a success or failure, significantly reducing the time and friction involved in shutting down an experiment or moving it to production.
As data fluency matured, it nearly eliminated politics and reduced decision-making time, improved customer experience, formalized the art and science of website design, and democratized access to A/B testing, allowing anyone with an idea to participate in evolving the website and, subsequently, the business.
This was one function. Imagine an organization repeating this dozens of times across all enterprise functions. The result is a highly data-fluent enterprise that can navigate, thrive and lead in any storm or chaos, setting a new standard for others to follow.
We have begun this journey in healthcare. For example, some healthcare organizations have even implemented their version of A/B testing to accelerate quality improvement efforts — learning more in months than could be done in a year. Though most are realizing improvements in efficiency, quality of care and workforce experience, the adoption rate and scope of impact vary greatly — in some cases, leveraging the same tools and delivery processes, and in all cases, comprised of smart, capable and motivated people. Data fluency is at the heart of the difference.
Let’s examine data fluency, its meaning and building blocks in greater depth. This should give you food for thought about how to accelerate your data innovation journey by improving data fluency.
Data Fluency Defined
In defining data fluency, it is helpful to understand the virtuous learning cycle. This cycle is an iterative process where each cycle of decision, then action, and then evaluation builds on previous experiences to drive continuous improvement, growth, and innovation. This cycle’s speed, scale, and effectiveness directly relate to an organization’s data fluency. See Caption 1 below.
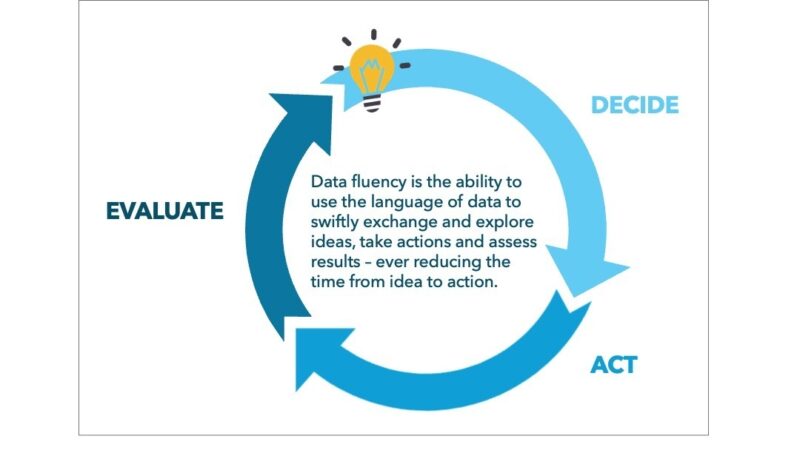
Data fluency is the ability to use the language of data to swiftly exchange and explore ideas, take action, and evaluate results—ever reducing the time from idea to action. As data fluency grows, the quality and time required to decide improves, accelerating the lifecycle. As more automation is introduced, the time from decision to action is nearly instantaneous. However, this requires a high level of data fluency. Let’s examine the building blocks of data fluency.
Data Fluency Building Blocks
Data-fluent organizations demonstrate high maturity across the four building blocks that power the learning cycle (see Caption 2 below). These building blocks are data consumer, data producer, data community, and data platform.
