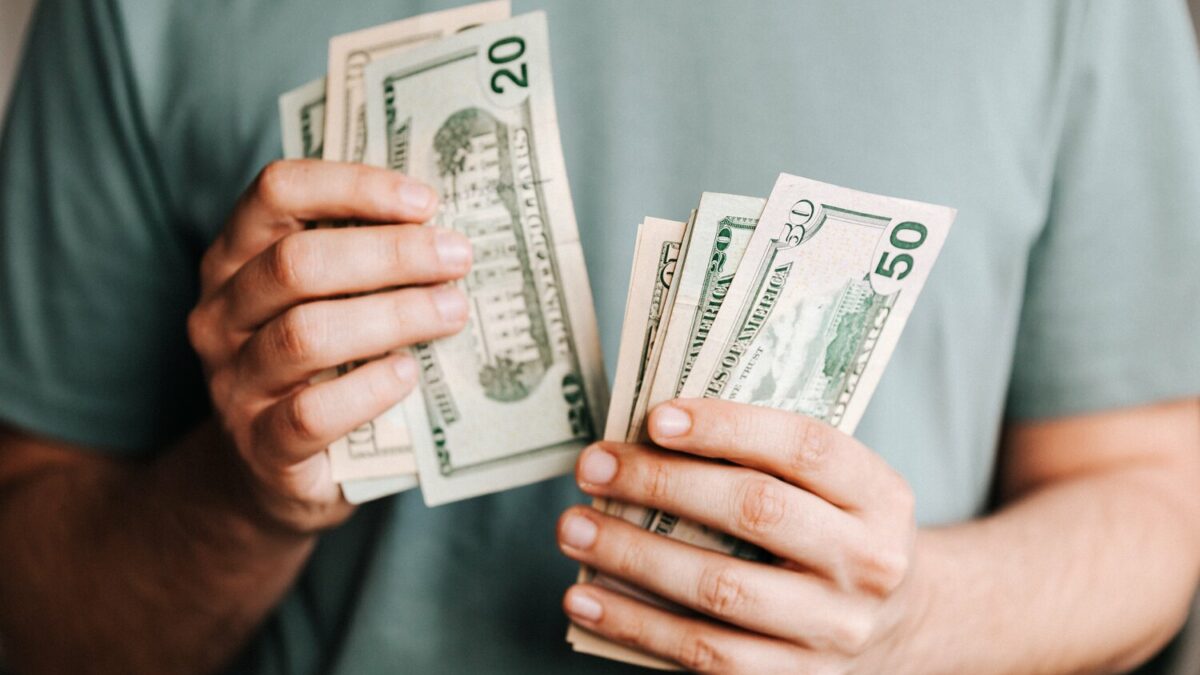
It is natural to get excited about the prospect of building and deploying an interesting and high impact new data science process. Unfortunately, you have to also put effort into some less exciting aspects of such an endeavor. One item that is often underestimated and neglected, if not omitted, is ongoing maintenance costs for a new process. This blog discusses why we got away with ignoring process maintenance in the past but also why we can’t get away with it any longer.
Homes And Cars
One of the most expensive and exciting things that some people do is to build a new home. Even a new home will need maintenance, however. One thing that often catches first time owners of any home by surprise, whether it be a new home or not, is how much maintenance is required. If a buyer is barely able to make the mortgage payments, they’ll be in trouble when the roof leaks or the deck needs stained. The buyer’s budget must account for ongoing maintenance.
A new car is another major purchase. New cars today come with maintenance schedules that lay out exactly what needs done and when. This lets you project accurately what costs you will incur over time and plan for them. A car won’t last without periodically getting new tires and oil filters.
Anyone who owns a home in a pool and tennis community or who lives in a condominium is familiar with having to pay annual or monthly fees that go toward upkeep of the common property. These fees and related costs are again tied to the basic, predictable maintenance that is a fact of life. Some states legally require homeowners associations to create and follow a plan for maintenance to avoid a glut of properties falling into disrepair.
Very few readers will find anything I just wrote the least bit surprising, unexpected, or unreasonable. I’ll bet, however, that many readers will be unable to say with confidence that they and their organizations take the ongoing maintenance required for data science processes just as seriously.
Data Science Processes Of The Past
In the old days where models were often used once or twice and then either discarded or explicitly updated as part of a new project, we didn’t have to think about maintenance very much. Models used once or twice were like disposable product that didn’t come with long term worries. Traditional models run at set time points in a batch mode typically had a lot of manual intervention.
I recall updating response models for each campaign. While there was a general template and approach, we still had to customize the process for each campaign. There was a budget for this process of “building a new model” even though we were really just tweaking the past model. Effectively we were doing maintenance on the process while not thinking of it that way. The template and approach evolved over time as data changed, business strategies changed, or our methodologies improved. Each model had the latest and greatest capabilities through the indirect maintenance gained through the ongoing usage occasion by usage occasion updates.
Data Science Processes Today
The approaches in the prior section often do not apply in today’s environment. Often, a data science process is built and deployed in an automated and operational setting as I discussed at length in my book The Analytics Revolution. This means that there are not the organically occurring ongoing opportunities of the past to revisit the process. As a result, there must be explicit planning and budgeting to revisit processes on a regular basis.
Aspects of a data science process that might need attention include, among others:
- Adjusting data ingest and preparation logic to account for new or changed data sources
- Updating modeling methodologies to make use of the latest techniques available
- Upgrading APIs or other interfaces with outside systems and processes as protocols change
- Diagnosing causes of model effectiveness degradation and taking mitigation action
- Developing new reports from the processes’ output so it can be used in new contexts
None of those items are free from time and cost investments. However, unless planning is done up front, the activities are often overlooked and not proactively budgeted. Then, when maintenance is needed, nobody has the time or budget and people end up having to do the work on top of their regular workload. This makes employees unhappy and also can lead to burnout.
Lay Out A Maintenance Plan From The Start
Approach a new data science process like it is a new car. Think through what attention the process is likely to require over time, at what intervals, and what the associated effort is. Use this information in two ways. First, when proposing the work be sure to call out the ongoing maintenance requirements so that stakeholders know what they are really committing to. Second, truly budget both the time and money required to do the maintenance so that you don’t end up with broken down and outdated processes that are not able to meet the needs of the organization.
We all know what it is like to visit a poorly kept house or to pass a beat up car on the road. Don’t be the data science equivalent of those run-down assets. Instead, strive to be like the well-kept house or car that you can tell the owners are proud of. I can assure you that those owners take maintenance seriously enough to both budget for it and get it done. Otherwise, their property wouldn’t be in the fine condition that it is in.
Originally published by the International Institute for Analytics
Bill Franks, Chief Analytics Officer, helps drive IIA's strategy and thought leadership, as well as heading up IIA's advisory services. IIA's advisory services help clients navigate common challenges that analytics organizations face throughout each annual cycle. Bill is also the author of Taming The Big Data Tidal Wave and The Analytics Revolution. His work has spanned clients in a variety of industries for companies ranging in size from Fortune 100 companies to small non-profit organizations. You can learn more at http://www.bill-franks.com.