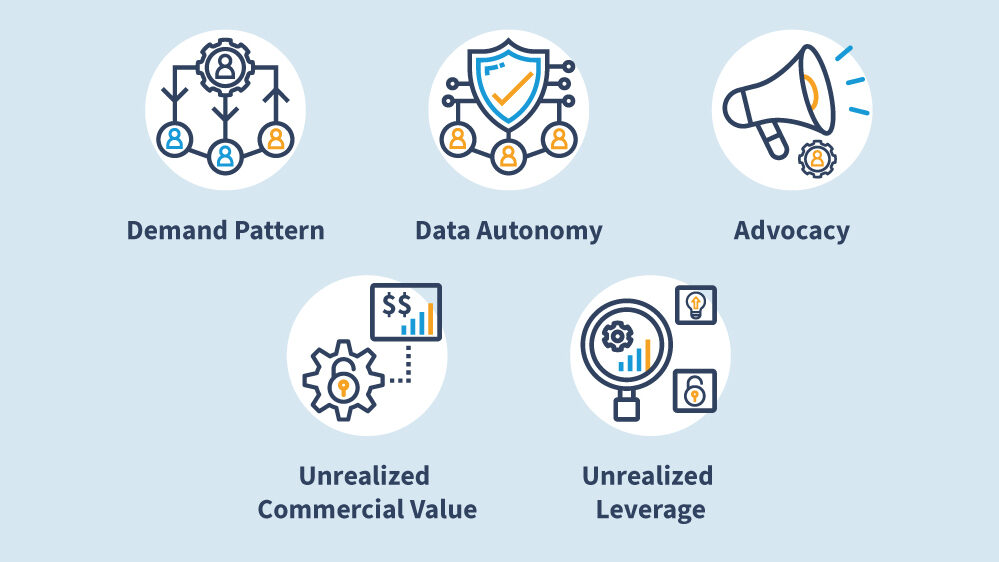
Every organization has an information economy with a supply-side that provides data and tooling to analysts and a demand-side that consumes data and analyses to make decisions (see sidebar for blog series on your firm’s information economy and the balancing act between supply and demand). Analytics and AI programs require an information economy oriented to the demand. This means moving away from what is highly likely to be a supply-controller, command-oriented information economy, driven by IT, to instead be an information economy where demand and business needs trumps supply.
However, in transitioning to a demand orientation, analytics leaders will quickly become aware that the demand-side is multifaceted and that no two data consumer groups are the same, creating complexity when trying to develop demand-based analytics strategies. They will find that demand segments need different things, have different abilities to articulate those needs, require different kinds of attention from the core analytics team and have different kinds of advocacy to bring to the table.
For example, if you have a particularly vocal and politically powerful constituency in the organization, say, sales and marketing, that feel they’re woefully underserved by the analytics and AI team, the fact that that this team has done a great job serving quiet, less powerful constituencies in supply chain and manufacturing will not ultimately be of benefit. When the next funding cycle occurs and the analytics team shares its successes working with the quiet groups, it can expect to be met with, “Yes, but the sales and marketing people say you’re not adding any value.”
If you read no further, remember this: Segmenting your demand is necessary not only because needs and requirements vary across constituents but also because expectations and advocacy differ. The primary job of the analytics team is to meet the needs and expectations of the demand side and effectively leverage advocacy for success.
This blog series is intended to help data and analytics leaders identify how their team is orienting toward data consumers and provide a framework for evaluating the critical dimensions of a demand segment, such as human resources or commercial. In this discussion, we assume that a centralized analytics team exists, but the insights here are applicable to any team structure.
In part 1, we’ll explore how your team might orient toward demand and five critical dimensions of a demand segment, ranging from demand patterns to advocacy. In part 2 we’ll finish out the framework and give you a method for scoring the process.
Supplier Orientation
The move toward demand-driven analytics creates a significant problem: traditional supply-side players, such as IT, have historically not thought about identifying, managing, and responding to demand as they are preoccupied with their substantial run and maintain burden. So, they tend to corral demand. They discipline demand, and they limit it. They try to control demand rather than position themselves as the response-oriented suppliers that consumers expect.
In general, we’ve observed that analytics suppliers orient themselves to demand in three ways:
- Authoritarian suppliers: They operate under the premise that if they build it, users will come. They believe they are in the best position to determine what users should want and how they should want it. The information economies of companies transitioning to advanced analytics and AI regard this class of suppliers with suspicion and seek alternative solutions for what they regard as supplier failures.
- Naïve suppliers: They supply exactly what consumers say they want, producing to the stated requirements with a minimal amount of investigation. These suppliers become swamped with requests, many of which end up being slight variations of requirements already fulfilled. In addition, naïve suppliers often contend with disappointment because consumers nearly always refine what they need once they see what’s been provided.
- Demand-driven suppliers: They see their roles as not only being good listeners and rapid responders, but also demand engineers who help consumers identify what they need and what would benefit them, given a particular business need, even when they can’t see this for themselves.
