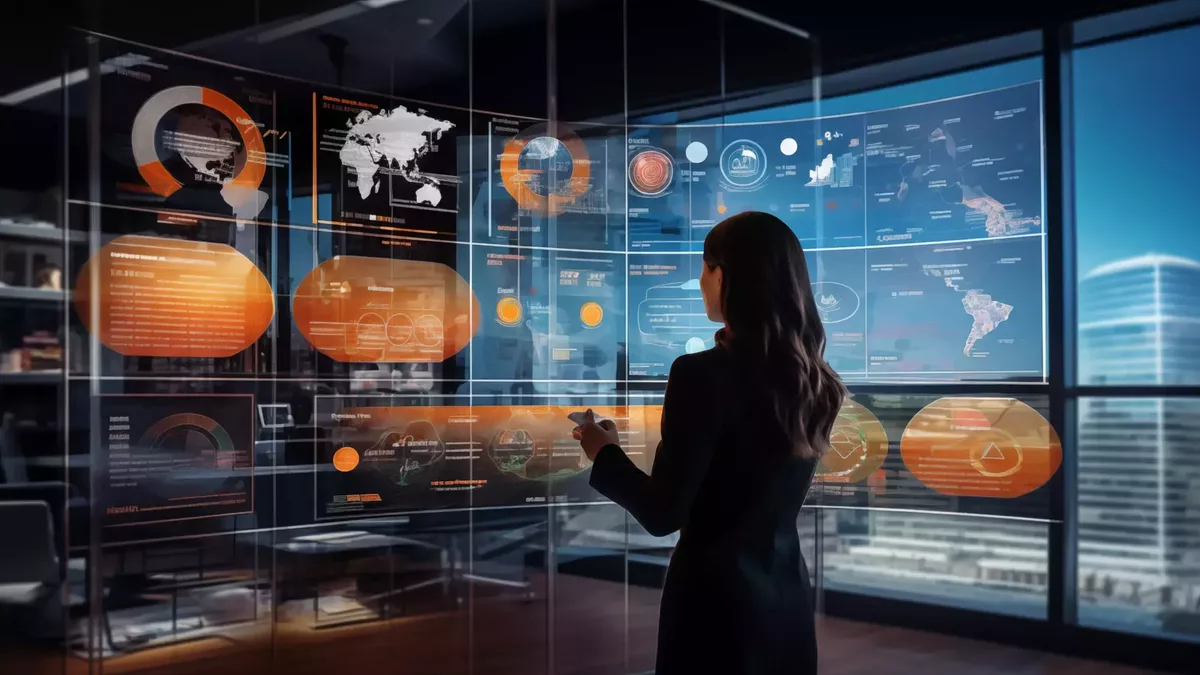
If the dashboard ecosystem (i.e., Pulse) discussed in the last piece is the compass that guides strategy implementation and operations, ad hoc or exploratory analytics form the map of the ever-shifting business landscape used to evolve strategy and operations.
The dashboard ecosystem streamlines and unifies decision-making by providing leaders at all levels with the health of their business at-a-glance with a clear call to action. This ecosystem shifts operations from reactive to proactive, reduces mountains of unused reports, eliminates significant redundancy and waste, and begins to bend the curve and improve all aspects of the quadruple aim (outcomes, patient, provider, and cost).
The dashboard ecosystem is NOT a control system to be mindlessly followed—we can’t set it and forget it. Often, the initial process measures envisioned fail to achieve the desired outcomes. Or, the business landscape evolves in ways we didn’t anticipate, so outcomes need to change. In either case, we need to adapt. We must be vigilant and constantly evaluate the business strategy and tactics—evolving the dashboard ecosystem along the way.
This dashboard evolution also involves continuously grooming measures to keep the interface simple and actionable. It is easy for a dashboard to become so packed with measures that it is unusable. Remember, an end-user should be able to tell the health of their business in four minutes or less—no analysis is required.
Exploratory analytics makes the dashboard evolution possible. These tools enable the business to evolve its strategy and continuously seek opportunities to improve operations. Often, this is supported by a small number of data analysts and data scientists working in partnership with the business, clinics, and communities. However, this approach is expensive and doesn’t scale to support a rapidly changing business landscape. For this to scale, tools need to be provided that democratize access to the masses, freeing data scientists and analysts to focus on higher-value analytics. Organizations need to implement self-service analytics.
In this piece, we will define self-service analytics and why it is essential; we will highlight several high-impact use cases, considerations when selecting a tool, and touch on a few products in the market. Let’s get started.
