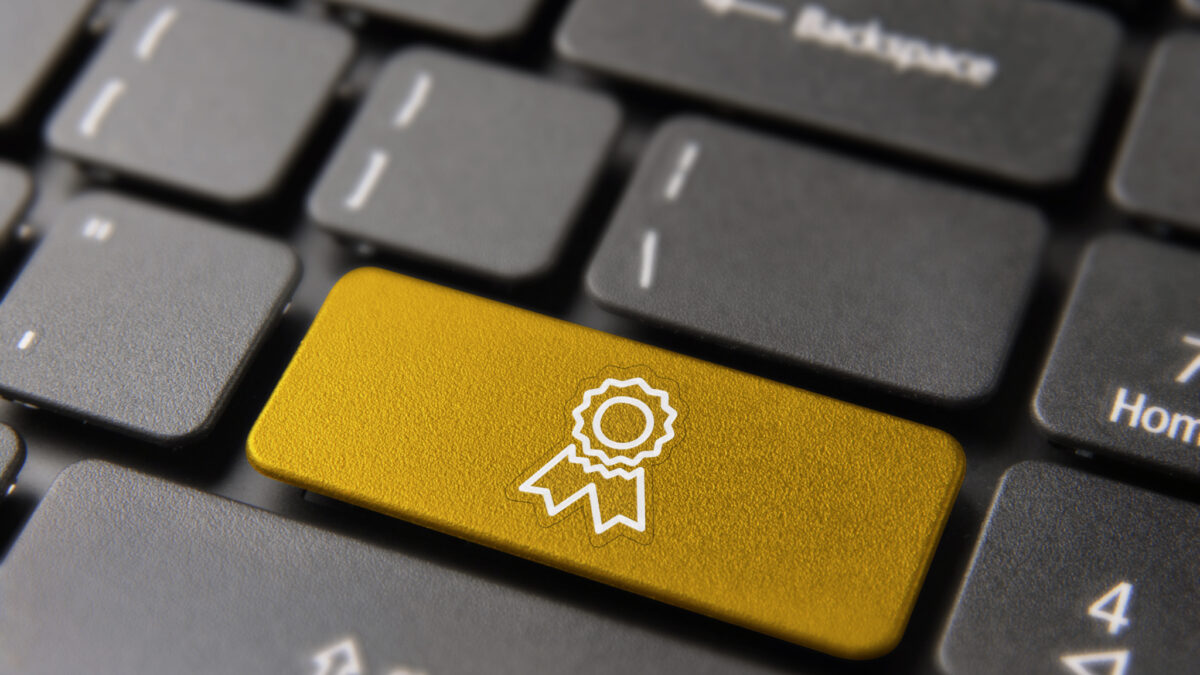
Read below for a roundup of interesting sites, resources, and articles from around the web, curated and contextualized by unbiased analytics experts at IIA. Highlights include articles about the seven stages of MLOps maturity, the rise of “small data”, low-code/no-code application development, and how to measure automation success. There is also an entertaining article on FiveThirtyEight’s NFL projection system. Follow us on Twitter and LinkedIn to receive daily updates on IIA content and curated content as it becomes available.
“Article of the Week” from IIA’s Normal Distribution
Each week, IIA’s Normal Distribution email (sent to anyone that has filled out a form on our website) features timely and relevant third-party articles. Here are the articles highlighted in the “Article of the Week” from the September Normal Distribution emails.
The Seven Stages of MLOps Maturity (Towards Data Science)
This article serves as a guide to building thorough and efficient MLOps capabilities that will maximize ROI. The seven steps discussed are:
- Accelerated Research
- Efficient Deploy
- Advanced AI Solutions
- Model Safety Nets
- Standardize, Collaborate, Streamline, Onboard
- Optimal Model Health and Risk Avoidance
- Repeatable AI Profit and Secure AI
Is “Small Data” The Next Big Thing In Data Science? (Towards Data Science)
There's been plenty of discussion over the years about the myriad of use cases and potential benefits of big data, but there's been very little talk of more "small data". This article argues that smaller datasets are necessarily going to see a rise in popularity due to the accessibility of AI and ML tools for SMEs. The author explains what the shift towards small data, and the increased importance of data quality, will mean for data scientists.
When Low-Code/No-Code Development Works — and When It Doesn’t (Harvard Business Review)
Businesses no longer need to choose between hiring a dev team or picking a software off the shelf when they need new information systems. The third option - low code applications are allowing orgs to develop bespoke software with an extremely low barrier to entry.
This article guides you through the functionality and use cases for low-code/no-code development as well as the potential management challenges with its usage.
How to Measure Automation Success for the Enterprise (Information Week)
With the many pieces of a business that automation tools can touch, measuring the effectiveness for those tools is not nearly as simple as more straightforward cost-related calculations.
This article covers steps you can take to make measuring the success of your automation efforts easier and explains exactly why it's so important to gauge automation's effectiveness.
