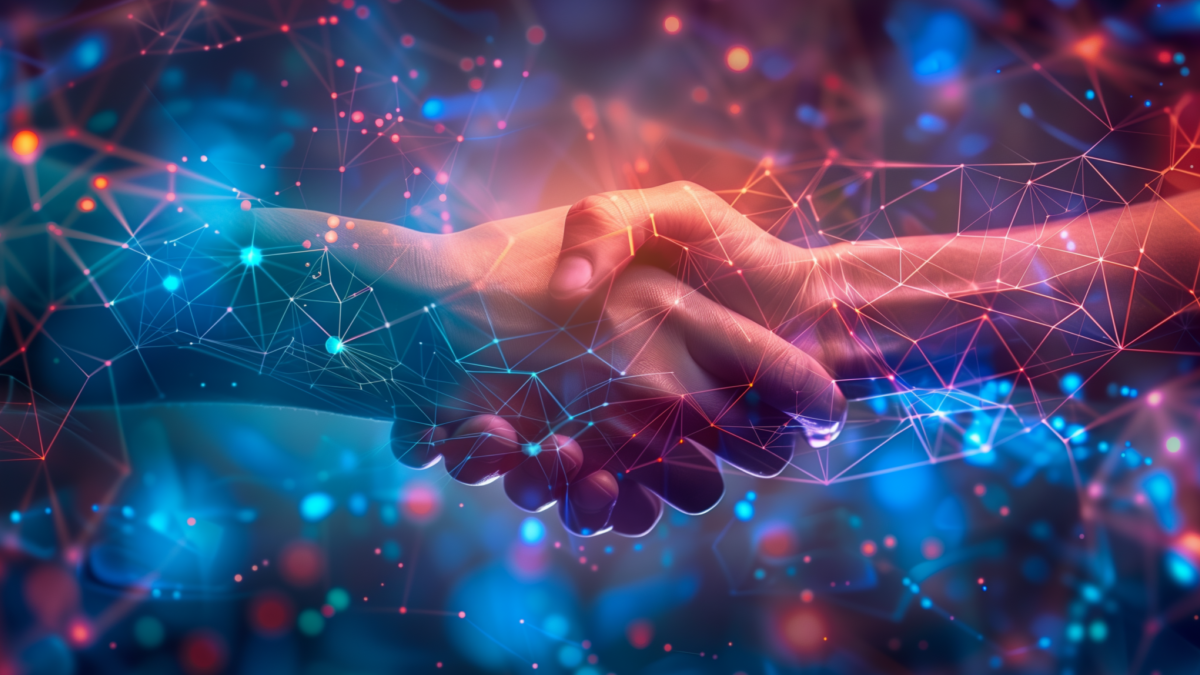
In our series, "Accelerating Your Data Innovation Journey," we've delved into healthcare analytics, fostering startup-like teams with an entrepreneurial spirit, creating an enterprise MAP (Maturity Acceleration Plan) to guide your journey, and creating a data, analytics and AI platform. In the last piece, we discussed powering your journey with AI, and how the definition of acceptable data quality and data ethics is changing. In short, what used to be acceptable is no longer acceptable.
Many of these posts have mentioned the need for data quality to ensure reliable analyses and the importance of ethical frameworks to inform the use of the data. In this post, we will describe the importance of ensuring that an ethical framework is at the center of data decisions, discuss in greater detail the role of data quality as a critical requirement for trustworthy analytics and AI, and provide practical guidance for implementing both.
Data Ethics and Quality
We decided to tackle data ethics and data quality together in this post because they are inexorably intertwined, and there is a bit of a chicken-and-egg conundrum. As our healthcare systems have become more complex, they increasingly rely on data to make decisions on patient care, operations and strategy.
For healthcare to be trusted, there is a strong need for transparent decision-making with an ethical framework that guides when and how data will be used. At the same time, our ethical framework requires a deep understanding of the data used and the nature of the decision tied to that data. In our current health ecosystem, data is a crucial asset, data quality is informed by ethical requirements, and our ethical frameworks require data to be meaningful.
If you use predictive analytics or other AI tools today, you probably have an ethical framework for evaluating these. Still, it may be either too informal or too formal for the scale and agility you will likely need in the future. We recommend a balanced approach of having clear frameworks that support a system-wide understanding of the requirements for the use of data, defined responsibilities for making decisions, and an integrated education program for data and AI fluency.
