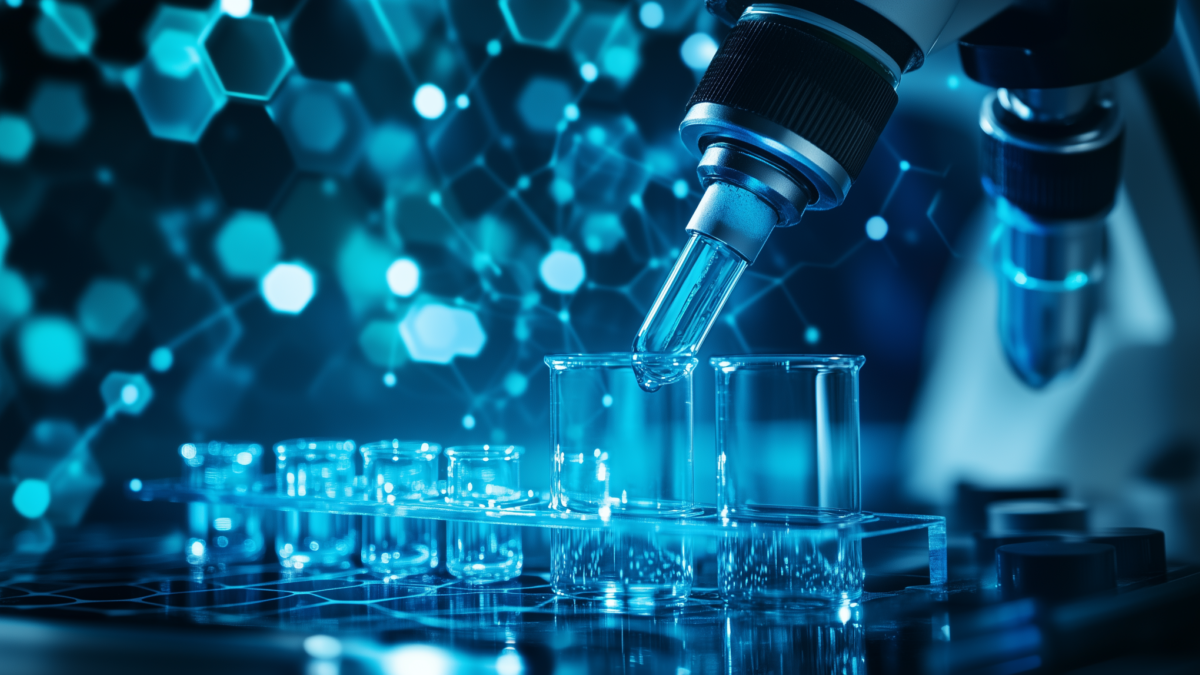
We've covered significant ground in accelerating our data innovation journey, leveraging analytics and AI at scale to transform the care ecosystem. This evolution can be symbolized by an infinity loop, where research on one side continuously fuels care delivery on the other, with each cycle enhancing care delivery and improving lives.
So far, our focus has been on the care delivery component of the ecosystem of care. In this article, Dr. Philip Hagedorn, chief research information officer at Cincinnati Children’s, shares how modern research is turbocharging this journey and revolutionizing healthcare.
I want to express gratitude to Phil for sharing his wealth of knowedge and experience. This is an excellent piece, and I am sure you will enjoy it. Let’s get started!
—Ryan Sousa
Introduction
The line between cutting-edge research and everyday patient care is blurring in today's healthcare landscape. Biomedical research informatics—the increasingly common use of biomedical data in combination with advanced computing—is playing a crucial role in this evolution. But what does this mean for hospitals, researchers, and most importantly, patients?
The Research Spectrum: From Lab Bench to Bedside
Biomedical research spans a wide range of activities aimed at generating new knowledge for the benefit of patients and families suffering from all kinds of maladies. The National Center for Advancing Translational Sciences uses a diagram to represent the interconnected nature of these activities with a patient appropriately centered in the middle as illustrated below.
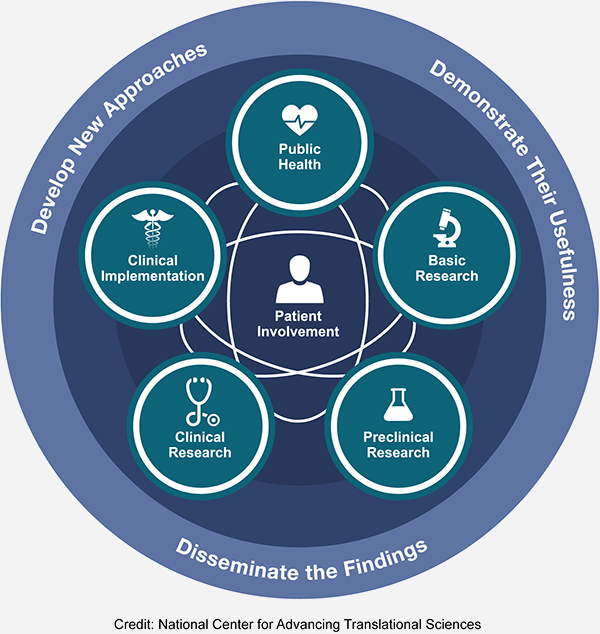
Broadly speaking, these activities can be defined as follows:
Basic Science: Think of scientists peering through microscopes at cells or using molecules to alter cellular behavior. This is where the scientific community uncovers the fundamental workings of biology.
