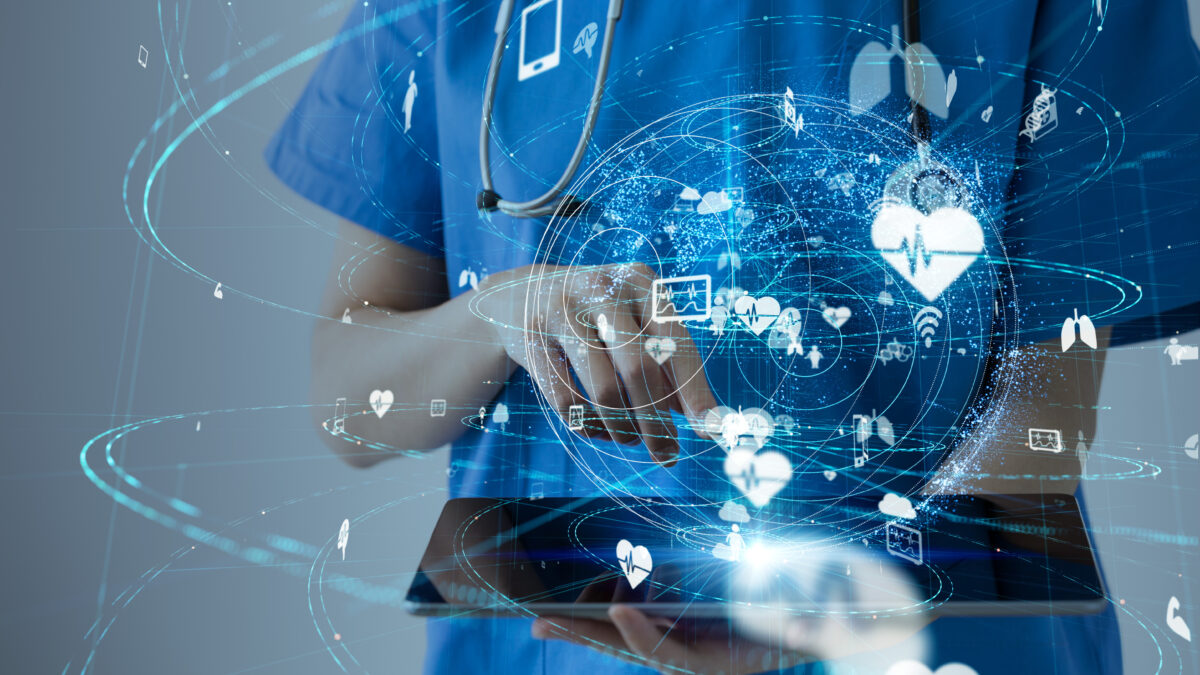
Powerful forces are disrupting the healthcare industry — healthcare costs, consumer convenience, patient empowerment, workforce health, health equity and emerging technology. These forces are driving changes that will improve patient outcomes, patient experience and workforce experience, reduces costs, and promote health equity. Those who quickly learn to innovate with data are well-positioned to thrive and lead. The rest will likely struggle to survive, go out of business, or be acquired — possibly by some newcomer we’ve never heard of.
Before diving into a proven operating model leveraged by organizations that have prospered, it is helpful to understand several equally powerful forces against change that need to be navigated to be successful — the most powerful being the legacy analytics ecosystem and status quo. Let’s explore each briefly.
The Forces Against Change
Legacy Analytics Ecosystem
I’ve always wondered what it would be like to join an organization where the analytics journey was just starting, or where people were raving fans of their analytics ecosystem. I am sure this world must exist, and if you are there, cherish it. Sadly, I’ve never had that good fortune in over thirty years. Within and outside of healthcare, the illustration below better reflects my experience as the starting point for the journey.
