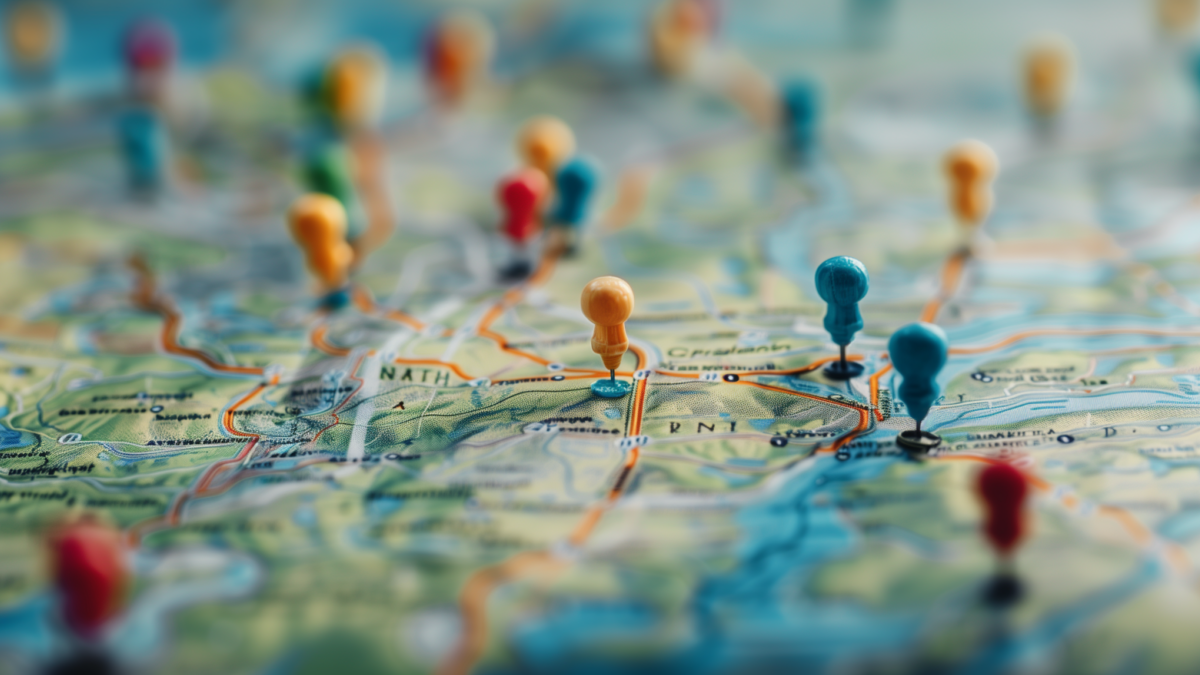
Every company and every function in all companies have operating models whether they know it or not. And even if they know it, they might not see value in clarifying and documenting it. In this sense an operating model is like a diet. Everyone eats and so everyone has a diet. Some people, however, are more intentional in their diet, often in pursuit of another goal—adherence to ethical or religious principles, improved health or to drive athletic performance. Similarly, companies and functions that are conscious that they could secure better alignment to their goals and improve their outcomes, should consider evaluating their operating model, which is why the evaluation and transformation of corporate operating models are often guided by elite consultancies at the behest of the CEOs and boards. The deliberate and famous operating efforts of companies like Disney, IKEA, and Alibaba attest to the power of this exercise.
In Part 1 of this series, we’ll explore the benefits of a better operating model and the components of IIA’s framework to map it. In the next article, we’ll discuss a scorecard that can be used to evaluate your operating model and an example of this evaluation in action.
IIA RAN clients have access to the full analytics operating model framework and supplemental resources here.
Bringing the Operating Model to Analytics
For the sake of this discussion, we will use an extremely simple definition of “operating model”: An analytics operating model is a description of how analytics products get done in an organization.