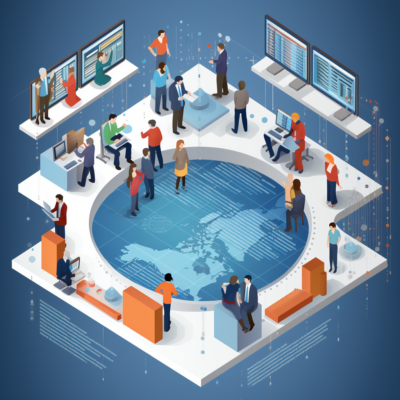
A Comprehensive Guide to Responsible Analytics Governance
Experts at IIA have crafted this complimentary version of our research brief, "A Comprehensive Guide to Responsible Analytics Governance." This brief lays the foundation for operationalizing analytics governance across all business functions in the enterprise.
A Governance Framework for Data Ethics and Quality
In his book AI in Health, Tom Lawry argues that all AI systems should be transparent and accountable, as embodied by four key principles:
- Fairness - AI systems should treat all people fairly.
- Reliability – AI systems should perform reliably and safely.
- Privacy & Security – AI systems should be secure and respect privacy.
- Inclusiveness – AI systems should empower everyone and engage people.
In the age of big data and self-service analytics, these same principles apply to all data usage, including AI-based solutions. Health systems must ensure these principles are incorporated into their data and AI solutions.
This does not necessarily mean standing up completely new governance structures. Each health system should assess whether its existing governance structures can be expanded as simply as possible.
Consider an approach that informs and empowers existing committees to make decisions about using data and AI within their areas of oversight. These committees will need enterprise-wide standards, defined frameworks that they can use and support as they come up to speed.
Workgroups may be needed temporarily to define and socialize these standards and frameworks, but can be disbanded when they are no longer required. All committees should assess if their membership would benefit by including experts in their data and AI solutions. As the organization matures, it may be that a gap is identified in the governance structure and if so, a committee can be created to address Data Governance, including data quality and data ethics.
One area where there needs to be a specific effort made is understanding the possibilities and implications of bias in our data and analytics. There is growing awareness about the risks of bias in our data, and how that can have downstream implications for analyses and algorithms.
In her book, Weapons of Mass Destruction, which we recommended in our last post, Cathy O’Neil states, “many of these models [encode] human prejudice, misunderstanding and bias into the software systems that increasingly [manage] our lives.” Algorithmic biases have the potential to impact large populations due to their repeated applications.
Even with positive intent, data products can exhibit bias based on gender, race, ethnicity, nationality, payor mix, or other sensitive features. These biases can persist even if demographic information is excluded from the model due to various kinds of confounding. Data users and system validators must be trained to interrogate their results and algorithms for bias assertively.
Toolkits, like the one at Seattle Children’s and described in this article, can be very helpful. This checklist approach is familiar to many healthcare providers and ensures that critical questions are consistently addressed. The checklist is developed by health system experts and includes sets of questions on data privacy, consent, bias and transparency. Data project teams can assess and self-score their risks in these areas. Escalation paths can be identified when the answers expose complex data usage. The goal with toolkits and other tools is to democratize access to AI solutions and empower teams to creatively address problems, while surfacing projects with the greatest risk. This approach ensures that teams can move rapidly on lower-risk projects without creating a cumbersome centralized governance structure.
As we’ve described above, evaluating the ethical impacts of data products and analyses requires data of sufficient quality to support the questions being asked and interventions being made. Accordingly, we turn our attention to better describing and understanding data quality.
What Is Data Quality?
The American Health Information Management Association (AHIMA) defines data quality and integrity as “the extent to which healthcare data are complete, accurate, consistent and timely throughout its lifecycle including collection, application (including aggregation), warehousing and analysis.” Adoption of digital health records was intended to fulfill the promise of high data quality for healthcare, but after many EHR implementations, there is a disappointing gap. Eric Topol describes this gap very well in his book Deep Medicine:
Digital record keeping was supposed to make the lives of clinicians much easier. But the widely used versions of electronic records defy simple organization or searchability and counter our ability to grasp key nuggets of data about the person we’re about to see. Just the fact that it takes more than twenty hours to be trained to use electronic healthcare records (EHR) indicates that the complexity of working with them often exceeds that of the patient being assessed. Perhaps even worse than poor searchability is incompleteness. We know there’s much more data and information about a given person than is found in the EHR.
There is a real cost to this data gap: AHIMA has calculated that mismatched patient data is the third leading cause of preventable death and accounts for 35% of denied insurance claims. In addition, there has been a well-documented decline in the patient and provider experience as providers spend more time interacting with the computer and less time with the patient.
Despite this, EHRs and other clinical tools remain poor data sources for rich analytics. There has been a rapidly emerging awareness in healthcare of the need to be much more focused and intentional about data quality and data collection. It is critical that the data meets the standards described above and is “fit for purpose.” Much of the important data about patients is locked in textual notes that are not easily accessed and are challenging to mine.