Ultimately, demand-driven suppliers think of themselves as consultants. There’s a give-and-take dialogue between demand-side constituents and analytics and AI practitioners that tends to produce high-value, collaborative relationships that return better outcomes for the demand side and burnish the reputation of the analytics suppliers. To this end, demand-driven practices produce value for delivering against real business needs and data consumer advocates who champion the analytics team. This is an ideal state for those who supply and consume the analytics—and of course for enterprise operations.
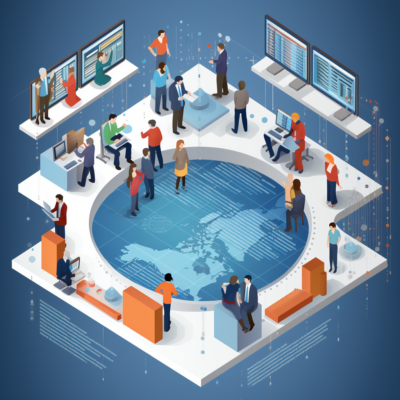
Segmenting Your Analytics Demand eBook
In transitioning to a demand orientation, analytics leaders will quickly become aware that the demand side is multifaceted and that no two demand constituent groups are the same, creating complexity when trying to develop demand-based analytics strategies. To ameliorate this, experts at IIA have identified the 10 critical dimensions to a segment and explained them in this eBook, allowing you to better understand where your current demand segments are and where your target demand segments should sit.
The Segments
In larger companies, analytics and AI practitioners often segment their data consumers by function (e.g., human resources) or by lines of business (e.g., commercial, residential). Any segmentation schema is ultimately fine if it identifies discrete groups with unique objectives, needs and capabilities, and you can understand each group’s advocacy value: the extent to which a given group—or segment—will help the analytics team engage with, penetrate and successfully supply other segments.
Demand Pattern
How does a segment’s pattern of demand appear to the analytics team?
On one end of the spectrum, there's “push,” involving hard-selling analytics capabilities to coax or force a segment into working with the team, sometimes resulting in avoidance or circumvention. On the other end is “pull,” where a segment insists on the team's involvement in all analytics-related activities, risking resource constraint. Assessing segments between push and pull helps prioritize efforts. Transitioning “push” segments to neutrality is job one.
Analytics Capability
How much analytical competency does a segment have in its own right?
Functions and business units accustomed to the traditional supply-driven paradigm often have no local analytics capabilities and can cause a significant resource drain for the analytics team. To mitigate the resource drain, migrate lower value-added analytics competencies—such as descriptive BI—to the demand segment via self-service tools. Upskilling segments so they are self-sufficient and can migrate from consuming reports to producing analyses themselves will allow the analytics team to focus on higher value-added advanced analytics and AI opportunities.
Data Autonomy
How autonomous is a segment in terms of its ability to source and manipulate the data it requires for operational reporting, BI, and advanced analytics?
Organizations with well-integrated analytics platforms and easy data access usually produce largely self-sufficient demand-side segments when searching for, sourcing, and integrating data. The ease with which a motivated demand-side constituency can perform these activities is an important measurement of the effectiveness of the data and analytics platform, its tooling, and the data supply. Even with a low-impedance platform, achieving data autonomy in a segment can be challenging, hindering progress toward optimal analytics autonomy, the next dimension.
Analytics Autonomy
How autonomous does a segment want to become in the future?
The analytics capability dimension assesses the current state, while this dimension gauges a segment’s desire for autonomy. Alongside enhancing a segment’s analytics capability, teams should boost each segment’s inclination to conduct more of its analytics. Self-sufficient demand-side groups mean more resources for advanced analytics and AI. However, it’s crucial not to push demand-side autonomy beyond manageable levels. For instance, avoid encouraging segments to import third-party data into the common analytical platform without proper tooling for managing it. Similarly, hold off on urging them to develop complex applications until processes are established for managing distributed development across their life cycles.
Advocacy
Is a segment an advocate for the analytics teams and its capabilities?
Can the segment become an advocate? If the segment practiced advocacy behavior, would it be of significant value to the analytics team?
In general, mature analytics teams help influential segments get their voices heard, so that the teams can leverage that advocacy when the time comes to argue for additional resources, budget, and scope of control.
When evaluating advocacy, consider these two things:
- Is the segment a net detractor? If so—and if the segment’s opinion will shape the opinion of other segments—as a top priority, develop a plan to advance the segment to a neutral posture, or better.
- Does the segment’s advocacy matter? For example, an analytics team may have advocate within the manufacturing, distribution and supply-chain segments of its portfolio, but if the enterprise heavily weights the opinions of sales and marketing, that’s a challenge the analytics team must address.
Stay tuned for part two, where we explore dimensions like unrealized value and resource drain, and a scoring system to transition from current states to target states within a demand segment.