Since analytics as a field continues to grow in scope, scale, and impact we believe data and analytics functions should examine their implicit operating model and actively work on continuously improving it if they want to deliver competitive advantage.
Some indications that you should consider mapping and improving your analytics operating model include:
- Continued high variability in whether or not your analytics products meet demand-side expectations.
- Continued high variability in whether or not your analytics products can be scaled.
- Confusion or opacity in how analytics products get delivered as evidenced by business leader disengagement.
- Uneven development of analytics and business leader capabilities in analytics, where some teams continuously improve while others are stuck in POC limbo or in only incremental gains in analytics sophistication.
Below are two key characteristics that should be in place to ensure that the effort of mapping your analytics operating model is a worthwhile pursuit. The first reflects that the way you deliver analytics products has enough complexity to merit mapping the operating model and the second indicates that your firm will have the motivation to act on the findings.
- Your analytics efforts have some enterprise underpinning, meaning analytics are a cooperative effort involving partners from other functions, like key business units, IT, data management, and so on.
- Your firm has ambitions to improve its analytics outcomes.
Provided these two characteristics are in place, it does not matter if you have high data literacy, a strong data culture, or analytics maturity. In some cases, mapping the analytics model can be a part of improving these. You can also undertake a mapping of your analytics model even if you’re managing functional analytics so long as there are dependencies in other parts of your organization like IT or centralized data management and governance.
The purpose of this blog series is not to define the optimal operating model. That would be silly. There is no such thing. Instead, we’ll offer guidance and a framework for mapping your operating model along two dimensions: effectiveness and efficiency.
In other words, is your operating model answering the right business questions with analytics solutions that increase value of the firm? And are you doing this with minimal waste, double work, and coworker frustration, thereby increasing throughput?
Operating models are not static, so a key outcome of the framework will be to help you define some overarching themes that should remain to guide you in the continued modification of your work optimizing your analytics operating model.
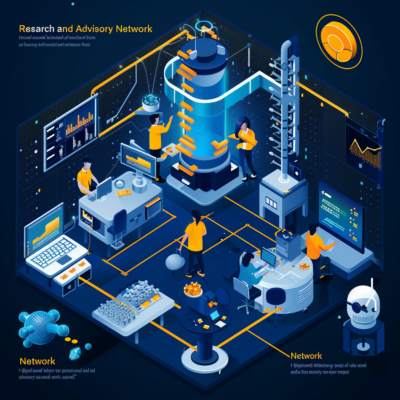
Mapping Your Analytics Operating Model eBook
Operating models are like diets. This is true of analytics operating models as well. Every firm that delivers analytics has an operating model, but few take the time to examine and improve theirs.
Benefits of a Better Operating Model
In Part 1 of this series, we’ll explore the benefits of a better operating model and the components of IIA’s framework. In the next article, we’ll discuss a scorecard that can be used to evaluate your operating model and an example of this evaluation in action.
From experience, research, and client interactions we see the following areas that can be substantially improved with an effort to optimize your analytics operating model.
- Increased connections between the parties that drive enterprise analytics (analytics, technology, and business, generically speaking) leading to stronger demand-side engagement which in turn increases the relevance and value of analytics products for the firm.
- More direct connections between business needs and the underlying data used in analytics.
- Increased understanding in the analytics organization about the variety of models that drive different analytics products including better matching of models to needs and a more informed use of more advanced and computationally intense models.
- More models in total in development and more that make it past any type of test or proof of concept phase and into deployment leading to greater throughput.
- Higher response by users that the models make their work better and are worth the required change, increasing the appetite for analytics more broadly in the firm.
- Increased transparency about which models continue to deliver and faster action on under-performing models.
The act of working through the framework and mapping your operating model is likely just as valuable as the output you get from completing the framework. This we know from experience and from client feedback. The discussions you have which surface the challenges, pain points and opportunities, as well as the clarity you will gain on the components of your analytical operating model will be worthwhile, even if you make only stepwise and iterative changes to the model.