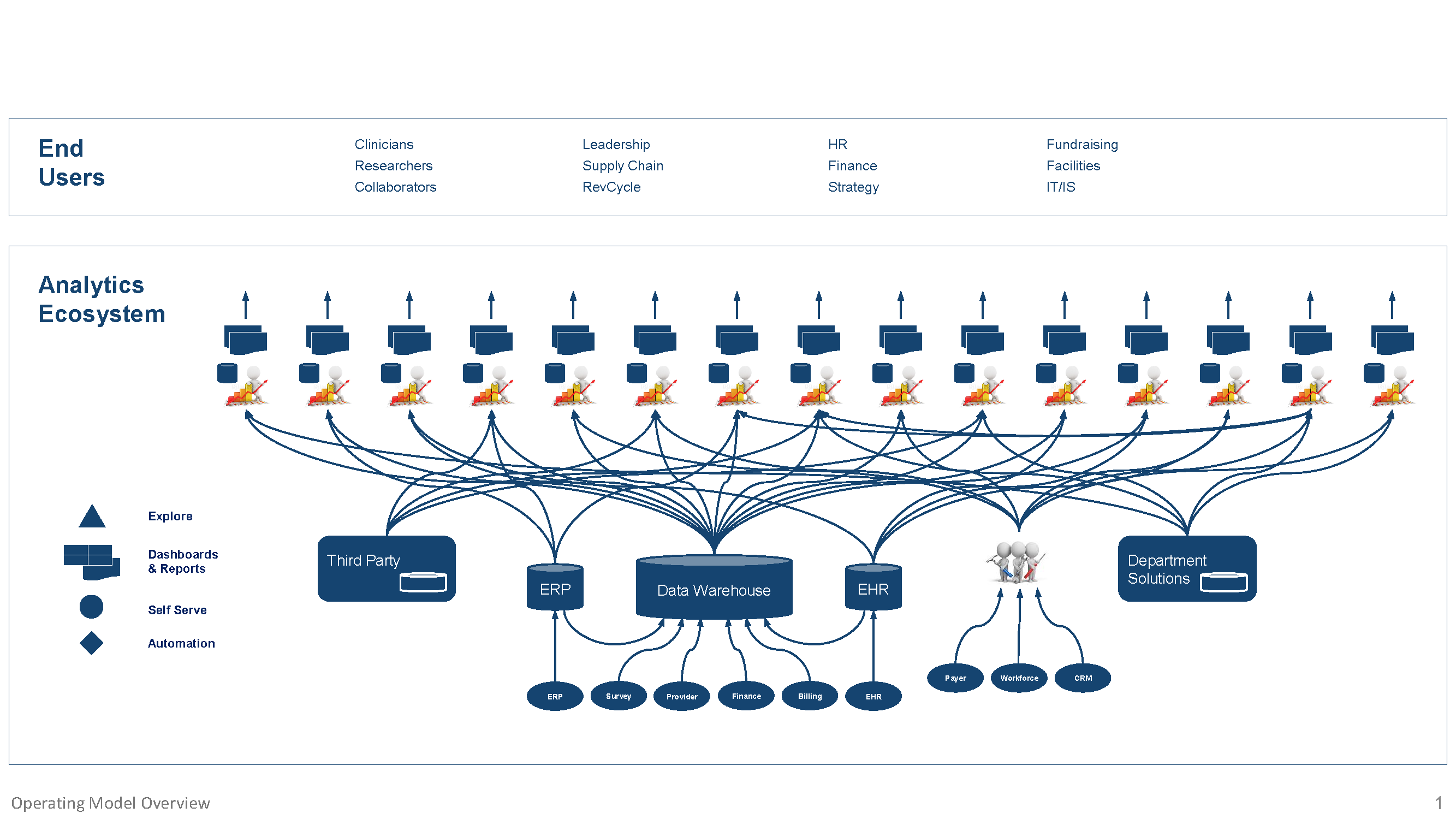
In this ecosystem, analytics started as a strategic effort to create a single data warehouse to support the organization with “strong” centralized governance. Over time, people got tired of waiting, converted operational positions to data analyst positions, gave these data analysts a tool like Tableau or PowerBI, and set them loose. These data analysts quickly exhausted what they could get from the data warehouse and began to source from operational systems directly (EHR, ERP, CRM, etc.), external third parties and other departments. In time, the ecosystem became a patchwork of departmental reporting solutions that were increasingly expensive to maintain and didn’t scale to support the strategic and operational priorities of the business. Sometimes, these reporting databases are large, sophisticated, heavily used, and support critical business operations – though the analytics capabilities are limited to reporting with some dashboards, with very little in the way of self-service, deep exploration, advanced analytics or automation.
As part of joining a new organization, I always enjoy meeting with people to understand what is and isn’t working. Almost without fail, departments are happy with their data analysts’ work. At the same time, they are very critical of the broader ecosystem. They have concerns about data quality, multiple versions of the truth, inaccessibility of data, limited access to self-service tools, lack of predictive analytics, immature data fluency, slowness of central IT to respond to requests for new data and tools, and the list goes on. Central IT teams are generally overworked trying to keep up with demand, and an aging data and analytics platform. They are increasingly isolated from the business, have decided to focus on what they can control and often give up trying to harmonize analytics across the ecosystem.
This is not a sustainable trajectory for an organization. However, I have come to appreciate that this is a natural part of the evolution of the analytics ecosystem — this isn’t a bad thing but a part of the evolution. At this stage, organizations have come to appreciate the value of analytics and have begun to innovate with data. They have started to grow their data fluency and talent density for the next stage in their journey. However, the growing lack of resiliency in solutions, the increasing overhead for maintenance, the limitations in the data platform, and the lack of trust in data prevent these organizations from evolving to the next level.
At this point, it is a common response to put centralized controls in place to manage the sprawl — especially in traditional industries like healthcare where extrinsic motivation is the default mode for leadership. I have seen this play out dozens and dozens of times, and have never seen this approach work. This approach slows the evolution of the ecosystem, decreases productivity, reduces employee satisfaction, and stifles innovation. Also, people always find ways to work around these controls – probably not where we want people innovating.
I have come to value and embrace the idea that the obstacle is the way. Rather than trying to slow down these embedded solutions and deal with resistance to change, invest the energy in creating a better place for them to go when they come looking for help — and they will either before or after they hit a wall. When this happens, partner with them to migrate their excellent work to an analytics ecosystem (and community) that provides the flexibility they have come to value while providing a high-fidelity data and analytics-AI platform that will scale to support future needs. We will discuss the operating model that provides the framework for this ecosystem a bit further down.