Data quality investments are even more critical with the coming wave of AI solutions targeted for healthcare. As described in the prior blog post in this series, “data fidelity may be the most critical issue to address over the coming years.”
Practical Guidance
Fortunately, there are best practices and lessons learned from other industries, as well as new innovations, that can be applied to improve quality. We have described an approach throughout this series of using delivery teams who own data assets. This approach works well to enable data stewardship, and data validation and data source improvements, as described below.
It is essential that data stewardship is an integral aspect of their development work. This means that delivery teams will develop and improve data monitoring tools for the entire data pipeline, business rules for data validation, code and data quality reviews, and data quality reporting. Data asset development and data validation must be conjoined activities throughout the delivery team’s activities.
As new data assets are released, improved data monitoring and validation capabilities are released alongside. One bonus of approaching data quality investments in this way is that we can balance out the need for data quality improvements while considering how the data is being used.
Ultimately, data quality improvements are best addressed at the data source, not at the time of reporting. Especially with a newly integrated data source, we find that much of the work is simply understanding and communicating the state of the data being explored.
Our business partners need data quality reporting to understand which processes are followed and how consistently documentation is completed. Our technical teams have a hands-on perspective of the current data realities while our clinical and business users understand and can interpret the data.
The delivery team model is very beneficial for quickly assessing and addressing data challenges. We find that our clinical owners are best positioned to drive improvements at the data source, such as refactoring a clinical workflow or targeted training, leading to rapid improvements in data quality.
But perhaps there is a way to leverage AI and other innovations to improve data collection at source, without further burdening our clinicians with more data entry. Eric Topol, again in Deep Medicine, shares a vision for “using natural-language processing to replace human scribes, reduce costs, and preserve face-to-face patient-doctor communication.”
Natural language processing can take data from notes and audio recordings and better organize it into discrete data fields, which are more valuable for reporting and analytics. Microsoft and Epic, as well as other vendors, have recently released initial solutions that are making this vision a reality, with tools that listen in as a clinician meets with a patient, and captures the notes and discrete data points for review by the clinician later.
The authors in The AI Revolution in Medicine: GPT-4 and Beyond take it a step further and propose using generative AI-based tools like ChatGPT-4 to collect a patient’s history and symptoms prior to meeting with a clinician, thus freeing the clinician up to consider this information without collecting it at all. Of course, building such generative AI models would require robust and representative data sets, which is a reminder of the chicken-and-egg problem we discussed previously. Health systems should proceed thoughtfully, aware of these concerns and risks.
Other Considerations
Data Fluency
Data fluency is mentioned several times above and throughout this series. As described above, there is a need for the entire analytics and AI community to become more knowledgeable about data ethics, data lineage, and data quality. There is a critical need to build data catalogs, data quality dashboards and other meta-data management tools, and keeping them current and integrating them into the user experience. Data ethics and AI training should also be developed and integrated into existing training programs.
Bias
We described above the importance of taking an assertive and somewhat skeptical approach to evaluating any analysis or algorithm. In our work, we have identified the need to independently assess the existence of potential bias based on several demographic features: age, gender, race, ethnicity, language, payor, etc.
It can be challenging to declare an analysis or algorithm bias-free. The existence of bias is not necessarily a showstopper but needs to be considered together with the use of the data and the overall impact on a patient or provider population.
For example, in the case of a no-show algorithm where the intervention is to reach out proactively and confirm a patient’s ability to make an appointment, a small potential for bias in the algorithm might be determined to be acceptable. On the other hand, if the intervention is to overbook appointments, then any bias could negatively impact the experience of patients and providers in already underserved communities, and that should be avoided. Evaluating bias in our data is a non-trivial exercise, complicated by the reality that demographic data in EHRs was originally collected for administrative purposes and suffers from misclassification and missingness.
Data Quality Management
We have shied away from overly technical deep dives into data quality management in this post. But in the classic book, Agile Analytics, Ken Collier provides a great description of best practices for agile business intelligence and data warehousing development. He describes how to make data testing and validation a part of every step in the development process. Part II of this book is a fantastic technical primer for delivery teams and is a strong recommendation.
In Closing
The topic of data ethics and quality in healthcare is a large one that could encompass many books. In this post, we have tried to simply describe the importance of ensuring that an ethical framework is at the center of data decisions, discuss in greater detail the role of data quality as a critical requirement for trustworthy analytics and AI, and provide practical guidance for implementing both. We’ve also hinted at possible deep-dive topics for future posts.
Scaling data ethics and quality governance requires a robust data platform that provides the automation necessary to make this possible at scale – we touch on this in this blog. In the following piece, we will discuss building the data platform and how to create an environment that promotes ethics and quality (and many other things) at scale without breaking the bank.