Defining Self-Service Analytics
Self-service analytics is a form of exploratory analysis that enables healthcare professionals to access, analyze, and visualize data independently. Unlike traditional analytics that require technical expertise, self-service analytics is designed for non-technical users. This approach democratizes data analysis, allowing clinicians, researchers, administrators, staff and leaders to make data-driven decisions without extensive reliance on data analysts or other technical support.
Common characteristics include an intuitive user interface, easy access to diverse data sources (patient, staffing, supply and financial data), and the ability to perform ad-hoc analysis and generate reports. Often, the interface is tailored to the use case or user type with guided navigation and out-of-the-box reports—sometimes leveraging advanced analytics.
A less common but essential feature is the ability to define cohorts. This enables comparative analysis within the tool. This is important when looking for process improvement opportunities, evaluating changes to processes or protocols, or comparing current practices to industry benchmarks. Without this capability, data lists need to be exported to Microsoft Excel (one at a time) where they are compared. This is costly, slow and prone to error.
Another less common feature is the ability to create a digital twin—a digital version of the physical environment you are analyzing. For example, if you need to make a staffing change because of unexpected demand, a digital twin would allow you to evaluate different staffing scenarios before bringing in more people—reducing the risk (and cost) of over or understaffing. You could also use this capability to analyze facility changes before making changes. This is also a considerable cost saving and helps eliminate much guesswork.
There are several other valuable features that I have only seen in custom solutions. The first is the ability to compare events to trends (operational, environment, industry, etc.) to draw causality. Also, features that enable data citizens can share and collaborate on the analysis being performed.
Now that we have discussed the features, let’s look at a few use cases.
High-Impact Use Cases
Patient Flow: One of the most visible and impactful use cases is optimizing patient flow. Many healthcare systems are struggling to make ends meet. This is an area where we can significantly reduce costs and improve the experience for our patients and workforce by shifting from reactive to proactive.
Self-service analytics allows clinicians and administrators to analyze near-time and predictive data on patient admissions, discharges, throughput, and workforce staffing. This analysis enables them to identify bottlenecks and inefficiencies in patient movement throughout the facility. For example, by examining trends in admission rates, bed availability, and average length of stay, healthcare professionals can predict peak times and prepare accordingly, thereby reducing wait times and avoiding overcrowding.
Moreover, self-service analytics can help optimize scheduling for surgeries and other procedures, ensuring that resources like operating rooms and staff are utilized effectively. This proactive patient flow management leads to a smoother patient experience, decreased patient wait times, and more efficient use of healthcare resources that contribute to improved patient outcomes and satisfaction, and significantly reduced costs to deliver care.
Another impact that is important to highlight is that leveraging tools of this ilk creates a work environment that is more stable and predictable, leading to improvements in workforce experience. When burnout is at a new high for our clinicians, this is a very BIG deal.
When selecting a tool, consider the predictive model accuracy, timeliness of data (hourly refresh or better) and ability to simulate different scenarios leveraging a digital twin. Also, cloud-hosted solutions speed up the implementation and reduce ongoing administration.
Exploring how far you can go with your EHR vendor offering is essential. To fill the gap, there are several popular products on the market. A few to consider are BigBear.ai’s FutureFlowRX and LeanTaas’s iQueue. Of the available products, FutureFlowRX is the only product that I am aware of that provides digital twin support.
Quality Improvement: Another use case is empowering clinicians with a self-service analytics tool to support quality improvement. Historically, clinicians worked closely with a data analyst, data scientist, or both to support this work. There are now tools becoming available that allow them to self-serve. Using these tools, clinicians can identify real-time trends, patterns, and improvement areas. For example, they can analyze patient outcomes, treatment efficacy, and post-operative recovery rates to assess the effectiveness of different medical procedures and interventions.
Additionally, self-service analytics facilitates monitoring key performance indicators (KPIs) such as hospital readmission rates, patient satisfaction scores, and compliance with clinical guidelines. This ongoing analysis enables healthcare providers to make evidence-based improvements, tailor treatments to individual patient needs, and implement best practices more effectively. Self-service analytics helps foster a culture of continuous quality improvement by continuously monitoring and analyzing healthcare data, leading to enhanced patient care, increased safety, and overall better health outcomes. These tools also improve workforce experience by providing the clinical community with greater agency.
When selecting a tool, features beyond an intuitive user interface include creating, managing, comparing cohorts, and presenting the information using SPC (Statistical Process Control) control charts. Also, the number of dimensions, attributes and measures can be daunting. So, having a user interface tailored to the specialty can significantly improve usability.
