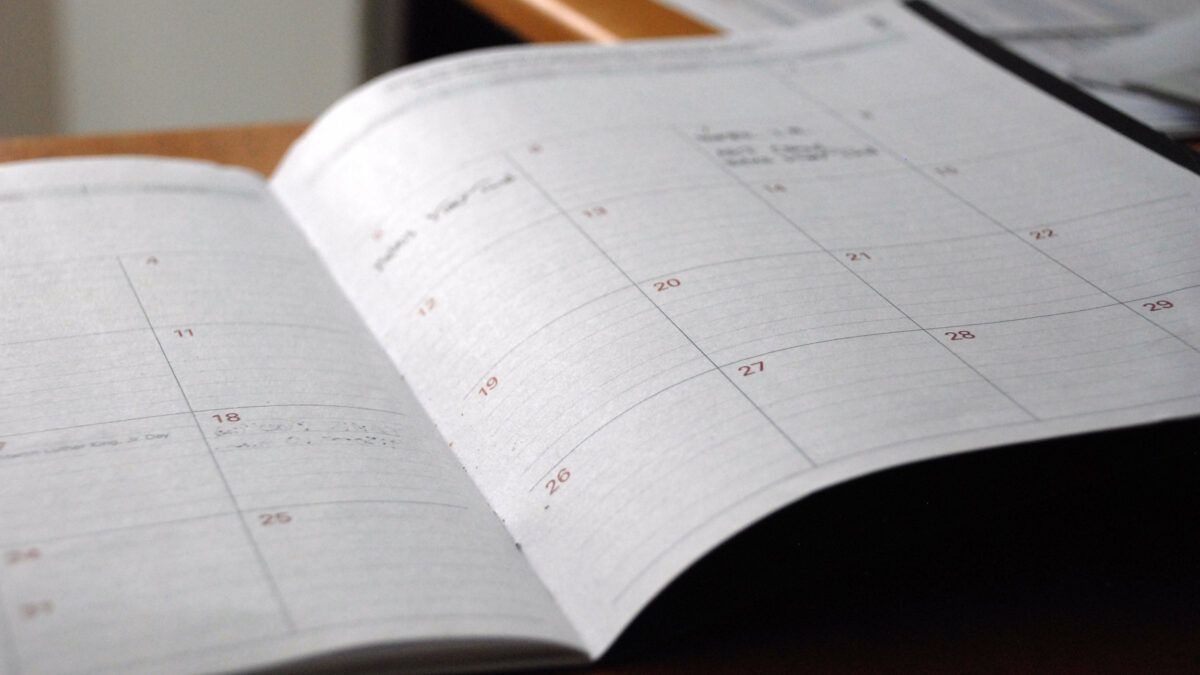
Analytics and big data have penetrated most large organizations by now, and are helping to improve many internal decisions. But they can also have a major impact on the decisions of customers or citizens. This applies not only to decisions about what products to buy, but also to decisions about safety and crime.
I’ve previously written here about the ever-advancing efforts of the New York Police Department in using analytics, which began over a decade ago. The NYPD’s CompStat systems were intended to inform internal decisions about where to focus resources and mobilize personnel to deter and arrest criminals.
But there are other analytical frontiers in the battle against crime, and they’re being adopted all over the world—including in Rio de Janeiro, which has a substantial need for them (as does Brazil in general, with roughly 60,000 homicides a year— more than any other country on the planet.)
You may recall the recent Summer Olympics in Rio, in which there was no highly visible crime (unless you count the run-in that the swimmer Ryan Lochte had with the police). But Rio has a long-term problem with crime, and the period before and during the Olympics saw a surge in it. One key aspect of the approach in Rio involves pushing crime data out to citizens.
Robert Muggah, a Canadian political scientist and security expert who lives in Rio, has co-founded the nonprofit Igarapé Institute to work with the state police in Rio (and law enforcement agencies around the world). One of their latest projects involves the development of data-driven tools to help predict criminal activity. The institute’s efforts have not only helped the Rio police monitor and solve crimes, but also have helped Cariocas (as Rio residents are known) figure out where they are most likely to avoid and encounter crime.