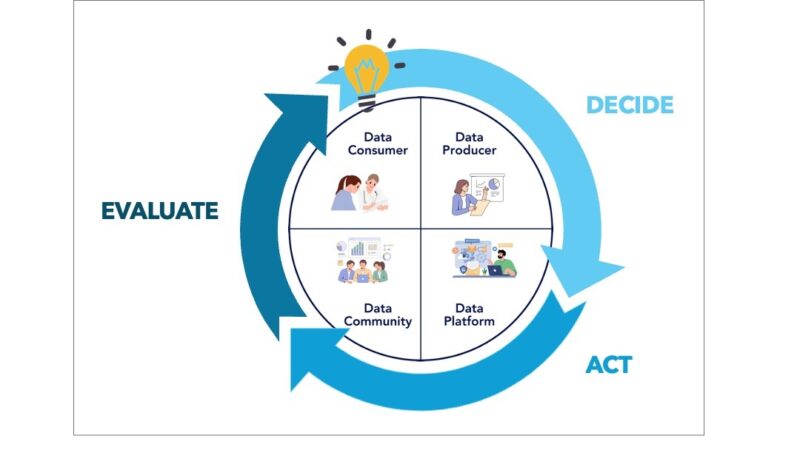
Data Consumer: The individuals and teams using data to inform decisions, design interventions, and continuously improve outcomes. Highly fluent consumers do more than consume static reports—they explore data, identify patterns, ask deeper questions, and provide producers feedback to improve the data’s quality and utility. We discussed a framework empowering data consumers as producers in this blog series titled “Self-Service Analytics.”
As data fluency grows, applications become data consumers in addition to humans. These applications integrate decisions into workflows and sometimes fully automate the decision, action and evaluation cycle. Examples include noting the risk of organ failure in a patient’s medical record or bedside monitor, diagnosing images, coding payer claims, recommending more resources to support unexpected spikes in census, and reordering supplies. In the article “Intelligent Automation,” we discussed a framework for powering this automation.
Data Producer: People responsible for preparing, curating and delivering data for consumption. They ensure data is accurate, accessible and structured to meet organizational needs. Fluent data producers understand the business context of the data and work closely with consumers to align on goals and definitions. In the article “Implementing the Pulse Framework,” we discussed a framework for engaging and aligning data consumers and producers, creating value and increasing their data fluency along the way.
In addition to data producers (data analysts and data scientists) who excel at telling stories with data and teaching this skill to data consumers, there is a class of data producers who deliver self-service tools and automation (data and software engineers), as described above. Over time, data engineers automate (bit by bit) what data analysts and data scientists do, and software engineers automate what data engineers do, freeing up time to work on more complex and high-value opportunities. The ultimate goal is to create greater agency and automation for data consumers—improving the speed and quality of the learning cycle.
Data Community: A culture and operating model that fosters collaboration, knowledge-sharing, and collective learning around data. A strong data community has common purpose and values, and ensures that data standards, definitions, and best practices are shared across the organization, reducing silos and driving consistency and trust. We discussed a framework for such a data community in “Operating Like a Start-Up” and “Thinking Like an Entrepreneur.”
Data Platform: The underlying infrastructure, tools and technologies that store, process and distribute data. A mature data platform provides seamless access to trusted data, supports advanced analytics and enables automation and scaling of data-driven initiatives. We discussed a framework for such a data platform in “Every Journey Needs a Platform” and “Voyaging to the Cloud,” Part 1 and Part 2.
Let’s use these building blocks to assess the data fluency for several real-world example information ecosystems and how that fluency translates to enterprise value.
Low Data Fluency Information Ecosystem
The caption below is an example of an information ecosystem with low data fluency—no doubt that is clear from the illustration. Evaluating this based on the four data fluency building blocks helps to highlight the challenges, risks, and opportunities.
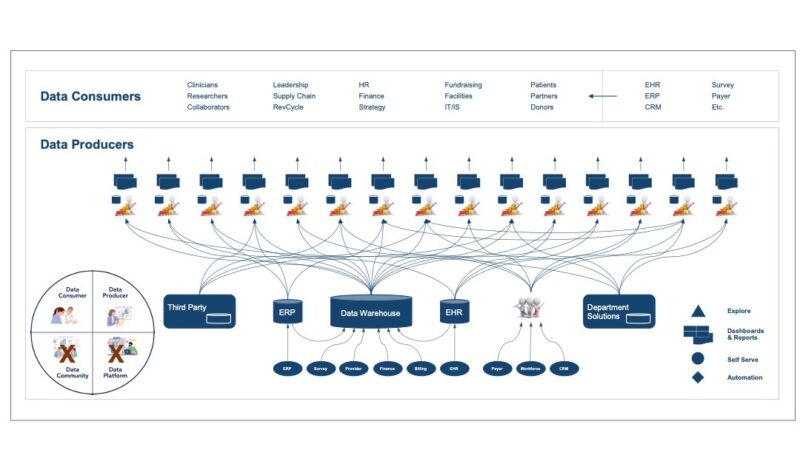
In this example, the data consumer and producer (for those departments that have them) are happy and effective in using data to explore ideas, answer questions, understand problems and act on decisions. That said, the decision is only as good as the data. So, they may be making more poor decisions faster.