As with patient flow, you can build a semi-custom solution leveraging products like Tableau and Qlik. However, several products on the market can give a more out-of-the-box experience that can accelerate your efforts, provide more features and reduce costs. AdaptX and Epic Cogito’s Slicer Dicer are notable products in this space.
If you are on the research side, several tools provide a deidentified, cross-institution patient dataset with an intuitive interface that can be used to compare outcomes with other organizations and evaluate the availability of patients for trials or studies. TriNetX, Epic Cosmos, and PCORI PEDSNet (pediatrics only) are two notable products.
Other Use Cases: The list of use cases is long. In addition to the areas we have discussed, there are opportunities in market planning, competitive analysis, financial planning, supply chain, revenue cycle, workforce management, patient recruiting, facilities management, and more. Where you begin should be driven based on your strategic and operational priorities. Regardless of where you start, more third-party products are becoming available to accelerate your analytics and AI journey.
Also, all EHR, ERP, and CRM vendors provide a growing suite of tools that should be leveraged before you explore other third-party products or build something more custom. That said, don’t be lulled into believing they are your enterprise data platforms. They are an essential part of the analytics and AI ecosystem but lack the breadth of features to support strategic and tactical enterprise needs and scale.
A Few Words of Caution
While self-service analytics tools offer numerous benefits and embolden the analytics community with data-driven decision-making capabilities by reducing reliance on data analysts, engineers and scientists, there are a few words of caution:
- Data Quality: With self-service tools, there is no longer a knowledgeable data analyst or data scientist between the data and end-user who can factor data quality issues into the final analysis. So, what was considered an acceptable level of data quality in terms of accuracy and completeness is no longer acceptable. You will need to invest in improving data quality. If this isn’t possible, this will increase the complexity of using the tools, and you will need to factor this into your education curriculum.
- Data Fluency: Learning any new tool is relatively easy. Learning how to tell stories with data is much, much more complicated. This can lead to incorrect conclusions and potentially harmful decisions, especially in sensitive fields like healthcare. This involves becoming proficient in framing the objective of the analysis, developing a deep understanding of the data (definition and lineage), and effectively interpreting and communicating the results—avoiding the cognitive bias that can often get in the way of the true story. This also involves learning the difference between deterministic data like the current census and probabilistic data like the predicted census and ensuring that this data is used ethically.
- Operational Integration: This is probably the most significant risk to successfully adopting self-service analytics. Without the right level of alignment and support within operations, there is no way to leverage what has been learned through self-service analysis. We learn (and spend) a lot but everything stays the same. What I have seen work well is when operations co-leads the implementation of these tools, and there is strong support from their executive, middle and frontline leadership. To help with this alignment, it can be beneficial to form a tiger team to solve specific problems—e.g., eliminating surgical cancelation, improving ED to acute care transfers, eliminating the use of opioids in outpatient surgeries, or improving OR turnaround for a procedure.
- Vendor Dependence: Relying on a specific vendor's tool can lead to dependence, making it challenging to switch tools or integrate with other systems in the future. To provide some insulation and protection, consider how you implement the solution. For example, building a single version of truth (i.e., data mart) as the base for these tools can make the migration to other products easier. Also, negotiate pricing as far out as possible—without making upfront commitments for payment of more than one to two years.
While self-service analytics tools offer significant advantages, they should be implemented carefully considering these potential issues. Proper training, data governance, and a balanced approach to data analysis are key to effectively leveraging these tools for informed decision-making and action.
In Closing
Throughout this series, we have discussed the importance of democratizing data access in powering data innovation. Creating a single version of the truth and increasing data analyst productivity and proficiency are essential steps in that direction. However, self-service analytics is the game changer. This makes data and analytics accessible to thousands—dramatically accelerating your journey and providing greater agency to people in the organization.
In this piece, we have discussed the role of self-service analytics and how it complements your dashboard ecosystem. In addition, we have discussed several areas where self-service analytics is being used to drive transformative impact, a sample of products available, considerations when selecting a product, and a few things to consider when you take this step in your journey to improve your odds of success. To this end, I hope you found this helpful and would greatly value other thoughts you may have and questions.
In the next piece, we are going to change gears a bit. We have talked about a great many things in this series: the changing business landscape and the need to accelerate our analytics and AI journey, the operating model and entrepreneurial mindset that makes this possible, how to get started, and the platform, frameworks, and technology that will enable the journey. In the next piece, we will focus on leading the expedition.