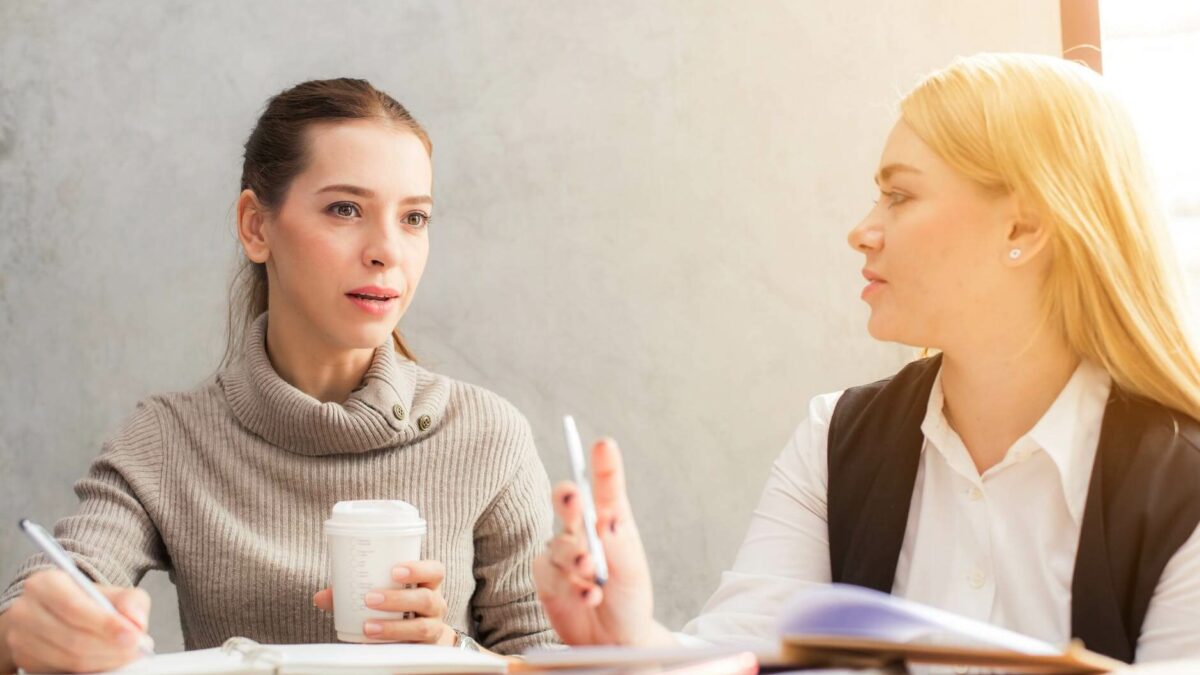
When I talk with analytical professionals about artificial intelligence, many are reluctant to claim expertise in the area or bill themselves as AI practitioners. “I don’t want to appear to be just jumping on the bandwagon,” one told me recently. They may see the enthusiasm for AI as somewhat faddish, and don’t want to exaggerate their own capabilities. Or they may not realize that the expertise they do have is highly relevant to AI.
I understand this reluctance, having experienced it myself with a previous switch in analytical eras. When the concept of “big data” started to take off in 2012 or so, I initially resisted using it. I had a big investment in the concept and term “analytics,” and thought that big data wasn’t really that different from it. I also didn’t want to give in to the faddistry of the contemporary business environment.
But I made a mistake in resisting—resistance to the Borg of business fads is futile—and should have begun writing and speaking about big data earlier than I did. Eventually I concluded that I should do some research on the phenomenon, and Randy Bean, Paul Barth and I wrote about “How Big Data Is Different” in 2012. Jill Dyche (then of SAS) and I investigated “big data in big companies” in 2013. We found that there were many similarities to “traditional” analytics, but some key differences as well. Eventually I incorporated big data into the overall stream of analytics thinking into what I called “Analytics 3.0.” I could have been faster about embracing big data, but at least I eventually did it.
There are also a lot of analytics in AI (also referred to by me, if not many others, as “Analytics 4.0,”) which I found when I started researching, speaking, and writing about it around 2015. You may have noticed that much of the world is now excited about AI rather than either big data or analytics. And as with big data, much of AI has analytics at its core. Machine learning in its simplest form is predictive analytics. Yes, it sometimes uses some very complex models, but it also often uses logistical and even linear regression. If you are an analytics person you are familiar with at least the simpler forms of machine learning.
So here’s what you should do. First, revise your resume, LinkedIn profile, etc. Instead of saying that you do “predictive analytics,” say you do “supervised machine learning” or something similar. The terminological substitution has the virtue of being true, and it will make you more desirable in the marketplace. You could even ask for a raise!
Similarly, if you run an analytics group for your company, you want to start billing it as an “Analytics and AI” group, or at least an “Analytics and Machine Learning” group. If you don’t claim AI expertise, your company will look elsewhere for it—perhaps to people who have no more expertise than you do.
Second, just as I had to do some research to find out more about big data (and then AI when I made that transition), you may need to do some research about forms of machine learning and AI with which you are unfamiliar. Maybe you need to try to understand and use some forms of machine learning with which you are unfamiliar, such as “gradient boosted trees” or the hot deep learning models. At their core they are all variations on statistical modeling—fitting lines to data.
Given advances in automated machine learning, you probably won’t have to learn as much about these modeling approaches as you would have in the past. If you understand how they work in general, AutoML tools can fit the models to your data and determine if they offer greater predictive power in a given situation. It’s most important for you to know what the assumptions are behind the models and what kind of data they work best with. Knowing how to describe them in common English terms is also very helpful—just in case one of your internal customers asks.
If you’re going to portray yourself as an AI expert in general, you may also want to learn about some of the approaches to it that are not fundamentally statistical in nature. Semantics-based natural language processing (NLP) has been around for a while, as have rule-based systems. These approaches are not in favor at the moment, but they do have advantages for certain types of applications. They’re still used by many companies. You don’t need to go back to school for a degree in computational linguistics or symbolic logic to understand them, but reading up on them would probably be useful.
Regardless of how much or how little change there is in the underlying methods, there will always be evolution in how the world describes our profession of making sense of data. The only people who suffer from these conceptual changes will be those who refuse to learn about and embrace them. It’s actually wonderful that a basic understanding of statistics can make one, over time, a decision support, business intelligence, analytics, big data, and AI expert—all while remaining the same individual!