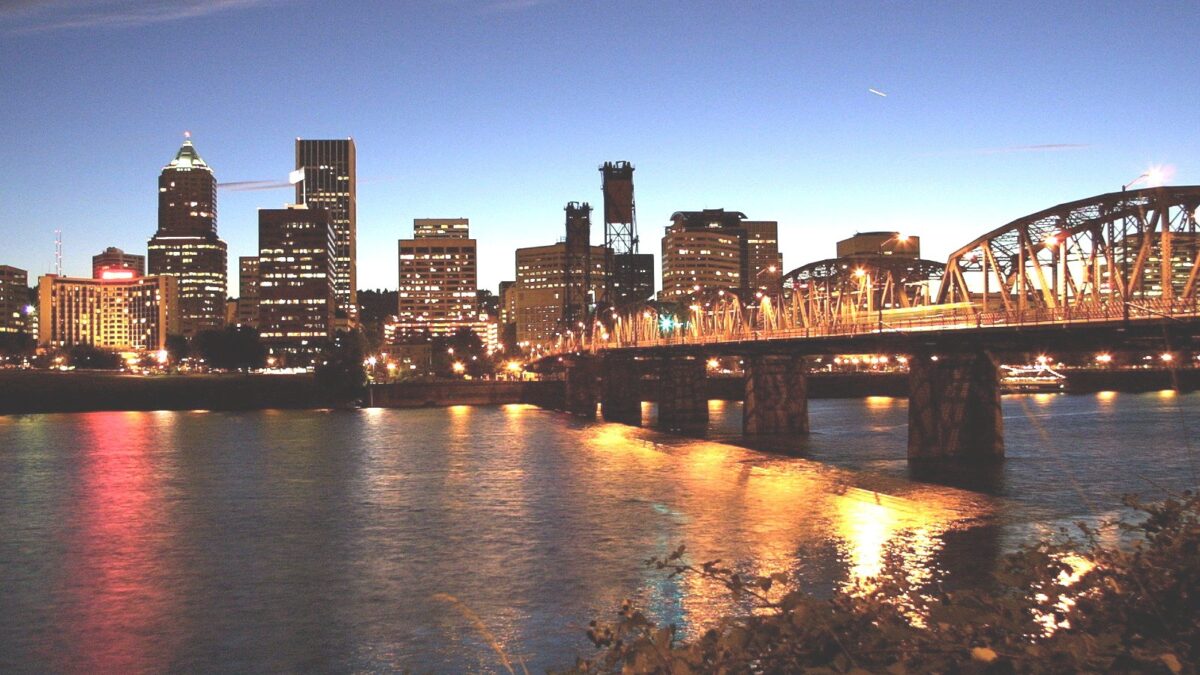
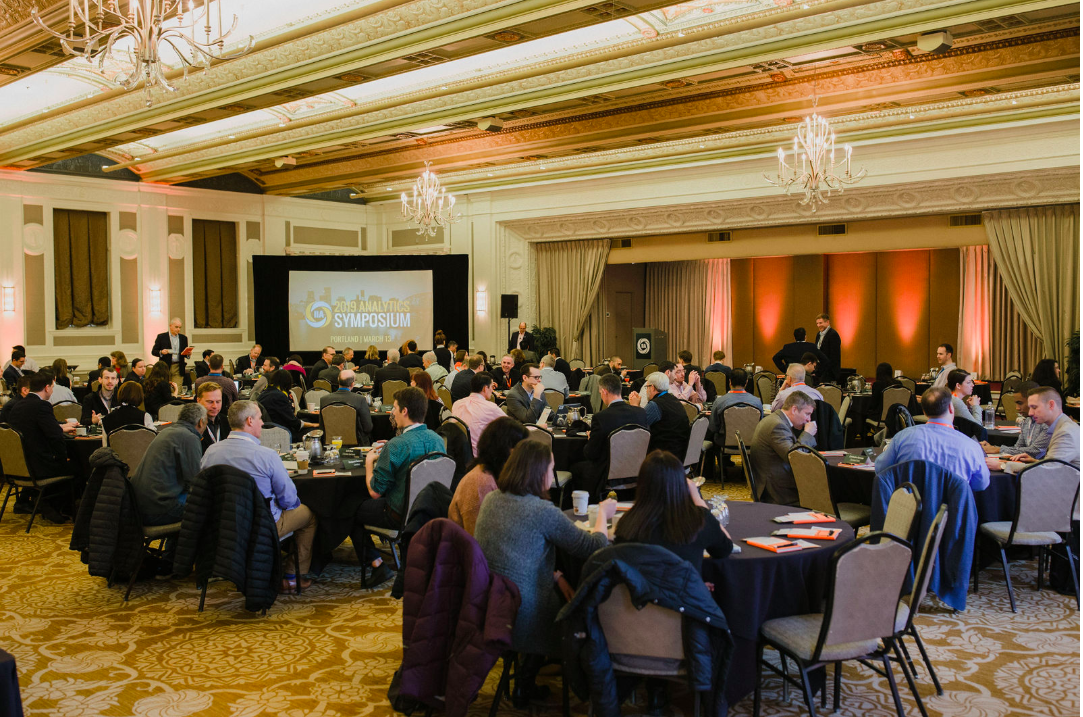
Nearly 200 of International Institute for Analytics’ clients, analytics experts, and members of the analytics community gathered in Portland, Oregon this week for the spring Analytics Symposium. International Institute for Analytics (IIA) also hosted its first Women in Analytics networking event, an interactive Analytics Workshop, and introduced two tracks of sessions to bring the most value to attendees. Below we will review key themes of the conference and highlights from each session.
MARK MADSEN – THE BLACK BOX: INTERPRETABILITY, REPRODUCIBILITY, AND RESPONSIBILITY
Mark Madsen, a member of IIA’s Analytics Expert Network, guided attendees over challenges of model deployment and technology implementation. After all, acquiring new tools is easy, but implementing tools is not. Mark recommends planning for technology first before building internal structures. Applying analytics is often not a technical problem; failures occur more frequently in execution by external functions, not internal analytics development. Problem solving requires closing the loop with both humans and processes. In order to figure out what went wrong with a model, the output must be reproduced to track its path.
MARC DEMAREST – MAPPING AN INFORMATION ECONOMY
Marc Demarest discussed a topic that he often speaks to IIA clients about on Inquiry Exchanges: managing the supply and demand of analytics in an organization; supply being the output of the analytics team to meet the demand of business functions.. The dominant model, particularly at legacy companies, has been driven by the supply side. An advanced analytics program requires a low-barrier, demand side-oriented information economy.
Here is Marc’s five-step process for mapping your organization’s information economy:
Characterize demand-side constituencies
Characterize the distribution infrastructure
Identify implicated suppliers and sources of data
Plot the lines of supply, as they exist today
Plot the lines of supply, as the demand side believes they should be
BRIAN T. O’NEILL – YOU BUILT IT, BUT THEY DIDN'T COME: HOW HUMAN-CENTERED DESIGN INCREASES THE VALUE OF DECISION SUPPORT TOOLS
Brian T. O’Neill, founder of Designing for Analytics, shared analytics UX best practices at the pre-Symposium Workshop. Analytics tool design and UX lead to demonstrable business value (on average, $1 invested in UX returns $100 in value), and it’s crucial to make it centered around humans. The first step to designing more engaging analytics is to decide as an organization what a “good” decision support tool is (the process in which it’s built, features, accessibility, etc.). Brian shared four tips and considerations for designing decision support tools with humans in mind:
Design the data to be relatable to the user
Most people want declarative analytics, not exploratory
Addressing problems in design much cheaper than code
Without usability testing, you’re just guessing and increasing risk
After all, decision support starts with people and problems, not data and code.