Depending on their fluency, they may or may not be effective in evaluating the results of actions to guide future decisions. They also probably struggle to access the data they need, and debate the quality of their dimensions and measures with other departments. There is also massive duplication and waste across the information ecosystem.
In addition, siloed solutions likely exist that started as prototypes or proof of concepts that were never fully implemented. These solutions have limited value because they can’t be leveraged more broadly. They also result in a patchwork solution that doesn’t scale and is expensive to maintain. Neither data consumers nor producers are happy and likely place blame on each other or the data platform.
The data community is non-existent. This means some departments have access to data while other departments don’t. There is no ownership of data, dimensions and measures, resulting in no trusted source of truth. This results in the organization spending an exorbitant amount of time debating the quality of data and how it is used rather than working together to understand the story the data is telling them about the health of the business and areas for improvement and growth. There is also no opportunity to build on shared learning because everyone works in isolation.
The data platform sits on an island. A data warehouse has been created with a subset of the data needed, and some capabilities for ad hoc analysis, reports and dashboards. The best case scenario for data consumers is that their department has a data producer and only relies on the data platform to access data. In the worst-case scenario, the data producers are aligned with the data platform, and all departments compete for access to data producers and the data platform. This results in a transaction relationship with data producers and data platforms.
As you can see, the organization's lack of data fluency across all building blocks has become a significant headwind to progress. The information ecosystem has become complex, wasteful, disconnected and competitive (in a bad way). As entropy sets in, the enterprise becomes incapable of adapting to the rapid change and chaos of the healthcare landscape. The information ecosystem has become a liability rather than an asset.
High Data Fluency Information Ecosystem
In the previous example, the information ecosystem is not only ineffective; it has become a liability to the organization, causing more damage than good over time. Now, let’s contrast this with a highly fluent, healthy information ecosystem.
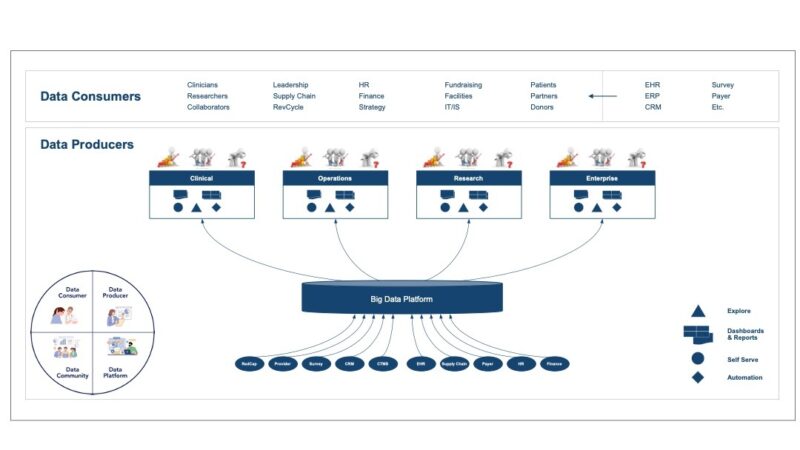
In this example, the data consumers and producers work in partnership on small, agile, multidisciplinary teams that are aligned and embedded in a project or functional area (business, clinical or research). Data Consumers have the skills and tools to access, analyze, and act on data effectively. They understand data quality parameters, and can confidently use data to drive decisions, take action, and evaluate results. Data producers serve as enablers and partners rather than bottlenecks, focusing on creating scalable, reliable data products that serve multiple use cases across the organization.
