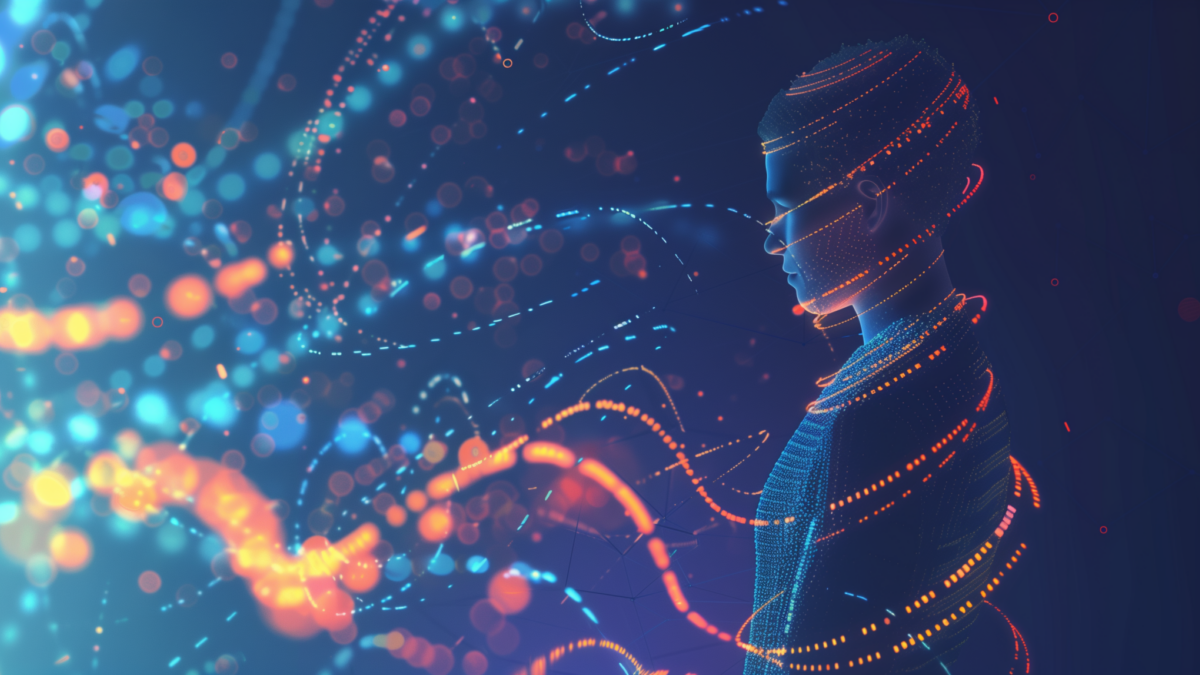
We have come a long way in discussing why and how to accelerate your data innovation journey. We discussed the rapidly evolving healthcare landscape and how the digitization of the consumer experience (and data) is fueling business initiatives to improve access and outcomes, accelerate research, enrich patient and workforce experience and streamline operations.
To ride the wave, we discussed the need to create a data-driven community. This community will innovate with data, embrace uncertainty, and adapt and thrive in the rapidly evolving business and technology landscape.
To this end, we discussed how a federated operating model and entrepreneurial mindset shape a community that can navigate this terrain. We examined the power of agency and leading intrinsically to promote innovation, productivity and employee engagement. We discussed the engine that powers the community—the data and analytics platform.
This led to discussions on leveraging the platform to demonstrate value by implementing a dashboard framework (Pulse) and self-service analytics to optimize patient flow, promote quality improvements, and streamline decision-making. We discussed leveraging these capabilities to shift focus from reactive to proactive, promote greater automation and advance health equity. If you’ve missed some of this discussion or want to start at the beginning, see sidebar.
You may be wondering how well this ecosystem supports the adoption of AI—especially with the introduction of Large Language Models (LLMs). I believe the answer is…very well.
The Evolution
The information ecosystem we have discussed to accelerate your data innovation journey evolved from organizations that have led the transformation in industries leveraging earlier generations of AI. Marco Iansiti and Karim R. Lakhani do an exceptional job telling this story in their book Competing in the Age of AI: Strategy and Leadership When Algorithms and Networks Run the World.
