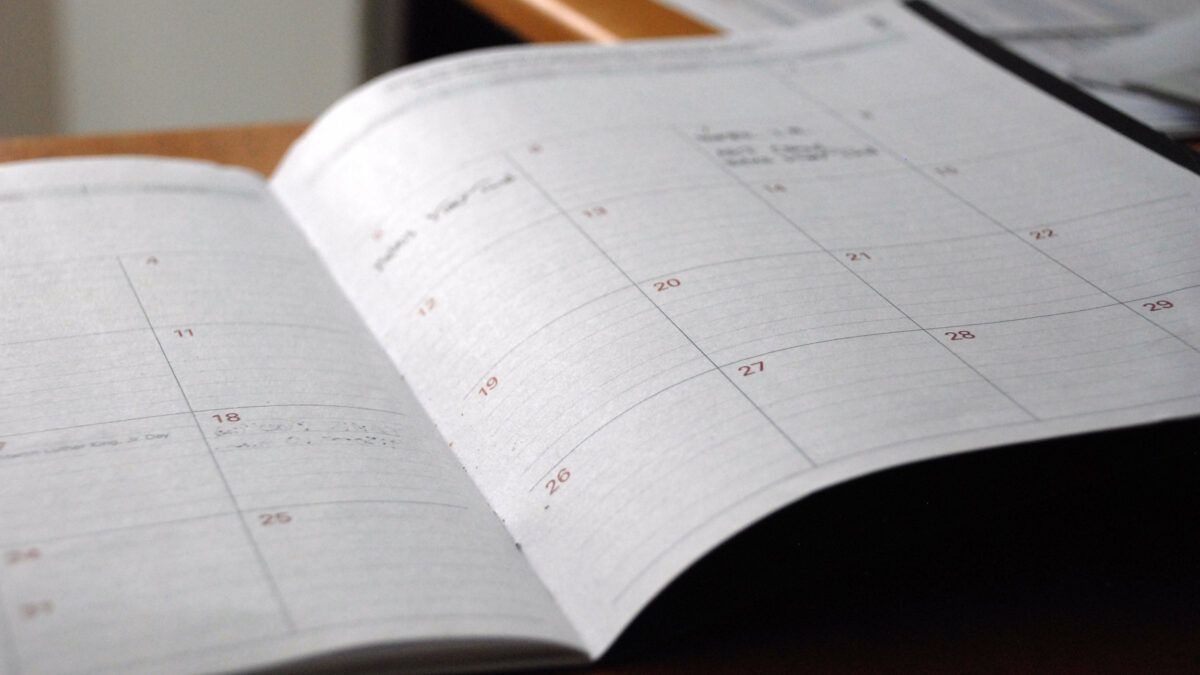

Certainly, it is important to have analytics available in the timeframe needed for making decisions. For many years, it was too difficult and expensive to execute analytics anywhere near real-time and so everything was done using infrequent batch processes. As processing power has increased exponentially and costs have dropped to unprecedented levels, it is feasible to perform a wide array of enterprise analytics on a near real-time basis. However, many organizations today are vastly over-utilizing real-time analytics and are paying a price for it that, unfortunately, isn’t always recognized.
FORGET REAL TIME. FOCUS ON DECISION TIME!
Naturally, I am a proponent of ensuring that business decisions are made in a timely fashion. However, many decisions do not need to be made in anything approaching real- time. Just because something canbe analyzed in real-time does not mean that it should be analyzed in real-time. Creating real-time processes where they aren’t needed leads to a lot of additional complexity and cost. Careful consideration is needed to decide how fast or frequently a given analytics process should run.
The first step is to identify the true speed required to meet business needs. I like to call this required speed “Decision Time”. While you might be able to make a decision faster than Decision Time, there is typically no benefit in doing so. An early decision will simply be held until it is needed. Contrary to the hype, there are surprisingly many cases in the real world where real-time speed is not needed. Here are some examples that illustrate different Decision Times:
Milliseconds/real-time. Optimizing ads on a website prior to the next page loading
Seconds. A predictive maintenance algorithm identifying if an abnormal situation is present in a car’s engine and suggesting a corrective action
Minutes. A courier approaching a package pickup needs the optimal delivery route, taking into account additional pickups that might be needed along the way
Hours. An AI process to detect cancer in a brain scan must be completed for the doctor before he returns in the morning
Days. A ship will arrive end of week, so analytics must decide which distribution centers are sent what percentage of the shipment given anticipated local demand and inventory levels
Weeks. Analytics must determine if a US tax return is fraudulent before paying a refund in several weeks
Indefinite. Particle accelerator data must be analyzed to determine if an experiment was successful in creating a new particle
WHY OVER-USE OF REAL-TIME ANALYTICS CAN LEAD TO EXCESS COST
Of the prior examples, only one requires real-time analytics. Why does engineering a solution to support real-time analytics have the potential to cost so much? Let’s explore the example of detecting tax fraud to illustrate.
The IRS typically pays refunds several weeks after submission of a return. Sure, fraud analytics could run within milliseconds of submission. But, the typical pattern for the IRS is that there will be a massive spike of activity on the mid-April due date, with very high activity the few days before and after. A steady increase of activity will occur in the weeks prior to the due date as well. For the remainder of the year there will be, relatively speaking, no submissions to handle.
A system to handle returns in real-time will have to be built to support the huge influx of returns on the peak days and also all of the associated analytics. This will result in a system sized such that it will have massive unneeded capacity most of the year. That’s expensive! Even if the solution is deployed on the cloud, it will still require lots of temporary capacity, which will cost more than a committed base level of usage. In addition, since the analytics must be guaranteed to complete in real-time, a range of redundancies and contingency plans will have to be made in case the primary systems go down, adding even more cost and complexity.
Alternatively, returns can be accepted during peak times with none of the analytics completed until later. Once the rush is over, there are still days or weeks to work through the returns and run the fraud analytics. An internal system of much smaller size can handle this processing over a period of time. Or, a cloud-based system can handle it within typical committed capacity. Overall, it is less complex, cheaper, and just as effective to execute the analytics on a delayed basis. And, all analytics will still be completed within the required Decision Time window.
ALWAYS BUILD TO THE REQUIRED DECISION TIME
Business owners may be enamored with the idea of building real-time analytics. Many analytics professionals are too. However, as analytic professionals it is upon us to meet business needs while also being good stewards of resources. If we understand when a decision is needed, and analytics processes are built to meet that need, business owners will be thrilled. They may ask, “Is this running in real-time”? A simple response is, “It is running as close to real-time as we need it to”. That’s really all that matters. The savings compared to the cost to make the process real-time can then be put toward an additional analytics effort targeted for completion in Decision Time. That will make everyone happy.