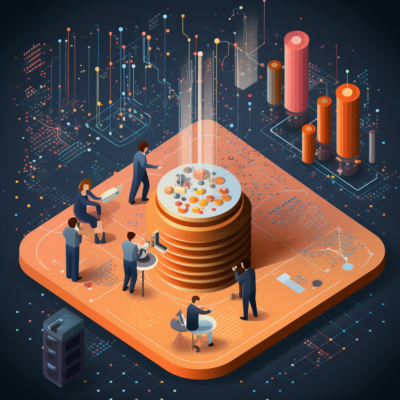
Analytics and AI Strategy Guide
Unfortunately, even in 2023, we're still seeing analytics leaders struggle to deliver measurable returns on analytics investments. To deliver value on your analytics and AI efforts, D&A leaders must start with a robust strategy, and we can help you get started.
The Components of the Framework
These five components are the elements of the framework that we use to map how analytics get done in your firm. They are not necessarily linear steps and might be more of a continuous circle with some overlap, but we make them distinct and look at them one by one to make the task of mapping and improving your analytics operating model easier.
Opportunity
The application of analytics in business is done to improve business, but often there are more areas to improve than time in the day or talent to find out how to improve them. Factor in that even data savvy firms sometimes don’t make data smart decisions or don’t make the changes to the business process needed to capitalize on these decisions and the reality is that it can be near impossible to understand why a given opportunity was chosen over another.
In the context of an analytics operating model mapping and improvement effort, the goal is to see how opportunities are identified across the enterprise and if a formal structure for identifying them exists, how to judge its effectiveness. (See “Prioritizing Analytics Efforts: A Framework” in sidebar).
Data
Data challenges, including access to data, understanding its elements, and trusting its veracity are always the top challenges of firms as they work to improve their analytics. For this reason it’s no surprise that entire functions are dedicated to this effort in firms, entire companies are dedicated to helping firms do this better and a Data Strategy is a critical component of an analytics effort. In the context of an analytics operating model mapping and improvement effort, we evaluate the data part of the model at a very high level and somewhat subjectively so that we can understand how big of a challenge it is. It’s important not to assume that all of your analytics challenges are data failures and equally important to accept that many data challenges will need to be addressed. Data, unlike most other elements in this framework can impact both effectiveness and efficiency dramatically.
Models
Whether or not the right models are chosen to address the defined opportunities in the data landscape of the firm is an important question in the mapping of the operating model. Over the long term, better matching of the models, including choosing ones with the right level of sophistication (where more is not necessarily better) will help increase the number of analytics experiments that become running analytics products, so also, over the long-term increase efficiency by reducing the number of ‘failed’ proofs of concept. The downside, of course could be a reduction in innovation, but that can be managed in an effective identification of the opportunities in that component of the operating model by making explicit that innovation and experimentation are a desired outcome, even if they yield lower immediate economic benefits.
Deploy/Fix/Kill
The decision to deploy a model or fix it or kill it is a fairly detailed one and can be quite technical. For the best approach on how to do this see the “Analytics Application Lifecycle Framework” in sidebar. In the context of mapping the analytics operating model the goal is to see that some agreed criteria are used, and that the decision is connected to other elements of the operating model, and the decision is fed back into the operating model. For example, if the reason to kill the model was that the underlying data was suitable in test but not in scaling the model in production there should be a method to bring that new understanding back to the data component of the operating model.
Manage
All models will decay in effectiveness as a result of changes in business conditions, shifts in the relative value of other models, and in changes in data and analytics techniques that make new models potentially more powerful. However, dependency on a given model can mean that functions or persons cannot objectively assess a model’s value, and this can lead to a situation where models are only added and never taken away, which increases cost (labor and data storage) and decreases efficiency or impedes the introduction of new models.
Stay tuned for Part 2 where we look at a scorecard system for evaluation and an example use case.