Here is an excerpt from a recent a16z (Andreessen Horowitz) article that provides context on the impact of an earlier generation of AI.
We can look to the introduction of the internet in the 1990s to see what happened in the last technological revolution. Pre-internet—in 1990—the biggest companies at the time were largely in non-tech industries like oil and gas (Exxon Mobil), pharma (Merck), CPG (Coca-Cola), auto (GM), and telecom (AT&T). While some small public companies in the 1990s like Apple and Microsoft embraced the internet and 500x-ed to become the biggest companies in the world, the vast majority of value accrued to startups founded as the internet was taking off. Today these startups—Alphabet, Meta, Amazon—have knocked all of the aforementioned biggest companies of 1990 off the top 10 list.
In addition to the seismic impact, a defining characteristic was the digitization of the consumer experience and the use of advanced analytics and AI to deliver a personalized experience for consumers and automate business functions (supply chain, merchandising, marketing, etc.).
Granted, the methods and models lacked the sophistication and precision of those used today. They also required much more time, effort, and expertise to build and use. However, they had a few core things in common—they needed a lot of data and computing power, the output was probabilistic and predictive, and they were capable of being used for good and evil.
So, I view LLMs and recent AI advances as more of an evolution than a revolution, albeit a significant step change. Let’s dig in and take a closer look.
The Coming Wave
As discussed, leading organizations have used predictive models since the 90s to target consumer communications, generate product recommendations, replenish inventories, purchase stocks, and so on, and, in the process, redefine industries.
More recently, in healthcare, we’ve been using models to optimize patient flow, replenish supplies, identify revenue cycle leakage, validate ICD-10 coding, plan staffing, and assess patient risk, among other things. We have also seen the growing adoption of more sophisticated models in radiology diagnosis and genomic research.
We have also experienced many of the issues we are discussing today around LLMs. Bad actors doing bad things, or more often, people with good intentions, doing bad things. Some of the things I saw in the early days of product recommendations still make me cringe. The book by Cathy O'Neil is a must-read of cautionary tales, Weapons of Math Destruction: How Big Data Increases Inequality and Threatens Democracy.
So, the landscape is familiar, and for more mature organizations, steps have been taken to mitigate risk and leverage capabilities responsibly—governance, operating models, policies, practices. I believe a BIG difference with LLMs is the scale. This will require even data-savvy organizations to evaluate the impact on their ecosystem and take steps to adapt.
For example, AI is no longer the domain of data scientists and people with deep expertise in using AI. With LLMs, we are democratizing access to AI. This is reminiscent of the days of the internet and mobile, where access to information and computing went from a hundred million to billions of people in a decade.
We are now doing the same thing with knowledge. Just imagine. In a decade, we will go from hundreds of thousands of people building AI solutions to hundreds of millions—data innovation on a massive scale. OpenAI recently opened its GPT marketplace. Already, there are over three million apps.
At a global level, people much smarter than me are addressing how to guide the social, economic, and political impact of this change. Mustafa Suleyman has a wonderful book that covers this topic: The Coming Wave: Technology, Power, and the Twenty-first Century's Greatest Dilemma. He provides a balanced view of the impact and offers guidance on containment steps.
Fortunately, our scale is more manageable within companies and healthcare systems. If you have formed the information ecosystem discussed in this series, you are well on your way to leveraging these new AI advances responsibly without stifling innovation.
Let’s examine ways to leverage the information ecosystem and where we need to think differently than in the past.
Frameworks Over Controls
With all the hype and fear around AI and LLMs, people are scrambling to decide what to do and how to control it so bad things don’t happen. Some organizations have gone as far as to block all access to ChatGPT—falsely thinking this will provide protection.
However, people always figure a way around controls, and if they don’t, this leaves good things undone that could improve or save lives. A lose-lose situation. This becomes an environment without agency, transparency or trust.
Though intentions are good, my experience is that controls rarely work. Granted, sometimes you don’t have a choice. However, controls should be a last resort. There are better ways to minimize the frequency and impact of adverse outcomes that promote transparency, innovation and agency within the organization.