GENE KIM – WHAT EVERY ANALYTICS AND PRODUCT LEADER NEEDS TO KNOW ABOUT DEVOPS
DataOps enables dynamic learning organizations and is a key strategy to advance analytics across the enterprise. “DevOps isn’t just for unicorns – it’s for horses, too.” Gene Kim of IT Revolution defined DevOps as “better, faster, safer, happier” than traditional software development and infrastructure processes. He used DevOps case studies from major companies including Capital One, Etsy, and Toyota to demonstrate what high engineering performance looks like. Key indicators of elite performers compared to low performers are: deployment frequency, deployment lead time, deployment success rate, and time to restore. To solve deployment problems, it’s important to focus on the people first; the organization and architecture must be congruent. Gene went over Dr. Steven Spear’s four capabilities and their connection to DevOps:
See problems as they occur
Swarm and solve problems to create new knowledge
Spread new knowledge throughout the organization
Leaders create new leaders
ZACK ANDERSON – IT'S NO GAME TO FIND AND KEEP YOUR DATA SCIENTISTS – EA BATTLES THE MARKET FORCES FOR TALENT
Zack Anderson is the Chief Analytics Officer of Electronic Art (EA), a company that used to have a turnover of data science talent higher than 20%. EA significantly reduced its data science turnover with three successful strategies: great people practices, fostering an analytics community, and life-long learning. EA cultivated an analytics community with an annual internal conference, inspired by Disney’s annual DDAC conference for the company’s internal analytics teams. In addition, EA now has periodic town hall sessions and Slack communication channels for analytics teams. The idea was to connect members of analytics teams across functions and silos to promote knowledge sharing and a thriving community. Zack and his team also created an innovation competition to engage the company’s data scientists.
JANA EGGERS – LESSONS FROM THE UNDERBELLY OF AI
What comes first – the chicken or the egg? According to Nara Logics CEO Jana Eggers and her AI trinity, the chicken is the algorithm, the egg is data, and the bacon is results. Jana believes the egg comes first; train data to learn and grow rather than shut it down. AI reflects the biases and values of its creators. While it is good at perception and categorization, AI is still lacking in contextualization, prediction, and decision-making. Like Jana said, “AI is like artificial light. Artificial light did not replace the sun, it gave us new opportunities. And it still has problems.”
LIZ CONZO & KIRA BARCLAY – BUILDING THE ANALYTICS FACTORY AT DEERE
John Deere has been around for 180 years, but that hasn’t stopped the company from advancing its analytics programs – particularly under the leadership of Kira Barclay and Liz Conzo. In this engaging session, Liz and Kira explored the differences and similarities between teams on the IT side and teams on the innovation side, and how they work together and learn from each other. Established processes are often viewed as a hindrance, but can give teams and enterprises an edge when used leveraged correctly. Kira and Liz work together to innovate and advance the company without losing sight of its core values.
MICHAEL LI – EMPLOYMENT AND TRAINING IN THE ERA OF AI
AI may be replacing jobs but it still needs human supervision, and that is creating new positions and opportunities for those willing to learn. The increasing demand for both data scientists and data-savvy leaders is leading organizations towards different specialized skills training, specifically four skill sets to answer the following questions:
What are bid data, machine learning, and artificial intelligence and how are they affecting my business?
How do we analyze and select winning data science projects?
How do we manage successful data projects from conceptualization to completion?
How do we adopt a data-driven mindset within our group and the broader organization?
MATT LEVINSON – NIKE GETS UP AND RUNNING WITH MACHINE LEARNING AND AI
Nike faces an issue similar to many of IIA’s clients: how can an established, non-digital native company progress towards advanced analytics maturity? Matt Levinson, Director of Personalization Data Science, shared how Nike’s challenge is not its lack of capabilities, but rather its culture – a common theme among non-digital natives. The pre-requisite to overcoming this issue is a strategic vision to create a data-driven culture, and this change starts from the top executive level down to each business function.
