
AI in the Future Workplace
Artificial algorithms and platforms are great equalizers. They work for large and small enterprises alike; they work for both novice and experienced analysts the same. Artificial intelligence supplements a lot of human judgment and knowledge, and levels the playing field between the workers in a given domain.
To Share or Not-to-Share
Organization Point of View
Usually, a development team collaborates with one or more subject matter experts (SMEs) who excel in a particular area of the business to create an Artificial Intelligence (AI) system or algorithm that addresses a specific organizational problem. The SMEs help the development team integrate various business nuances into the AI models, making them more sophisticated and effective. According to research, 87% of development teams involve SMEs in the AI system creation process, highlighting the significance of their expertise in developing effective solutions.
Once the system or platform is implemented, it can empower not only the SMEs but also all team members in the business unit and possibly beyond. The expertise and knowledge that previously resided with only a few SMEs is now accessible to low-performing team members as well, democratizing information across the organization.
When evaluating team performance, management expects low-performers to improve their performance after the system's implementation. However, it may leave one wondering what benefits the high-performing SMEs gain from the process.
Industry Point of View
If we take a broader perspective and look at the current AI development ecosystem, it becomes apparent that a handful of organizations possess a significant portion of the world's data and computing power. These data-rich organizations are at the forefront of developing AI solutions by utilizing the data they have collected. These systems then become a source of competitive advantage and revenue for these organizations. For example, the top tech giants such as Google, Amazon, Microsoft, and Facebook collectively held vast amounts of data, with Google processing over 3.5 billion searches per day and Facebook having more than 2.8 billion monthly active users.
Unless these organizations adopt a service-oriented (SaaS or PaaS) revenue model, they typically do not seek to share their models for the benefit of others. According to a survey conducted by AI Singapore, only 35% of organizations are currently willing to share their AI models with external parties, primarily due to concerns related to intellectual property and competitive advantage.
Both of these thought processes presents unique challenges to incentivize both the producers (read developers) and consumers (read users) of an AI system simultaneously, due to the innate competitive advantage that a well-designed AI system presents to its creator.
Evolution of “Strong” AI
“Strong” AI systems are best described as omniscient human-like intelligence capable of performing more than a few narrow/specific tasks. Of course, no such system exists in today’s world. However, in the past decade big strides have been made in making the traditional “weak AI” much “stronger.”
“Weak AI” refers to intelligent systems trained to do very specific things. Some examples include systems that analyze medical images and detect cancer or play a song on voice command or drive a car on an urban highway – or even forecast the market size of a drug in next five years. Since these systems do not have the knowledge of performing any other tasks outside its domain, they can’t be qualified as “strong” AI.
Developing Incentives for “Stronger” Intelligence Systems
In the ever-evolving landscape of artificial intelligence, organizations and industries have been trying to figure out ways to collaborate and cooperate to resolve the incentive conflicts mentioned above. Some of the recommended ways are as follows:
Intra-Organization Incentives
Strategic Alignment on Future-looking Business Goals
The failure to successfully build or expand an intelligent business system is often attributed to a lack of alignment between the objectives of the business and those of the technology teams. When the business is solely focused on tracking quarterly figures and the technology team takes on the responsibility of creating an intelligent system to support or automate crucial business processes, the result is an elegant system that fails to meet the business's requirements and lacks the necessary adoption to generate value.
A crucial factor in establishing an intelligent ecosystem lies in clearly articulating the specific business value that this system should deliver and obtaining the endorsement of the business team for this initiative. Effective persuasion and clear communication at the senior management level, along with aligned incentives, are essential in driving home this message.
In my personal experience, forward-thinking organizations who are relying on tech and AI systems to give them a competitive advantage over their peers, call out clear technological aspirations (often linked to business value) in their annual or 5-year roadmap.
Reward and Incentives for High-Performers
Organizations that view technology adoption and enablement as a growth and competitive advantage strategy implement various reward structures to cultivate the necessary skills among their top performers.
Frequently, candidates who possess expertise in technology-integrated business functions are prioritized for promotions and rewards. This emphasis ensures that individuals with relevant knowledge and experience are recognized and incentivized within the organization.
Furthermore, employees are actively encouraged to engage in projects that go beyond their regular responsibilities, enabling them to acquire cross-functional and technology-oriented skills. This approach promotes a culture of continuous learning and skill development throughout the workforce.
To foster innovation and skill enhancement, top-performing employees are allocated a percentage of their working time to incubate, experiment, and pursue ideas. This dedicated time allows them to upskill themselves while also exploring potential revenue streams for the company. By providing this opportunity, organizations foster a culture of creativity and entrepreneurial thinking among their employees.
For example, Google is known for its "20% time" policy, where employees are encouraged to spend 20% of their working hours on projects of personal interest. This initiative has resulted in the creation of innovative products and services such as Gmail and Google Maps, which have not only enhanced employee skills but also generated significant value for the company.
Drawing from prior experience, allowing high-performers the flexibility to set their own “moonshot” goals (aside from BAU metrics) as a part of their annual goals, encourages and motivates them to innovate for the next horizon.
Inter-Organization Incentives
Productization and Monetization Tactics
Creating an AI product for a narrow audience may not be a sustainable business strategy. However, by focusing on productization and collaborating with high-performing experts, it is possible to design a product that is relevant to a larger audience. This approach also provides an opportunity for these experts to gain new skills in AI-augmentation and product development.
For service-oriented organizations, monetizing the product across a new customer base and sharing the benefits with the high-performers can foster enthusiasm and a sense of ownership among team members.