To this end, it is essential to implement frameworks that guide decisions and trust people to act responsibly to leverage and evolve the efficacy of these frameworks. This is at the core of a healthy information ecosystem, and is achievable with the right people, culture and leadership.
In the operating model we discussed in this series, delivery teams are central to this governance. They own and manage all data assets and how they are used. They are also central to these frameworks' development, use and evolution.
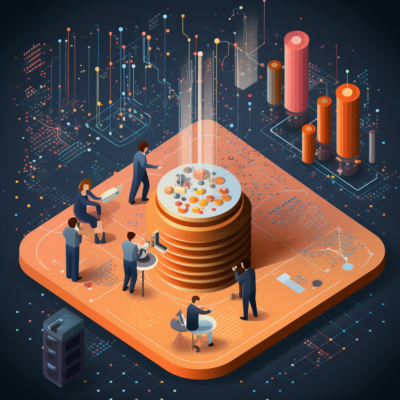
Creating a Data Strategy eBook
The growth in the demand for better decision-making with data means that a comprehensive data strategy is no longer a nice to have. You need a data strategy. It needs to address questions about how to improve the availability, timeliness and quality of data, in that order of priority.
Download this eBook now to get actionable recommendations, including context and vision for data strategy must reflect business needs, specific objectives must include scope, rationale, and benefits, how to develop a framework to evaluate where you are now and qualitatively assess your end state, and more!
Level Up Data Fidelity
Data fidelity may be the most critical issue to address over the coming years. Even if you believe your data quality is excellent, and let’s say it is, it probably isn’t good enough for future AI use cases. Today, we rely on human intervention to make judgments to compensate for gaps in the data and, often, identify quality issues—probably more than we realize.
With the new models, the data volumes and complexity make it impossible for humans to intervene. Data gaps are no longer acceptable. Accuracy and completeness need to be as close to perfect as possible. Also, monitoring tools are required to provide ongoing validation of the data and models.
To give a sense of the new bar for data quality, let’s look at a real-life example in travel. For this company, the SLA for booking data, when comparing the difference from the source booking systems to the analytics and AI platform, is less than a thousand dollars per billion dollars booked. To accomplish this, they built a tool called Guardian that monitors data in their platform 24/7. This is the new bar.
Scale Data Ethics
If you already use predictive analytics, you probably have governance to ensure your models are used ethically. The challenge you will have is scaling your governance.
The new AI tools (e.g., ChatGPT) are much more accessible. So, rather than a few big project requests, you will have hundreds of smaller requests to assess. To avoid stifling innovation, you will need a lightweight process to vet requests that need peer review and those that don’t.
One approach is to use a checklist to score projects to assess risk. Those that score high enough undergo peer review. Seattle Children’s Hospital and Carnegie Mellon partnered a few years back to do pioneering work in this area. I don’t believe this addresses the evolving need entirely, but it is a good starting point.
All AI projects should be logged, piloted, and tracked. A journal should be created to record outcomes and lessons learned.
In our next piece, we will discuss scaling data ethics (and data fidelity) in more detail.
Bullets vs Cannonballs
Jim Collins introduced the idea of bullets vs cannonballs in his book, Great by Choice: Uncertainty, Chaos, and Luck—Why Some Thrive Despite Them All. The idea is simple. Rather than pick a few things and go big (cannonballs), pick many things and go small (bullets).
He observed that during uncertain times, successful companies focus on bullets rather than cannonballs to reduce risk and cost, accelerate delivery, and improve their odds of success. Or, as the proverb goes, don’t put all your eggs in one basket.
Companies that lead in leveraging AI have adopted a similar philosophy. Rather than debate where to invest, they do a bunch of small pilots that are budgeted and time-boxed. For those things that fail, they fail quickly and record lessons learned. For those that succeed, they double down and scale the solution.
This is a great way to reduce cost and risk, engage the broader organization, and demonstrate value quickly. This learn-by-doing approach is also a great way to accelerate the growth of data fluency in an organization.