Pre-Clinical and Clinical Research: These activities serve as a bridge, turning basic science discoveries into potential treatments for patients. Scientists working in these domains ask questions like “Is this drug safe?” or “Does this treatment cure disease?”
Clinical Implementation Research: There’s a difference between a scientist knowing a treatment works and ensuring that treatment is used consistently and reliably in everyday healthcare settings. The latter boils down to altering the behavior of healthcare providers and healthcare systems. Implementation research helps spread and scale from “this drug works” to “this drug is prescribed for and taken by patients who stand to benefit.”
Public Health Research: These activities zoom out from individual patients to understand how interventions impact populations of patients.
Each type of research described above generates valuable data, but traditionally, these data often remain isolated by domain or project. Even when forethought is invested in generating integration-ready, reusable data, these data suffer in institutional silos for lack of tools and methods to render them discoverable and useable by other members of the research community.
The Current Challenge: Research as a Cottage Industry
Prior to the Industrial Revolution, the manufacture of goods relied upon individuals, families, or small groups of laborers working in an isolated, unharmonized fashion. This “cottage industry” model created goods in bespoke environments and without a standard approach to tools, method, or workflow. This meant that output from one “cottage” was often incompatible with that from another. The Industrial Revolution brought fit-for-purpose environments, standard tools, methods and output that allowed for compatibility and scalability. The conduct of research at many institutions still resembles the “cottage industry” approach and results in many of the same shortcomings:
- Data trapped in individual labs
- Inconsistent tools and methods across departments
- Difficulty in combining data for big-picture insights
Learning from Industry: The Power of Shared Platforms
Industry partners in biotech and the pharmaceutical industry have shown us a different approach:
- Standardized data systems across the organization
- Clear rules for data sharing and security
- Collaboration tools that connect researchers across projects
The result? Faster discoveries and more efficient use of resources.
Bringing This Approach to Academic Medical Centers
How can hospitals and universities adopt these practices?
- Build a Common Data Foundation: Implement shared systems for storing and analyzing research data. There are already maturing efforts, like ODHSI’s OMOP in common clinical data modeling, which is gaining steam. These efforts need to expand beyond clinical data to biomedical data.
- Establish Clear Data Rules: Combine tools, workflows and policies that make data sharing safe and easy. This includes rendering research data discoverable by potential collaborators and easily re-usable through curation and sharing efforts.
- Foster a Collaborative Culture: Encourage researchers to collaborate and work differently across boundaries with the reuse of research data as a focal point.
- Invest in Enterprise Solutions: Leverage the institution's resources to provide powerful, scalable research tools. Having a data platform shared across hospital and research is foundational. Let’s dig in here a bit.
Breaking Down the Hospital-Research Divide
Here's a key insight: we need to stop thinking of "hospital data" and "research data" as separate entities. Instead, imagine a unified data ecosystem that serves both clinical care and research needs. This unified ecosystem will serve the efforts to create a true “learning health system” that can drive discovery of new therapies, test their safety and efficacy, implement these new interventions in delivery systems and understand their impact at scale.
Real-World Impact: Research Informatics in Action
The real-world impact of these improvements is not the plotline in a science-fiction novel. They are happening today in a health system near you. Instead of a patient receiving one-size-fits-all chemotherapy, a genetic analysis of their tumor led their healthcare team to select targeted therapy. A radiologist uses a machine learning model, trained on vast image datasets, to identify subtle signs of disease in an imaging study. Global data-sharing networks are now connecting patients, researchers, and clinicians to co-produce interventions and improve outcomes for entire populations suffering from disease.
The Road Ahead: Challenges and Opportunities
While the potential of an integrated research informatics ecosystem is enormous, there are many hurdles to overcome, including considerations of patient confidentiality and consent, cross-institutional interoperability and agreements, and, finally, a fundamental shift amongst the research community toward openness and collaboration.
Despite these challenges, the benefits make the effort worthwhile. Institutions that successfully integrate research informatics will be better positioned to recruit world-class talent attracted by a collaborative environment, and discover and drive implementation of cutting edge treatments for patients and families seeking best-in-class care.
Conclusion: A New Era of Data-Driven Healthcare
The future of healthcare lies in breaking down the barriers between research and clinical practice. By embracing modern research informatics, we can create a seamless flow of knowledge—from laboratory discoveries to real-world patient care.
For patients, this means access to ground-breaking, personalized treatments and care. For healthcare providers, it offers the tools to make more informed and impactful decisions. Finally, for researchers, it opens up new possibilities to tackle the most pressing medical challenges of our time.
As we move forward, remember that there aren’t separate hospital and research communities. There's one healthcare community, united in the goal of improving lives through science and compassionate care.