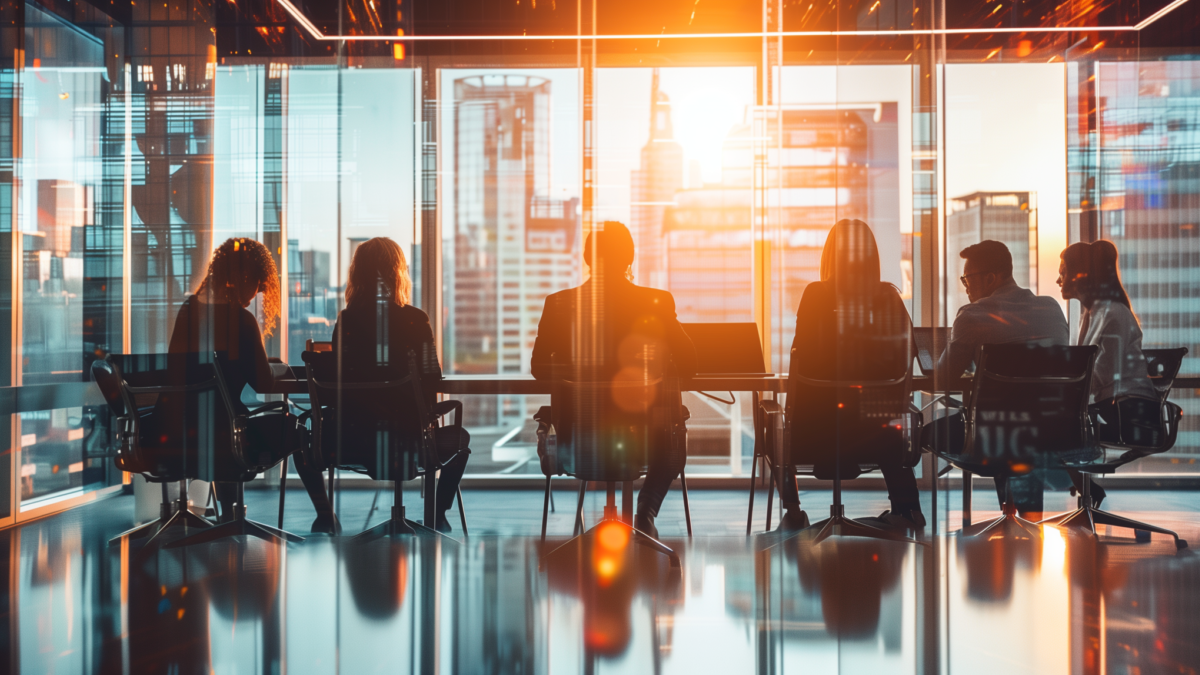
IIA’s Research and Advisory Network (RAN) clients leverage battle-tested frameworks and an exclusive network of over 150 active practitioners and unbiased experts to plan, prioritize, and execute strategic enterprise data and analytics initiatives. We regularly check the pulse of trending topics for the RAN community and facilitate critical conversations in virtual roundtable format for peer-to-peer exchange.
In a recent roundtable discussion with IIA RAN clients, data and analytics leaders from diverse industries gathered to share their approach to building and leading AI teams. The discussion covered a wide range of topics, from talent acquisition and retention to team structures and roles. Here are the key takeaways:
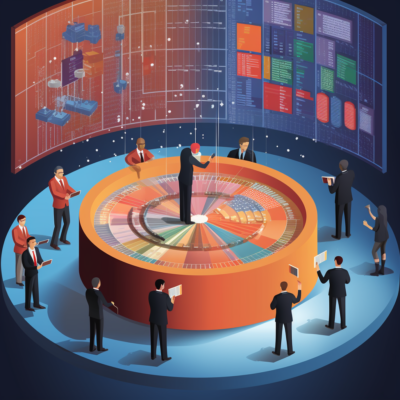
Research and Advisory Network (RAN)
How could you benefit from hundreds of C-suite level D&A executives at your fingertips? Get access to the leading network of independent analytics expertise, allowing you to apply real practitioner insights against initiatives, projects, and problems through RAN today.