There are three key areas in which the Rio crime analytics work is setting a new direction. One is the use of advanced analytical methods to predict where and at what time crimes are likely to take place. A second is the application of analytics to analyze video, audio and location data from open source body cameras used by police officers. A third is the extension of predictive, causal and visual analytics outcomes to citizens. Similar steps could be adopted by businesses and organizations in many industries.
The predictive crime-fighting project was a partnership between Igarapé, the analytics vendor Via Science (I am an advisor to the Cambridge, MA-based company), and Rio’s Institute for Public Security (ISP). The work began in May 2016. The goal was to complete a predictive model and working app by the Olympics in August.
The partners very quickly built the crime-forecasting platform. They had a head start since the Igarapé Institute and its partners were simultaneously designing a new CompStat-style crime monitoring system for ISP. As a result, the team was able to draw on more than 14 million geo-located crime incidents stretching back to 2010.
Via Science uses machine learning to build “Bayesian networks” which are well suited to analyzing risks such as the safety and security issues in urban crime. A city like Rio de Janeiro has huge variation in the location and seasonality of crime. Therefore, building many local models rather than one global model has a tremendous accuracy advantage. Via Science’s expertise in causal analytics and high performance computing (HPC) helped Igarapé develop thousands of local models quickly.
Muggah, his Igarapé colleagues, and Via Science began to experiment with different assumptions, variable weights, and algorithm changes to shape models that would predict crimes. It employed such variables as the season, location, type of incident, and so forth. In a city as large and diverse as Rio de Janiero, a “global model” of the entire city can be meaningless at best and misleading at worst. Instead, the team built literally thousands of models, each one representing a 250 square meter sector of the city. Once the model reached 85 to 90% accuracy in predicting crime in each sector, Igarapé launched a web-based app called CrimeRadar (https://rio.crimeradar.org). It was released midway through the Olympics and made it available to both police and citizens.
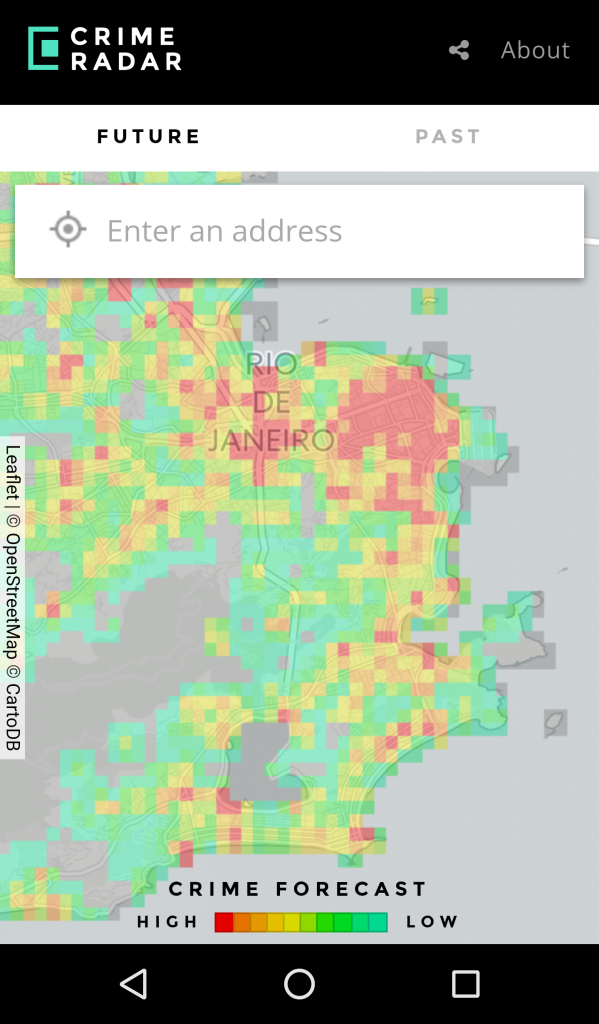
CrimeRadar is a predictive forecasting system that predicts crimes a week into the future, broken down by time of day. For each quadrant it assigns a numerical value (zero to 10) for risk, displayed in various shades of green, orange, and red. When the various quadrants are viewed in an overall picture (see “Future” screenshot below), they comprise a “heat map” of predicted crime. Igarapé worked with Mosaico, a startup incubator, to design the system and the visual analytics—always important when pushing data to customers or citizens.
