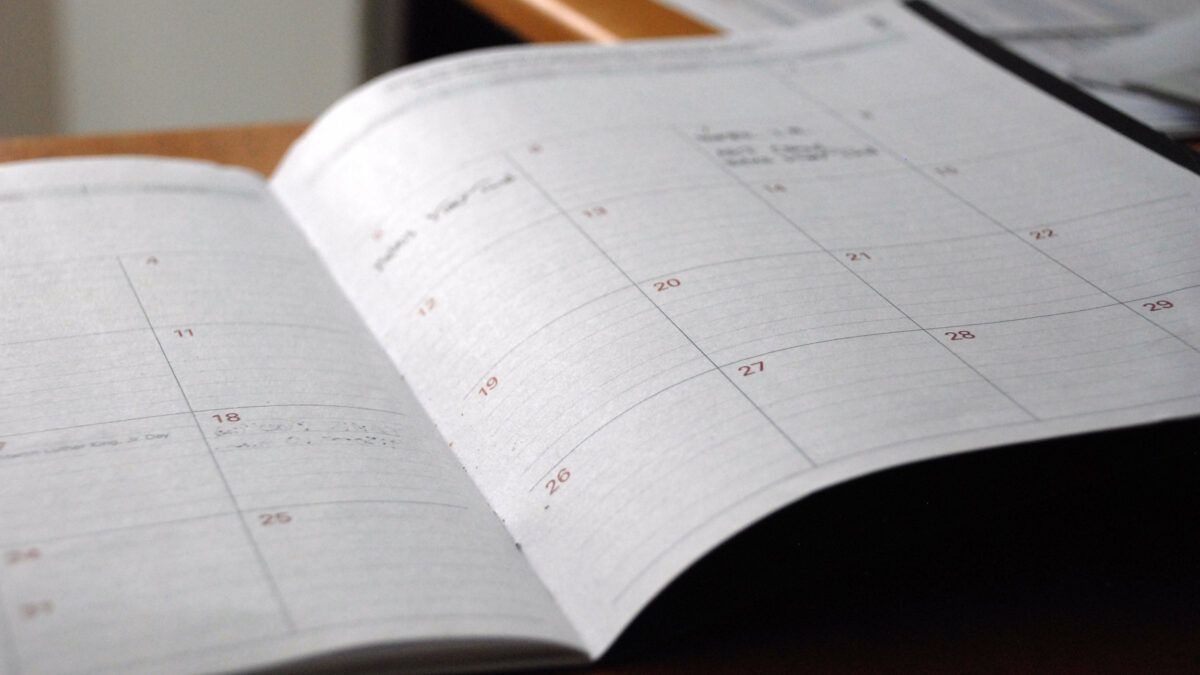
Historically, most analytics have had laser focus on specific entities like a customer, a product, a vendor, or a variety of others. When performing analysis, the focus is usually purely based upon facts about each entity. For example, each customer’s individual spend, frequency, and demographics. While such analytics have proven quite valuable, they usually don’t account for the relationships between entities and the nature of those relationships.
This is where connection analytics (often called graph analysis) comes into play. Connection analytics is best known for its use in social network analysis, which is commonly used to explore the relationships between people within social media environments. However, connection analytics can be used for a much broader range of purposes that aren’t often given the credit deserved. After all, there are myriad situations where understanding relationships can provide meaningful insights. A few include:
The famous approach of taking into account the fact that members of a calling circle have a greatly increased risk of churn as other members of the circle defect to a different telecom service provider.
A variation on that example is for human resources to study the relationships between employees as evidenced by email communications to enable appropriate retention actions when an associate resigns.
Compliance officers and law enforcement can explore the patterns of communications and transactions to uncover fraudulent or otherwise suspicious activity between people or organizations.
Network engineers can explore the communications between various sensors to determine when network traffic is taking unexpected routes that may be caused by trouble with certain pieces of equipment.
Marketers can dive more deeply into the indirect linkages between products or product groups to come up with better cross- and up-sell opportunities.
As the examples illustrate, there is broad applicability of connection analytics. However, most organizations have not yet added it to their analytics arsenal.
This is a mistake.
Part of what makes the analysis of connections so powerful is that while virtually every metric typically used for analysis focuses only on facts about each individual entity, the analysis of connections makes it possible to also understand each entity’s relationships to others. The analysis of connections provides distinctive information that has very little overlap with other information typically available.
Of course, analyzing connections on a large scale is a computationally intensive process. To be effective, it is necessary to implement a graph analysis engine. One recent and strong entrant into this area is the Teradata Aster SQL-GR graph engine. This engine allows not just scalable graph analytics to be generated, but also makes it easy to combine graph analytics with a broad range of other analytics. This is important because analyzing connections is rarely all that is needed. Usually multiple types of analysis combined will yield the best results.
The concept of combining multiple types of analysis is very important. In the telecom churn example, service providers don’t react based only upon who is connected to a defecting customer. They also take into account the other factors they know about each customer to determine the risk of churn. For example, customers with longer tenure, multiple services, and multiple sub-accounts will be less likely to churn than newer customers with only a single, basic service. This will still hold as a customer’s connections defect. The power is in the cumulative effect of all of the information being combined together.
While connection analytics won’t solve all of your organization’s problems, it can probably help solve some of them better. Given that it isn’t widely adopted yet, there is a chance to get a competitive advantage by putting it to use first. Ignore connection analytics at your own peril!