1. Talent Acquisition and Retention
Data and analytics leaders are rethinking traditional talent acquisition strategies that heavily focus on technical skills. Instead, there is a growing emphasis on sourcing candidates from diverse academic backgrounds like English or Philosophy, valuing their critical thinking and effective communication abilities. This shift recognizes the crucial need for team members who can not only manage complex data but also articulate actionable insights that resonate with business leaders. The need for this balance became evident as organizations faced challenges in demonstrating the value of analytics work to business stakeholders, often finding that without the ability to effectively communicate, even technically proficient work went unrecognized and underutilized.
Talent retention poses its own set of challenges, particularly in retaining top talent with expertise in artificial intelligence. Many leaders reported difficulties in filling senior positions with most applicants being at the junior level and a notable gap in mentoring and development for early-career professionals. To address this, some organizations have successfully hired senior-level talent from international markets, balancing budget constraints with the need for experienced professionals. Moreover, in an era where the tech sector's allure is waning due to its instability and narrow job scope, some companies report low attrition rates by focusing on long-term career growth over immediate financial incentives. One participant drew parallels between career growth and stock market investments, encouraging employees to consider the potential long-term value of their positions.
This broader conversation about talent in data and analytics underscores a pivotal shift: the integration of technical acumen with strong communication and a deep business understanding is becoming the gold standard. As data and analytics roles evolve, the ability to navigate complex technical tasks while influencing and leading across organizational boundaries is increasingly recognized as a marker of truly valuable professionals.
2. AI Training and Upskilling
The discussion on AI training and upskilling within organizations highlights several approaches aimed at enhancing workforce capabilities and adapting to the evolving technology landscape. Key strategies include the implementation of mentorship roles by senior figures guiding diverse teams across departments, crucial for fostering responsible use of AI and data analytics. This mentorship is complemented by the "pod concept" where experienced developers pair with less seasoned ones to facilitate skill transfer and deeper understanding of the data landscape, enhancing the overall talent pool.
Internships and structured upskilling programs are pivotal in cultivating a knowledgeable workforce, with some organizations transitioning analysts to more advanced data science roles to meet the increasing demand for AI expertise. These programs are tailored to various educational levels, from recent bachelor's degree graduates focusing on basic tasks to PhD holders specializing in quantitative analysis. Furthermore, the adoption of machine learning roles, such as ML engineers and MLOps teams, reflects a response to the need for scalable solutions and the integration of advanced AI tools, which simplify processes and potentially blur traditional role distinctions within data teams.
Overall, these approaches not only aim to retain talent by aligning career goals with organizational opportunities but also prepare companies to effectively navigate the shift toward more complex AI-driven environments. This strategic talent management ensures organizations remain competitive by harnessing and developing top-tier professionals from diverse backgrounds and skill sets.
3. The Evolution of Team Structures and Roles
The evolution of team structures and roles in data and analytics reflects a growing complexity and specialization within the field. Organizations have adapted by creating distinct groups within their analytics teams, where one group focuses on interfacing with customers and stakeholders, excelling in communication and understanding business needs without deep technical skills. The other group, highly technical, delves into data science and programming, applying their skills to creatively solve business problems. This dual structure ensures a balance between technical expertise and business acumen, crucial for effective problem-solving and decision-making.
Further refining this model, some organizations have adopted a "pod strategy" as mentioned earlier, integrating various disciplines like product owners, engineering managers, and data scientists into dynamic product delivery teams. These pods operate within a federated model that combines central strategic support with operational autonomy, enhancing specialization and responsiveness. Additionally, roles such as ML engineers and data catalysts emphasize the importance of technical literacy across teams to effectively leverage data insights. The implementation of cross-functional teams, frequent interactions with business stakeholders, and roles specifically designed to bridge business and technical gaps, like the "business activator," are pivotal in aligning projects with business goals and enhancing the practical application of analytics solutions.
This structured approach is complemented by strategic realignments and innovations in team operations, responding to the dynamic needs of the AI and ML landscapes. Organizations are transitioning from human-driven decision supports to automated, data-driven systems, necessitating new strategies and team alignments to handle increased data migration to the cloud and the integration of intelligent automation. These shifts are not just about technical adjustments but also about fostering a culture that supports continuous learning and adaptation to maintain relevance and efficiency in an increasingly data-centric world.
IIA virtual roundtables are exclusive, invite-only discussions designed to promote peer-to-peer exchange on pressing challenges in the data and analytics community. Seats are limited and reserved for C-suite data and analytics leaders or equivalent at mid- to large-sized enterprises. Conversations are geared toward non-digital native companies. If you meet these criteria, contact us for more information.