Status Quo
The status quo is another considerable force against change. As we discussed above, the legacy operating model for analytics isn’t resilient, isn’t trusted, isn’t scalable, and is wasteful. However, people resist changing it. Fear of change and cognitive bias has a lot to do with it. In healthcare, we have the added force of standard work. In this environment, people are strongly encouraged to color inside the lines and reprimanded when they don’t. To be clear, I am a big fan of standard work and evidence-based medicine in the clinical setting. If I must have open heart surgery, I want my surgeon to use standard work informed by research, and not experiment with something they dreamed up while showering earlier in the day.
Though this approach is paramount to evolving healthcare delivery that is safe and effective, this approach stifles our ability to innovate with data. Leading organizations have become very adept at democratizing access to data, creating an ecosystem that rewards trying many things quickly, and learning from each experience. Where they find success, they double down on the opportunity; where they encounter failure, they quickly stop the effort and reflect on lessons learned. In this environment, there needs to be a healthy disrespect for the status quo, and people need to be encouraged to color outside the lines with the caveat that they don’t create legal liability, financial risk or put patients in harm’s way.
As you might expect, best practices continuously evolve in this seemingly “chaotic” environment as teams learn and adapt. Often tribal mentality kicks in and people begin to see this as a problem to solve because these teams operate differently than other groups. Leading organizations see these differences as healthy. They have embraced the idea of one team, one mission but not one way. They adopt a bi-model approach to delivery that leverages team diversity to improve productivity, promote innovation and increase employee satisfaction. They understand that if you hire diverse talent, you must allow them to express themselves in diverse ways to realize their potential. We will discuss an operating model that promotes this diversity shortly, and in the next article will dive into the entrepreneurial mindset that powers this operating model.
The Genesis
You have undoubtedly seen many organizations bouncing back and forth between centralized and decentralized operating models — always moving two steps forward and one step back. Each approach has its benefits, as illustrated below. The centralized approach promotes more resilient, sustainable, efficient and reusable solutions. The decentralized approach promotes solutions better aligned to strategic and operational priorities, more responsive to business needs, have a high business impact (perceived or otherwise), and deliver value incrementally and often. So, the ideal operating model would blend the best of these worlds into a balanced ecosystem.
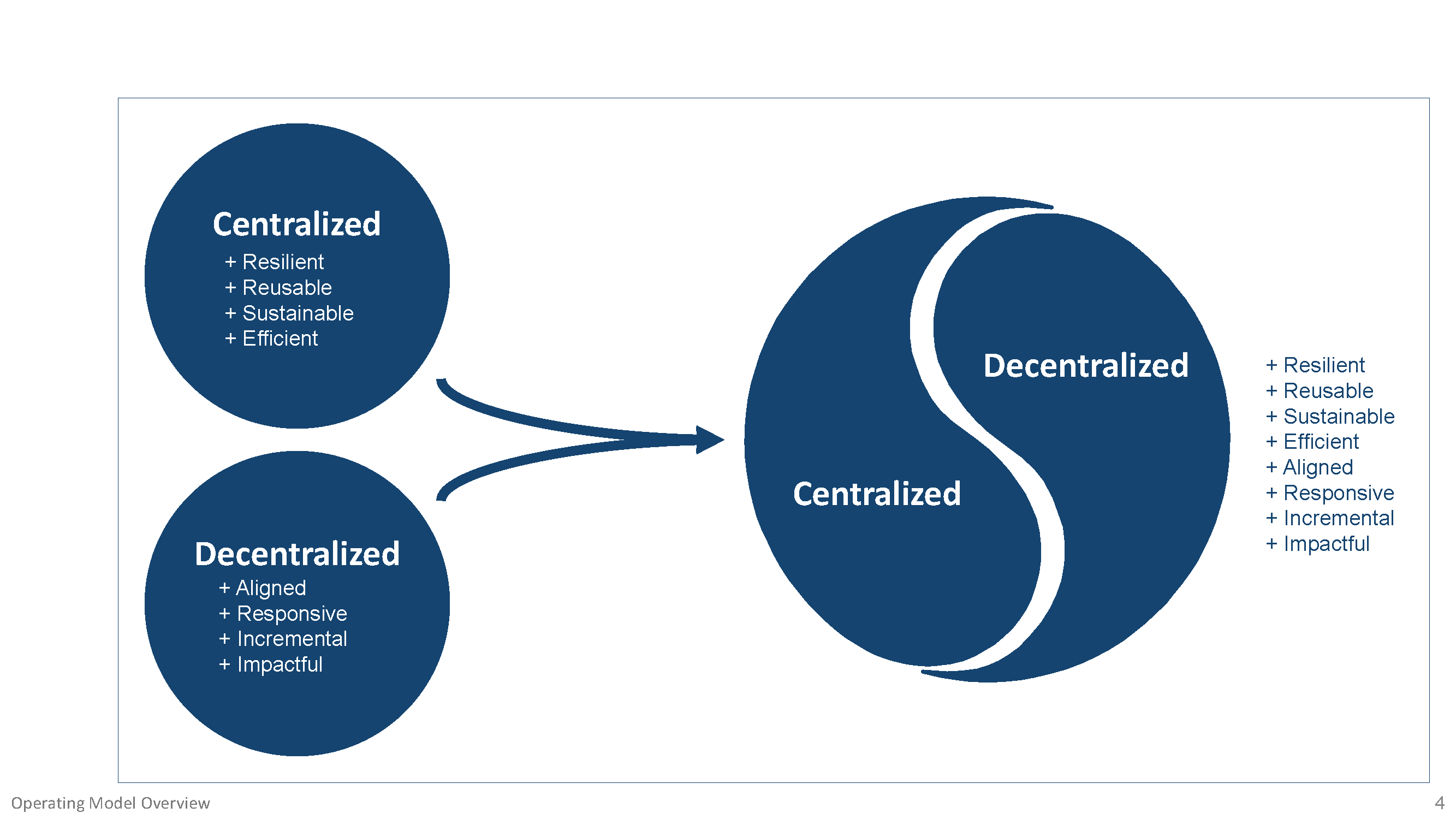
You can’t have your cake and eat it too. Right? No doubt we have all heard adages of this ilk growing up and have quickly accepted sacrifices that limited our imagination and possibilities. Fortunately, several early mentors reprogrammed me to ask a different question, “How can you have your cake and eat it too?” This was the genesis of my journey to find an operating model that was adaptive and uncompromising, a model that would allow me to have my cake and eat it too.