If a police officer or citizen is interested in past patterns of crime, CrimeRadar also has a historical function, displaying retrospective trends in lethal violence (see “Past” screenshot below). The retrospective analysis focuses on different types of lethal violence—including straight-up murder, robberies leading to death, and police killings (unfortunately common in Rio). The app will eventually feature historical data on armed robbery, carjacking, and other serious crimes against person and property.
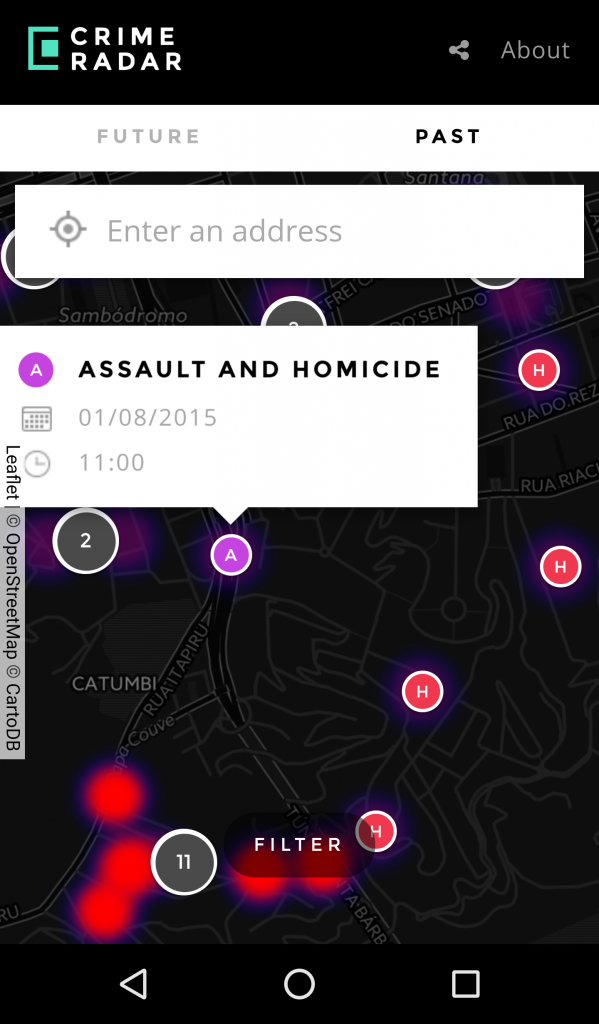
While there are other predictive policing applications in use (“CompStat 2.0” at the NYPD, for example), all of them are police-facing. But citizens benefit just as much as the police from knowing whether crimes are likely to happen in a particular area. In some cases they can simply avoid hot spots at specific times of the day and week. Of course, CrimeRadar does not claim to state exactly when and where crime will occur, which can be impossible to predict. The app includes a disclaimer encouraging users to consult multiple data sources when assessing their risk of victimization.
There are some other examples of pushing predictive applications out to customers—for example, the websites that publish the likelihood that an airline ticket price will increase or decrease over a specified time period. At some point in the near future we’ll probably also be able to predict traffic jams, delayed flights, and late shipments. If your internal analytics tell you those things are likely to happen, you should probably tell customers about it.
This article was originally published by DataInformed on December 27, 2016 and was also published on LinkedIn Pulse