One real-life example of this strategy can be seen with Amazon's Echo device. Initially, the device was designed as a smart speaker with voice assistant capabilities, aimed at a narrow audience of early adopters. However, by productizing the device and collaborating with high-performing experts in fields such as home automation and entertainment, Amazon was able to create a product that was relevant to a much larger audience. They also offered opportunities for third-party developers to create "skills" or custom applications for the device, further expanding its capabilities and appeal.
Another example can be seen with the collaboration between Ford and Microsoft on the development of the Ford GT supercar. Ford utilized Microsoft's expertise in data analytics and machine learning to improve the car's performance and reliability, while Microsoft gained valuable experience in applying their technologies to the automotive industry.
Or to take an example from first-hand experience, development of a medical imaging or Karyotype-identification algorithm can be beneficial to a life sciences firm for its own use. However, productizing the features generic to the industry and marketing to peers allows you to better monetize the efforts.
Data Access Through Partnerships
Data-rich organizations often hold a significant advantage over their competitors due to their ability to collect and analyze large amounts of data. However, collaboration with organizations that are still catching up can provide mutual benefits.
Google and DeepMind: Google is one of the world's largest data-rich organizations, with access to vast amounts of data collected from its search engine and other products. In 2015, Google acquired DeepMind, a UK-based AI research company that had developed advanced techniques in reinforcement learning. This acquisition allowed Google to incorporate DeepMind's expertise into its AI systems, while also providing DeepMind with access to Google's vast resources and data sets.
Microsoft and OpenAI: In 2019, Microsoft announced a partnership with OpenAI, a research organization focused on developing advanced AI algorithms. As part of this partnership, Microsoft agreed to invest $1 billion in OpenAI, and the two organizations agreed to collaborate on the development of new AI technologies. This partnership allows Microsoft to gain access to OpenAI's expertise in advanced AI algorithms, while also providing OpenAI with access to Microsoft's Azure cloud computing platform.
In another example, a data-rich organization in the healthcare industry may collaborate with a smaller organization that has limited access to data but has expertise in a particular field. By combining their resources, they can develop an AI system that leverages the data-rich organization's data and the smaller organization's expertise. This collaboration can result in a more accurate and efficient AI system, benefiting both organizations.
Removing Bias and Improving Consistency
Collaboration with other firms is essential for enhancing even the most basic AI systems, as it allows for the identification and correction of blind spots using data and techniques from multiple sources. Improved collaboration can result in reduced bias, discrimination, and errors in these models, leading to increased reliability and consistency.
One real-life example of increased collaboration leading to reduced bias in AI systems can be seen in the partnership between Google and the Harvard Global Health Institute. The two organizations collaborated to create an AI-powered tool to identify the location of impoverished areas that lacked access to safe water sources. The tool relied on satellite imagery and machine learning algorithms to identify potential water sources.
During the development of the tool, the team realized that the algorithms were not accurately identifying areas with water scarcity in certain regions of the world, particularly in rural areas. To address this issue, the team collaborated with local organizations and experts to gather ground-level data on water availability and quality. This data was then used to train the machine learning algorithms to better identify areas in need of safe water sources.
Conclusion
The success of an AI product or algorithm is often determined more by incentivizing the right stakeholders and ensuring the alignment of business and technological aspects rather than solely relying on the utilization of appropriate technology. To gain support within an organization, it is crucial for CXOs and their business goals to endorse large-scale and strategic AI interventions, while also implementing incentive structures to engage key high-performing personnel and secure their commitment to these initiatives. To foster ecosystem support, analytics leaders should extend their focus beyond organizational needs and cultivate mutually beneficial relationships with potential customers of the solution, as well as data aggregators and AI-expert firms who can provide valuable assistance in enhancing the AI system.
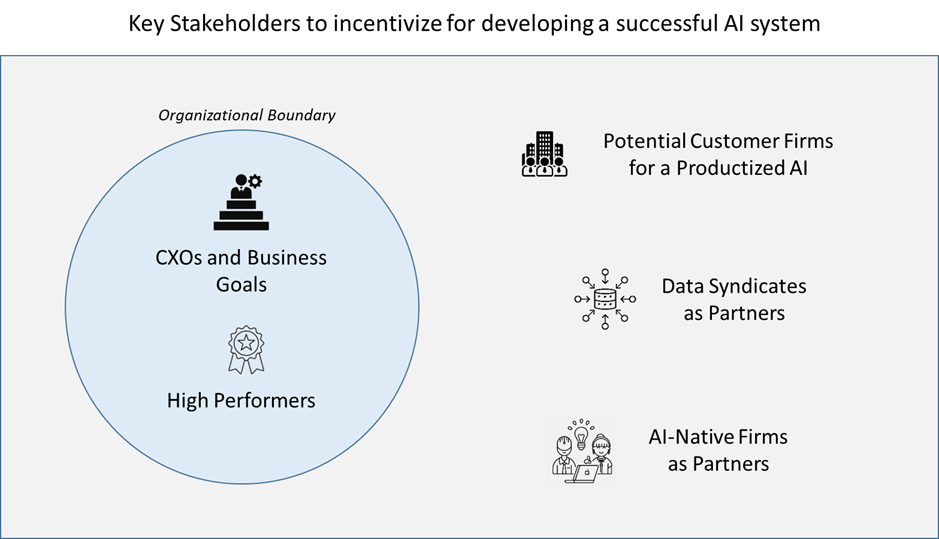
The rapid advancement of the AI industry in recent years has brought about significant changes in engagement and business models across all sectors. To keep up with these changes, organizations need to ensure they are properly aligned and incentivize high-performers while developing strategic partnerships to collaborate across domains. This is crucial for the long-term success and sustainability of businesses that seek to leverage AI technology.
In our next blog, we’ll explore the challenges and opportunities of social AI.