This journey landed on a federated operating model common in startups, digital natives and some traditional organizations. This model is often adopted because it is scalable and adapts well to complex environments that are changing rapidly. This model’s foundation comprises small multidisciplinary product teams organized around strategic and operational priorities. These teams operate like a startup and are organized into a community by a lightweight framework. The guiding principles for this framework are:
- Promote decision-making where work is done
- Deeply integrate business, clinical and technology
- Enable team members to develop deep expertise
- Increase focus on strategic and operational priorities
- Engage senior leadership at the appropriate level
- Minimize handoffs to complete work products
- Evolve a single data and analytics platform
- Encourage a strong sense of community
- Promote loose coupling
The product teams are the heart of the operating model. This is where work gets done, and value is created. They are called “delivery teams” to reinforce their purpose of delivering business value. Let’s take a closer look at a delivery team.
The Delivery Team
The idea of the delivery team is simple and proven. We only need to reflect on our recent experience during the pandemic to see this in action. To create focus, efficiency and speed, we organized into small teams focused on areas that were critical to the pandemic response. These teams were multidisciplinary, and comprised of business, clinical and technology resources. They had a clear purpose and the autonomy to respond as necessary to achieve this purpose. No two teams operated the same way – we were too focused on results to care. However, they were highly effective and adaptable in responding to the ever-changing landscape throughout the pandemic. Reflecting on this experience, people often ask, “Why don’t we always operate this way?” This is an excellent question. The answer is, “We can.” This is the essence of a delivery team.
Rather than a pandemic driving urgency and focus, delivery teams organize around strategic and operational priorities. Typical areas of focus are patient flow, clinical excellence, patient access, revenue cycle, supply chain, quality and safety, infection prevention, clinical research integration, systems medicine, business strategy, corporate finance, ambulatory, surgical services, service lines, health equity, EHR migration, ERP migration, etc. As you probably noticed, delivery teams can be organized to support departments, functions, or projects. So, the life expectancy can be as short as 6-18 months or as long as 2-plus years. Once these teams are formed, they continue to operate as long as they drive value to justify the investment, or it is decided that greater value can be achieved elsewhere.
Here are some of the delivery team responsibilities:
- Form and evolve team charter
- Own data product innovation, delivery, operations, and adoption
- Ensure alignment with strategic and operational priorities
- Develop and leverage delivery team best practices
- Own data stewardship for selected data domains
- Promote reuse across delivery teams
- Contribute to tool assessments
- Promote data fluency
In many ways, these delivery teams operate like a startup. They have the freedom to do what needs to be done to maximize the value of their business. They also have ultimate accountability for their decisions and actions. Let’s take a closer look at the anatomy of a delivery team.
The Delivery Team Anatomy
Delivery teams embody the agile manifesto and guiding principles. If you have scrum experience, the delivery team's structure and practices will look familiar. However, there are some significant differences. Let’s dig in.
There are two leaders for the delivery team: product owner and dev owner. Embracing the guiding principle of decision-making where the work is done, the product owner is responsible for deciding what to do, and the dev owner is responsible for determining how to do it — they are the team’s co-CEOs.
In high-functioning teams, the product owner has a deep understanding of the business they represent with an affinity for technology, and the dev owner has a deep understanding of the technology with an affinity for the business. These leaders will often challenge each other. However, they respect the product owner's final say in “what” gets done and the dev owner's final say in “how” it gets done. For clinically focused delivery teams, the product owner will often have a clinical leader assigned that actively represents the clinical community.