Each pilot should have a delivery team. This team will ensure ownership and alignment with business, clinical, and technology; it will ensure the capacity to support the pilot and, more importantly, the capability if the pilot is successful. It will also ensure frameworks are appropriately leveraged.
Prepare to Scale Massively
If you aren’t there already, the data volumes managed and used will be measured in tens if not hundreds of petabytes. Existing data will become more granular. A good example is biometric data. Instead of point-in-time measures, data will be streamed continuously from the bedside. There will also be new IoT data and other external data sources to integrate, as well as genomic data.
As if that weren’t enough, the new AI tools will not only leverage data but produce massive amounts of unstructured data. Ambient technology is getting a lot of attention these days. Just imagine how much data will be generated when every conversation is digitized.
So, you will need a highly distributed computing environment where data is captured near real-time and data streaming is used to deliver real-time predictive analytics. If you have the scale as an organization, it might make sense to implement your own technology stack. The cloud (GCP, AWS, Azure) will be more scalable and cost-effective for most.
You will not only need to store data at a massive scale, you will need to use this data at a massive scale. So, affordable scaling is essential.
Another consideration is that change will be continuous in this environment. So, automating user access, data stewardship, and deployment will be essential to the ecosystem's scalability and stability.
We will dive into this area in our piece in April.
Formalize Training and Education
Delivery teams are vital to developing the organization's data fluency. However, they aren’t enough. Investing in a broad education program around data fluency is essential.
If you already have such a program, congratulations! All you need to do is expand your curriculum to discuss how things are different and the same when leveraging new predictive AI tools.
If you don’t, I highly recommend you invest in it. You may have been able to get away without it in the past because it was the domain of data scientists and data engineers with a high level of AI proficiency. That will no longer be the case with these new methods and technology.
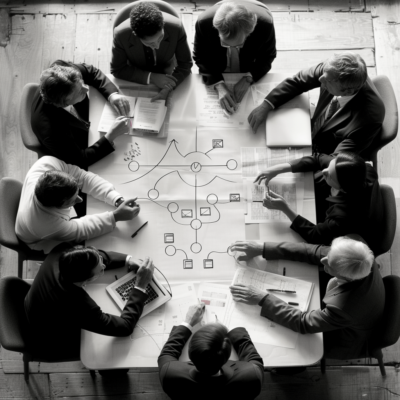
Upskilling Your Organization Webinar
This panel discussion, moderated by IIA's Bill Franks and featuring leaders from Northwestern Mutual, Travelers Insurance, and Data Society, provided different viewpoints, insights, and success stories on upskilling the enterprise for data, analytics and AI. The session explored several dimensions of successful upskilling programs, from leveraging university partners to advanced training to showcasing innovation and rewarding employees.
Think Creatively
As you explore solutions to the problems discussed, thinking creatively about using LLMs will be essential. For example, you may use an LLM to validate another. A simple example is something I’ve been doing recently. Whenever I use ChatGPT, I have two sessions—one to answer my question and the other to validate the answer.
Also, LLMs can generate code and automation for data acquisition and validation. Also, some organizations are beginning to explore leveraging LLMs as an alternative to accessing the data platform. So, rather than looking at a dashboard to understand the health of your business, you can ask ChatGPT. It's still early days, but seeing where something like it can go is exciting. Will we create a HAL or Computer?
These are just a few ideas. No doubt there are others if you think creatively.
In Closing
LLMs are a game changer on the scale of the internet and mobile phones combined, and the adoption is happening at a pace never seen by any technology. Though there are thorny and complex problems to solve when looking globally, if we focus on our health systems, navigating this terrain is much more manageable.
The information ecosystem discussed in this series is well-positioned to support the next generation of AI. However, there are important considerations as you evolve your information ecosystem. We must reset our thinking around data quality, data ethics and solution delivery. We also need new capabilities and religion around automation. Lastly, we will need a data platform that can start small and scale massively over time—and affordably.
In our next two pieces, we will dive deeper into evolving data fidelity and ethics tools and practices to support this next generation of AI. Also, we will dive deeper into building a data platform where you can start small and scale massively over time.