MELANIE MITCHELL – AI HITS THE BARRIER OF MEANING
What is required for “human level” AI, or AI that is at least reliable and trustworthy in specific business situations? What do AI systems actually need to “understand”? The deep learning revolution has five problems:
Unreliability in translation, automated image captioning, and (in edge cases) autonomous cars
Subtle (and not-so-subtle) bias in translation, facial detection and recognition, pedestrian recognition, and speech recognition
Problems with generalization, abstraction, “transfer learning”
Lack of “common sense”, background knowledge
Vulnerability to adversarial attacks
BRENT DYKES – MASTERING THE ART AND SCIENCE OF STORYTELLING
If your data doesn’t tell a story, it won’t have the desired impact. Stories beat statistics; they are more memorable and even trigger responses in more parts of the human brain than statistics do. Brent Dykes, Director of Data Strategy at Domo, shared specific tactics to tell more engaging stories with your data:
Tailor your message to your audience
Align data to that message
Don’t overwhelm your audience with too much content – only tell and show what they need to know
Highlight what matters by using color strategically
Stage content to reveal insights
Utilize the data storytelling arc: set up, hook, rising insights, “aha!” moment, and finally the solution and next steps
JESSE ANDERSON – CREATING A DATA ENGINEERING CULTURE
85% of big data projects fail to get into production, and it’s not because of technology – it’s a management problem. The manifestation of a data engineering culture is a correct ratio of data engineers to data scientists. Jesse Anderson of Big Data Institute defines a data engineer as someone who has specialized their skills in creating software solutions around big data, a data scientist as someone who has augmented their math and statistics background with programming to analytics data and create applied mathematical models, and a database analyst as an SQL-focused person. An effective data engineering team should be multidisciplinary and needs experience in distributed systems, programming, analysis, visual communication, verbal communication, project veteran, schema, and knowledge.
JENNIFER PRENDKI – AGILE FOR THE DATA SCIENCE TEAM
Dr. Jennifer Prendki, a member of IIA’s Analytics Expert Network, made the point that machine learning and data science functions are “notorious” for poor ROI. A solution? Implementing Agile to utilize skills and resources more efficiently. The Kanban methodology is suited for small teams, while lean and minimum viable products (MVP) works well for larger organizations. It makes more sense to build just enough functionality to get feedback from users.
MICHAEL HOFFMAN – MIXED REALITY AND ANALYTICS
Mixed reality has enabled and enhanced analytics efforts, but it still has limitations. Michael Hoffman, Founding Partner of Object Theory, shared use cases of various mixed reality technologies. Mixed reality helps with:
Effective collaboration across dispersed teams while interacting with a wide variety of geospatially accurate 3D information and supplementary 2D information
With ML/AI + MR, the collected data can be analyzed for patterns and can be presented geospatially right where an issues need addressing
Simulations and visualizations of collected sensor data can be played and animated geospatially and can be aligned with physical objects related to the data
Augmenting existing screen real estate with a nearly infinite amount of additional 2D panels as well as 3D more available information for analysis
The combination of AI+MR reduces training cost by making it possible to recreate realistic interactive soft skill scenarios, automate the AI/ML assessment of performance and generation of precise feedback
Helps design collaborators establish an accurate shared context of a product under design for efficient, accurate decision making and faster iteration cycle times
Collaborators feel a sense of presence, plus virtual geospatial annotations and markers can accurately convey intent to establish a more accurate shared context resulting in more informed decisions and fewer mistakes due to miscommunication
CATHY HUYGHE – UNCORKING ANALYTICS: MOVING THE WINE INDUSTRY TOWARDS DATA-DRIVEN DECISIONS
The wine industry is more than 6,000 years old, which is significantly more than most industries can say. Cathy Huyghe, Co-Founder of Enolytics, is creating a revolution in this ancient industry by tapping the vast potential of winery data and consumer data. She described the challenge in communicating the business case for data while maintaining the romantic culture of wine. Attendees got a taste of a new wine analytics tool called Quini as well as a taste of three local Oregon wines – just for the sake of collecting data, of course!
With 16 speakers sharing varying perspectives of the analytics journey and future, one thing was consistent: the story you tell with analytics is just as important as the way you derived the results. This ever-growing and evolving industry is making huge impacts on business. We at International Institute for Analytics want to give a huge thanks to all of the speakers and attendees for making this Analytics Symposium the most well-attended and engaged one yet! Keep an eye out for more detailed write-ups on the sessions over the coming weeks. We hope to see you at IIA’s next Analytics Symposium in Detroit on October 8-9, 2019.