The size of the team is three to six people. Amazon calls these “pizza teams” (if it takes more than two pizzas to feed the team, it is too big). These teams comprise full-time data engineers, data analysts, and sometimes data scientists and software engineers. They also might have part-time representation from security, data architecture and data ops. Noticeably absent are project managers, business analysts and testers. These roles are either unnecessary or absorbed into other roles on the team.
To provide visibility and solicit guidance around decision-making, the co-leaders partner closely with the delivery sponsors and advisory team. The delivery sponsors include the senior analytics leader (e.g., Chief Data and Analytics Officer) plus one to two executive leaders — ideally, one who reports to the CEO. These leaders provide guidance and support to help ensure the work aligns with strategic and operational priorities, and they assist in clearing barriers. They also advocate for the work of THEIR delivery team and share accountability for outcomes.
The other important function is the advisory team. This team comprises representatives that will use the data products created by the delivery team. Their role is to provide context to guide decision-making, provide feedback on early versions of data products, and promote adopting data products released into the wild. Below is an illustration of a delivery team.
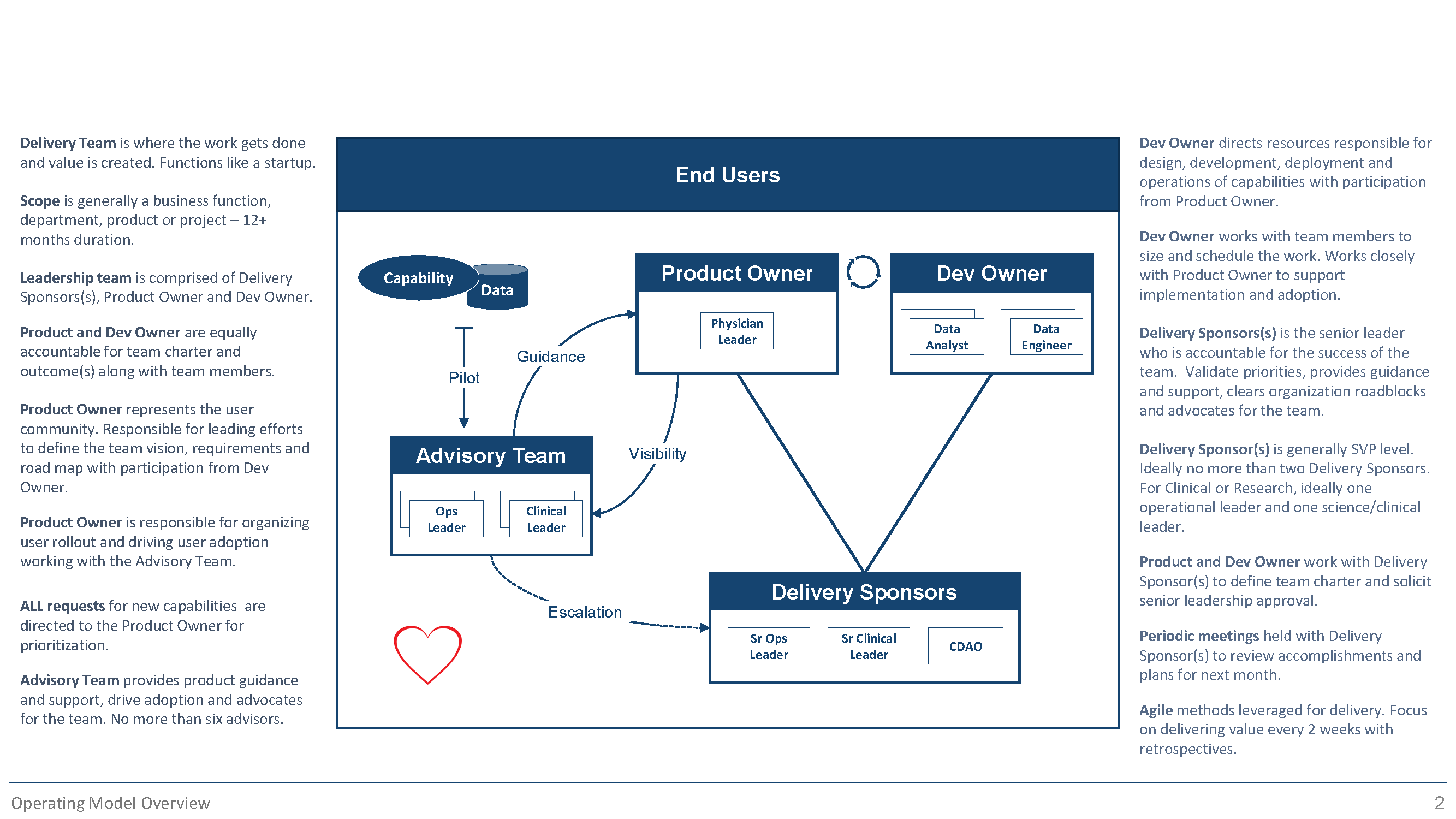
Those experienced with scrum will notice two crucial differences. The scrum master has been replaced by a dev owner, and team members report to the dev owner. In some circles, this is sacrilege. I get it. The spirit of agile is to create an environment that motivates people intrinsically to promote innovation, productivity and employee satisfaction in a way that enables team leaders to inspire from a position of influence rather than authority. I have experienced the power of intrinsic motivation, and I am a passionate advocate.