The collaboration between consumers and producers is characterized by mutual understanding and shared goals. Producers actively seek feedback from consumers to improve data products, while consumers understand the complexities of data management and contribute to data quality improvements. This partnership ensures that data solutions are practical and sustainable, leading to better, data-driven decisions across the organization.
The data community thrives as a vibrant, interconnected network that spans departments and roles. It is the foundation for knowledge sharing, best practices and collective learning. Data stewards take ownership of key data domains, ensuring consistent definitions and quality standards across the organization. Regular community events, forums and working groups facilitate collaboration and prevent silos from forming. Examples include town halls, demo days and hackathons where the community comes together to share and grow their shared experiences.
The community also maintains clear governance frameworks and automation that promote loose coupling and balance innovation with control, always striving to give data consumers and producers more agency. This creates a culture where data is treated as a valuable enterprise asset rather than departmental property. Cross-functional teams regularly come together to solve complex problems, share insights, and build upon each other's successes. This collaborative environment accelerates learning and helps the organization adapt quickly to new challenges and opportunities.
The data platform is a central nervous system for the organization's information ecosystem. It provides a robust, scalable foundation supporting standardized reporting and innovative analytics use cases. The platform is designed with technical excellence and user experience in mind, making it easy for data consumers to access the data they need while ensuring security and governance requirements are met.
Key features include self-service capabilities, automated data quality checks and clear data lineage documentation. The platform team works closely with data producers and consumers to continuously improve capabilities and address emerging needs. Rather than being a static repository, the platform evolves alongside the organization's data maturity, incorporating new technologies and capabilities as needed.
The combined result is an information ecosystem that operates as a true force multiplier for the organization. Data flows seamlessly between systems and teams, enabling rapid innovation and decision-making. The community's collective intelligence helps identify and solve problems quickly, while the robust platform ensures sustainable and scalable solutions. This creates a virtuous cycle where improved data fluency leads to better outcomes, which in turn drives greater investment and commitment to data excellence across the organization.
In contrast to the liability created by low data fluency, this ecosystem becomes a strategic asset that helps the organization thrive in an increasingly complex and data-driven healthcare landscape. The combination of skilled people, a strong community and capable technology creates resilience and adaptability, essential for long-term success.
Final Thoughts
As we navigate an increasingly complex healthcare landscape, data fluency has emerged as the crucial differentiator between organizations that merely survive and those that truly thrive. Much like my journey into healthcare a decade ago required learning new languages and ways of working, organizations today must embrace the language of data to evolve and innovate effectively.
The path from low to high data fluency is not just about implementing new tools or hiring more analysts—it requires fundamental shifts in how we think about and work with data. When we successfully mature the four building blocks of data fluency—empowered data consumers, skilled data producers, a vibrant data community and a robust data platform—we create an information ecosystem that serves as a strategic asset rather than a liability.
This journey to data fluency may seem daunting, but it is both necessary and achievable. As we've seen through examples ranging from Amazon's early days to modern healthcare organizations, the rewards of increased data fluency are transformative: faster decision-making, improved outcomes and efficiencies, greater innovation and enhanced ability to adapt to change. In an era where healthcare complexity continues to grow exponentially, data fluency isn't just an aspiration—it's an imperative for organizations that wish to lead the way in delivering better care and outcomes for the patients they serve.
The question is no longer whether to invest in data fluency but how quickly we can evolve our organizations to embrace this new language of innovation and transformation. The future of healthcare belongs to those who can speak it fluently.
Next month, we will explore steps you can take to evolve data fluency within your organization regardless of your starting point. In the meantime, I would recommend a great book on data fluency, Data Fluency: Empowering Your Organization with Effective Data Communication. It is an older book, but its concepts and lessons are timeless.