Featured Articles on Analytics Strategy
When Data Goes Bad: How To Improve Data Quality? (Instinctools)
"Handling issues caused by bad data can cost a company from 15% to 25% of its annual revenue." The connection between data quality and efficient, sound business decisions is clear, but how can an organization go about fixing data quality issues?
This article lists common data quality issues orgs face and outlines how to ameliorate them.
The Rise of DataOps (Towards Data Science)
"Today, 75% of executives don’t trust their own data, and only 27% of data projects are successful. Those are dismal numbers in what has been called the 'golden age of data.'" As data continues to grow in size to less manageable sizes, a solution is needed for data operations. This article covers the rise in popularity of DataOps as well as the four fundamentals behind the movement: 1. Lean, 2. Product Thinking, 3. Agile, and 4.DevOps
Measuring Your Algorithm’s Performance (Harvard Business Review)
With the complexities of algorithms, the easiest way to measure success is through aggregate metrics, but often underlying those aggregate metrics are inefficiencies that could be holding back your profitability. Understanding how to manage algorithmic performance is not like understanding how to run metrics on other projects, and this article outlines six ways to determine what to measure for your algorithms
Featured Articles on Analytics Leadership and Talent
The Universe of “Data Science” Roles (Towards AI)
This article breaks down every role within the data science field from the main roles like Data Analyst and ML Engineer and also includes less-talked-about support roles such as Analytics Translator and Model Validator. In addition to breaking down the roles, the article includes some helpful skill comparison charts and what tech stacks are utilized for each role.
Let’s Move Fast And Get Rid Of Data Engineers (Seattle Data Guy)
We all want to move fast and break things, right? Well, some companies have tried doing that with their data teams by removing data engineering from the equation entirely. The companies saw data engineering as an unnecessary bottleneck. This article covers what happened to those companies and an in-depth analysis of the effects of data engineering.
3 Reasons Why Teamwork is an Essential Skill in Data Science (KDnuggets)
“Without teamwork, real-world data science problems would be impossible to solve.” This brief article covers three reasons why teamwork is needed specifically in data science teams: 1. Lack of Domain Knowledge, 2. Scope of the Project, and 3. Business Implication of the Model. The article also makes the argument for why business education needs to incorporate more real-world teamwork exercises and what teamwork best practices you should adopt.
Featured Articles on Analytics Uses and Case Studies
Unlocking the Potential of Digital Twins in Supply Chains (Sloan MIT Review)
"Since the early 2000s, digital twin technology has been adopted across many industries and become more accessible and affordable, yet it remains underutilized in supply chain management. This is partly explained by the complex nature of supply chains themselves and partly due to misunderstandings about the technology’s applications, capabilities, and potential value. However, with a proper adoption and implementation strategy, digital twins can deliver immense benefits across a wide range of supply chains." This article delves into the various potential, and underutilized, applications of digital twins for supply chains
Featured Articles on AI
When — and Why — You Should Explain How Your AI Works (Harvard Business Review)
AI adds value by identifying patterns so complex that they can defy human understanding. That can create a problem: AI can be a black box, which often renders us unable to answer crucial questions about its operations. That matters more in some cases than others. Companies need to understand what it means for AI to be “explainable” and when it’s important to be able to explain how an AI produced its outputs. In general, companies need explainability in AI when: 1) regulation requires it, 2) it’s important for understanding how to use the tool, 3) it could improve the system, and 4) it can help determine fairness.
Achieving Return on AI Projects (Sloan MIT Review)
In the current economy, driving tangible business value is more important for data teams than ever before. This article, co-authored by Tom Davenport, provides six strategies to help you achieve a high ROI on your AI projects.
The Art of The AI KPI (Towards Data Science)
Need help deciding which KPIs to track for your AI projects? Check out this article from author David Sweenor, founder of TinyTechGuides. This all-encompassing piece covers:
- How to think about AI KPIs
- Use cases for AI KPIs
- How to know when you've chosen the right KPIs
Responsible AI is a Top Management Concern, So Why Aren’t Organizations Deploying It? (VentureBeat)
Even though responsible artificial intelligence (AI) is considered a top management concern, a newly released report from Boston Consulting Group and MIT Sloan Management Review finds that only 56% of respondents said that #ResponsibleAI is, in fact, a top priority — with only 25% of those reporting their organizations has a fully mature program in place. This article covers what’s holding back responsible AI and why it needs to change.
Artificial Intelligence Needs Both Pragmatists and Blue-Sky Visionaries (Scientific American)
This article, written by notable Computer Science professor Ben Shneiderman, dives into the history of AI futurists and pragmatists and explains with real-life examples why we need both of them to have a healthy tech ecosystem.
Entertaining Analytics Articles
2022 NFL Predictions (FiveThirtyEight)With the NFL season in full swing, FiveThirtyEight created a league-wide projection system. The predictions are based on 50,000 different simulations of the season and will be updated after every game.