Here is the rationale for these differences – right or wrong. Scrum masters are often shared across teams and don’t have the same level of commitment to the cause. They are more a consultant to the business than an employee. The full-time dev owner role was created to promote greater ownership and engagement from the development leader and their team members. First and foremost, this is a person who can motivate intrinsically. They own the build, deployment and management of data products working in partnership with the product owner and team members. This person does many of the same things as the scrum master. In addition, they roll up their sleeves, participate in the delivery of data products, and share accountability for the outcome with the rest of the team.
Now that we’ve discussed the delivery teams, let’s explore how they evolve to form a community.
The Delivery Team Community
To select the initial delivery teams, it is helpful to understand what analytics are being performed and how they align with strategic and operational priorities. It is also helpful to explore which groups are receptive to making a change, those who are interested but don’t want to be first, and those who are not interested. The ideal starting point is one or two delivery teams focused on areas where there are highly engaged business partners, some level of analytics being done, active executive engagement, and an opportunity to deliver value quickly that aligns with visible business priorities.
At first, it may not be clear where to start — especially if you are new to an industry. However, time is your friend. As you dig deeper and deeper, the path forward will come into focus and you can get to work. The first couple of teams take three to five months to ramp up, and the journey can be a little bumpy as people learn a new way of working. In time, these teams demonstrate success, and others express interest in joining the journey. It isn’t long thereafter that the delivery team community begins to form — see the illustration below of how this might look.
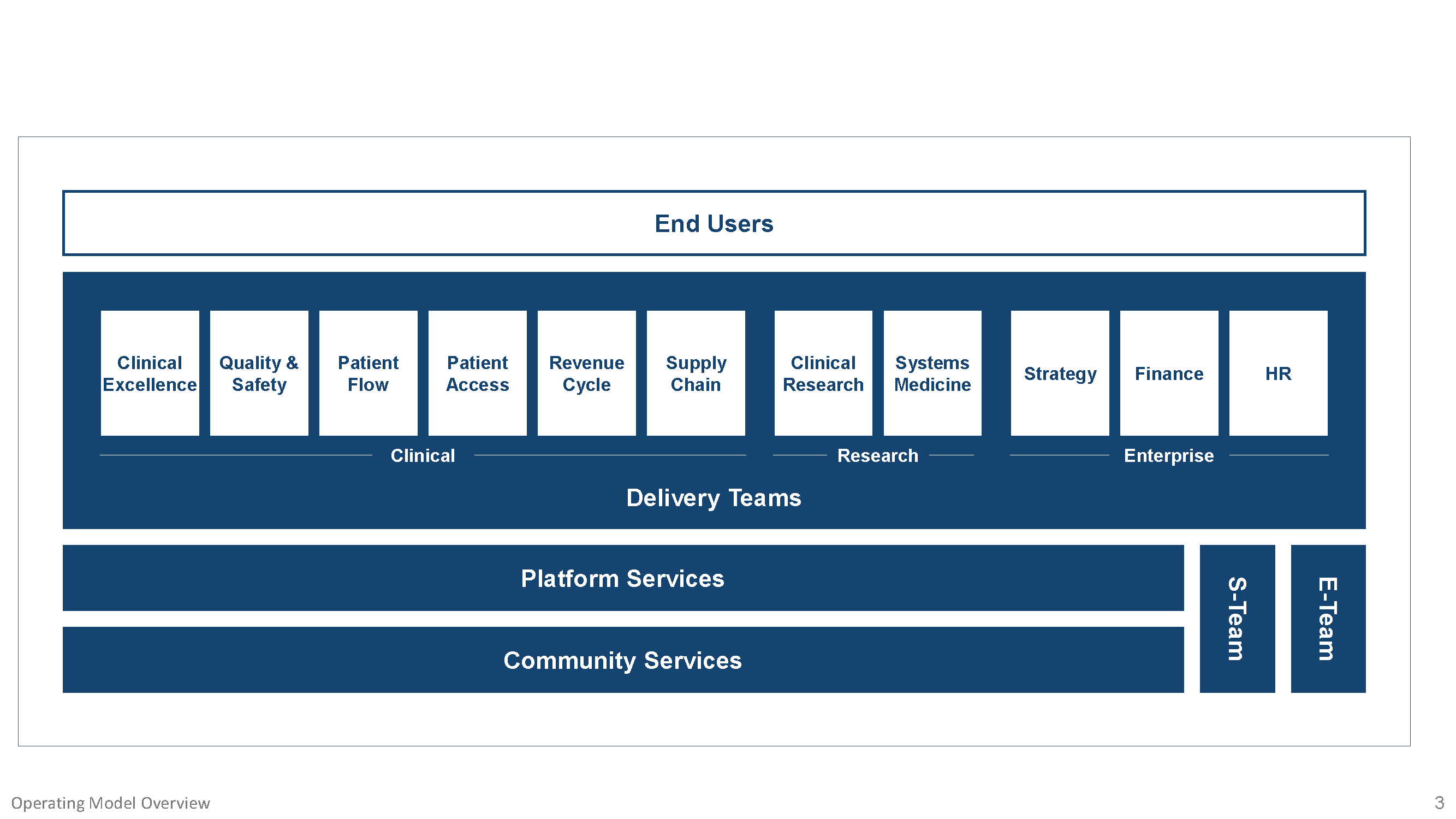
As discussed earlier, the delivery teams are loosely coupled and operate independently with an obsession to demonstrate value in their business area. Left to its own, this approach is slightly better than a decentralized operating model. To achieve success, it is essential that the delivery teams operate as a community, and evolve a resilient, scalable, and extensible data and analytics platform that looks like it was built by a single team — one that doesn’t look like a Frankenstein. To this end, four teams provide the lightweight framework to align and unify the analytics community.
Platform Services
Platform services’ first purpose (job 1) is to keep the data and analytics-AI platform healthy and operating at peak capacity. Their second purpose is to deeply integrate and enable other delivery teams – staying one step ahead of their needs. This team is akin to DevOps and DataOps and operates like other delivery teams. They are responsible for systems monitoring, database administration, incident management, performance tuning, capacity management, process automation, tools assessments, platform migrations, build and deployment automation, etc. To this end, platform services partners closely with other delivery teams to maintain and evolve the data and analytics-AI platform.
Community Services
Community services is usually a smaller team responsible for partnering with delivery teams to implement frameworks that align and nurture the analytics community.
The goal of the operating model is to have as few processes and rules as possible to promote freedom, accountability and adaptability. The benefits of this approach to motivating have been researched extensively over fifty-plus years in advancing innovation, productivity and employee satisfaction. Daniel Pink does a wonderful job covering this topic in his book titled Drive: The Surprising Truth About What Motivates Us. There are also a couple of great books that demonstrate how leading organizations have implemented and benefited from this approach: No Rules Rules: Netflix and the Culture of Reinvention by Reed Hastings and Erin Meyer and Skunk Works: A Personal Memoir of My Years of Lockheed by Leo Janos and Ben R. Rich.
To this end, the community services team leverages frameworks. These frameworks are guidelines and not hard and fast rules. They are developed by working in close partnership with delivery teams – often initiated by delivery teams. Ultimately, the delivery teams own the frameworks and understand the benefits. It is also worth pointing out that some frameworks are embodied in a tool to promote efficiency and consistency.
If people see the need to operate outside the framework, they are encouraged to do so with the caveat that they understand the guideline's purpose. They are also expected to leverage their lessons learned to improve the framework. This is part of what it means to be a citizen of the analytics community.
S-Team
The senior leadership team (or S-Team) is comprised of the leadership of the delivery teams. These leaders work together to evolve the enterprise analytics strategy, develop quarterly “big rocks” to deliver that strategy, and work collaboratively throughout the quarter to deliver the “big rocks.” This may be the most crucial function in creating a unified community. Here are some of the S-Team responsibilities.
- Align strategy: Align strategies across delivery teams and with business strategic and operational priorities
- Promote delivery: Co-own delivery of quarterly “big rocks” (i.e., OKRs), assist in resolving blocking issues, develop and adopt practices that accelerate delivery
- Create a data common: Co-own developing and leveraging frameworks that promote the creation of a unified data and analytics-AI ecosystem
- Promote data fluency and ethics: Develop a working understanding of how analytics-AI is disrupting healthcare and ethical considerations, mentor and coach others
- Advocate for the community: Promote accomplishments and solicit feedback on plans for business and technology partners
E-Team
The executive sponsor team (or E-Team) comprises executive sponsors for the analytics community. The team should be as small as possible to keep it nimble and effective. The ideal team would include an executive business leader (e.g., COO, CCO, CFO, etc.), clinical leader (e.g., CMO or CVO), research leader (CRO) and analytics leader (Chief Data and Analytics Officer). Here are some of the E-Team responsibilities.
- Guide strategy: Ensure alignment with business strategy and operational priorities
- Support execution: Clear organizational barriers and promote adoption, provide feedback and support on policies and practices, and approve delivery team formation
- Promote data fluency, ethics and equity: Develop a working understanding of how analytics-AI are disrupting healthcare and ethical considerations, mentor and coach others
- Advocate for the community: Promote accomplishments and solicit feedback on plans from senior leaders, board members, donors, and patients and families
Understand the operating model: Guide the evolution and mentor others
Chief Data and Analytics Officer
The one function integrated into nearly every aspect of the formation and evolution of the community is Chief Data and Analytics Officer (CDAO). Among other things, the CDAO is responsible for stewarding the evolution of the ecosystem and instilling the vision, mission and guiding principles. Here are some of the responsibilities of the CDAO.
- Power data innovation that drives action leveraging analytics-AI
- Lead efforts to define and prioritize transformative strategies
- Harmonize strategies and leverage rich data assets to fuel these strategies
- Work within the business to deeply embed and evolve an analytics ecosystem that emboldens these strategies
- Work within technology to build and evolve a scalable, adaptable analytics platform that powers the ecosystem
- Work across business and technology to implement an operating model to ignite and evolve the ecosystem
- Stewards and guides strategy definition, execution and evolution, working in partnership with business, clinical, research, payer and technology partners
The Community Evolution
As the first couple of delivery teams ramp up and begin to demonstrate value, you will likely no longer need to look for candidates for new delivery teams. People will come to you. So, you will need to have a process to vet what teams to build and what teams to table. There is never enough capacity to support demand.
Let’s start by saying there is no right way to decide which delivery teams should be formed and which should be allowed to continue. Here is the process I have used to make the decision as democratic as possible and help to ensure great ideas don’t get lost in committee.
- Someone or a group has an idea – growth, quality, cost savings, etc.
- The idea is assessed to ensure it aligns with strategic and operational priorities.
- An Action Plan for the delivery team is created that is focused and compelling. The Action Plan is akin to a lightweight business plan. We will talk more about its role in a future article.
- The Action Plan is pitched to executive sponsorship for approval and seed funding.
- If seed funding is received, the team will have 3-6 months to demonstrate value to the business.
- If value is demonstrated, the team will receive incremental funding to expand product functionality and use.
- Investment will continue until the value proposition no longer justifies the investment, or funds can be better used elsewhere.
In Closing
We have covered much ground. We’ve explored challenges faced as we evolve the analytics ecosystem – legacy analytics ecosystem and status quo. We’ve discussed the genesis of the delivery team, and how it can be used to navigate this landscape by incrementally organizing resources into adaptive, multidisciplinary product teams focused on strategic and operational priorities. We’ve discussed how these teams can evolve to create a robust, valued analytics community that is adaptable, scalable, resilient, and continuously drives greater business impact.
That said, there is so much more to discuss: How do we fund delivery teams? How do delivery teams deliver? What are the frameworks in a mature environment? How do we build talent diversity and density? How does the quarterly planning and review process work? How does this align with overall enterprise strategic and operational priority? How does this approach harmonize with lean practices? How does this model promote data fluency and ethics? And the list goes on. These are great topics for future articles. If you have others, please let me know – and if you would like to co-author.
If you have made it this far, congratulations, and thank you for your time. I hope you found the content valuable and would love any feedback.
In the next article, I am excited to share that Mary Jo Stojak will join me. She is a practitioner and leader who exemplifies an entrepreneurial mindset. We will dive into how this mindset powers and is emboldened by the federated operating model. As I have mentioned, I have seen poor operating models do well and great operating models do poorly. In the end, the people and mindset make the difference. We will discuss the power of intrinsic motivation and how to harness this power. We will explore how to nurture and shift to this mindset in a healthcare organization that tends to be more extrinsically motivated. Lastly, we will discuss the importance of talent density in this evolution and how to nurture it.
Below is recommended reading for those who want to explore the topics discussed – carried over from the last article. Also, I’ve included books that provide great context for the upcoming article on accelerating your data innovation journey requires thinking like an entrepreneur.
Onward…
Recommended Reading
Accelerating your journey requires operating like a startup
The books I suggest are Competing in the Age of AI, co-authored by Marco Iansiti and Karim R. Lakhani, and Fail Fast, Learn Faster by Randy Bean. These books do a wonderful job describing the impact of this digital operating model. The first book discusses how digital natives (Amazon, Ant Financial, etc.) have leveraged this model to disrupt industries (retail, finance, hospitality, etc.). There are no healthcare examples, as we have only begun that journey. I found reading this book a great creative exercise as I adapted the concepts to healthcare – it forced me to think outside the box. The second book does a nice job of describing how traditional organizations that survived made the transition. This book is much easier to translate to healthcare but doesn’t get as deep into the digital operating model.
As for articles, I suggest two. The first is a paper published in Learning Health System about the Seattle Children’s Hospital data innovation journey titled “Successful Operational Integration of Healthcare Analytics at Seattle Children's.” This provides a good case study for how the digital operating model can be leveraged in healthcare. The other article was published by McKinsey & Company, “How to Unlock the Full Value of Data? Manage It Like a Product.” A core element of the operating model is shifting from a project management framework to a product management framework. This article does a nice job of introducing this idea and the steps to get started. If you like this article, a version goes into more detail in Harvard Business Review.
For IIA’s viewpoint and observations on organizing analytics and data science organization, check out this eBook. It describes and offers guidance on the fundamental goals and objectives of organizational structure, a new continuum-based approach to organizing models, guidance for determining where your organization falls on the continuum, how analytics organizations commonly evolve and their paths, and questions and considerations tied to planning your next move.
Accelerating your journey requires thinking like an entrepreneur
Several books provide great context for the upcoming article on accelerating your data innovation journey requires thinking like an entrepreneur. Intrinsic motivation is at the core of the entrepreneurial mindset that powers the operating model presented in this article. To understand this way of motivating, there is no better place to start than Drive by Daniel H. Pink. To see the power of this way of motivating, I recommend three books: Think Like a Rocket Scientist by Ozan Varol, No Rules Rules by Reed Hastings and Erin Meyer, and Skunk Works by Ben Rich and Leo Janos. To see a framework for intrinsically motivating, I would recommend The Art of Action by Stephen Bungay.